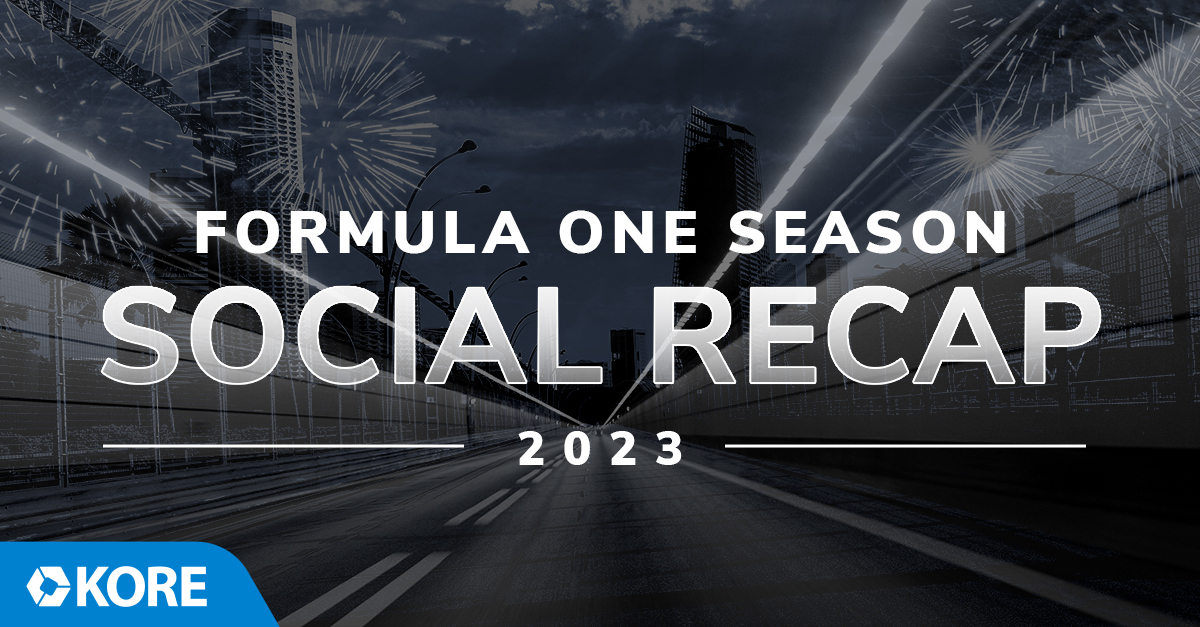
Who Won the Fan Engagement? KORE’s Formula One Season Social Recap
Learn which F1 teams, drivers and sponsors won the hearts of fans online gaining the most visibility, engagement and value from social media.
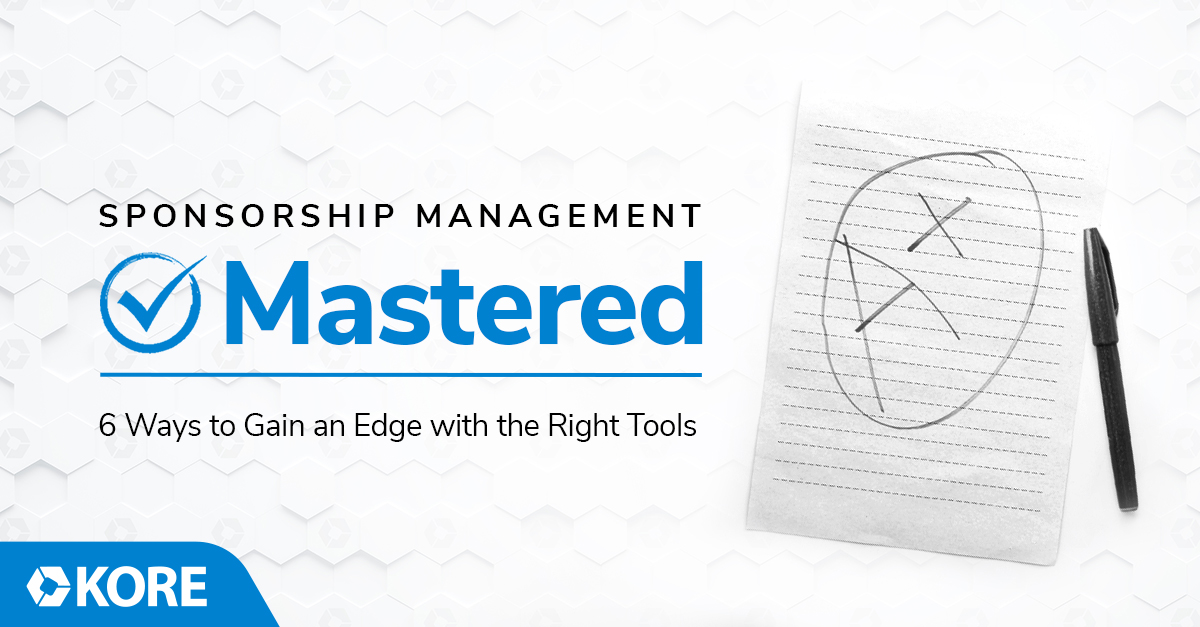
Sponsorship Management Mastered: 6 Ways to Gain an Edge with the Right Tools
Dive deep into success stories from prominent sports teams and events using sponsorship tools that help gain a competitive edge, boost revenue and improve efficiency.
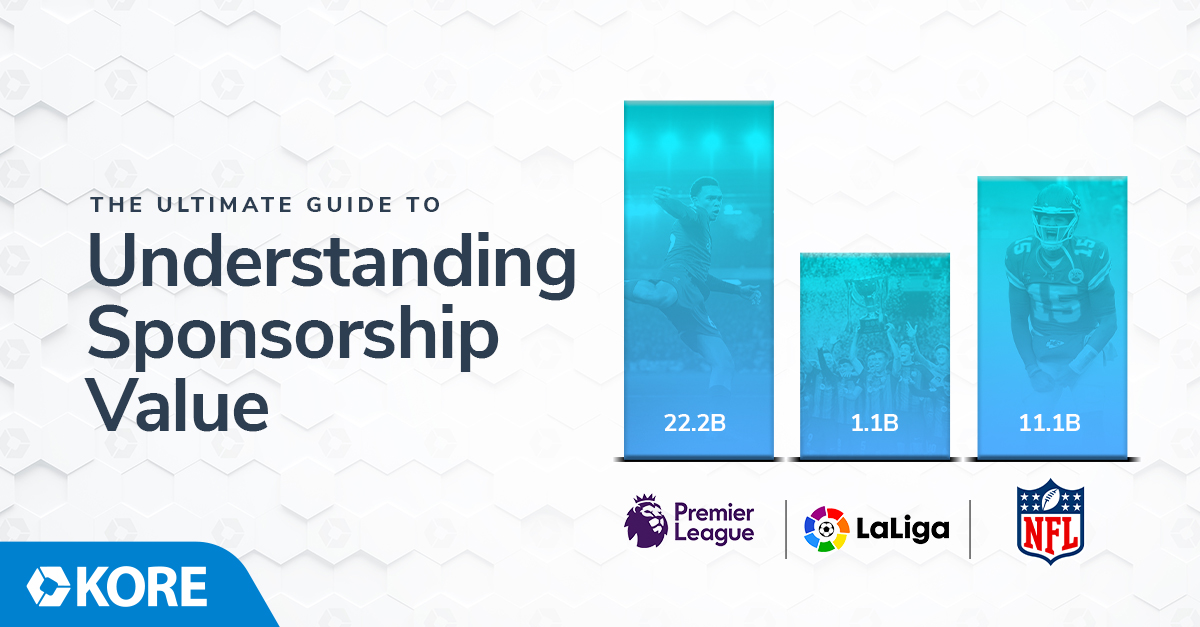
Understanding All Aspects of Sponsorship Value: How to Identify and Quantify Your Investment for Maximum Impact
As the year draws to a close, brands are encouraged to reflect on their sponsorship activities and prepare for a stronger 2024. The article highlights the importance of setting clear objectives, maintaining consistent partner interactions, and using a unified platform for data, insights, and communication.
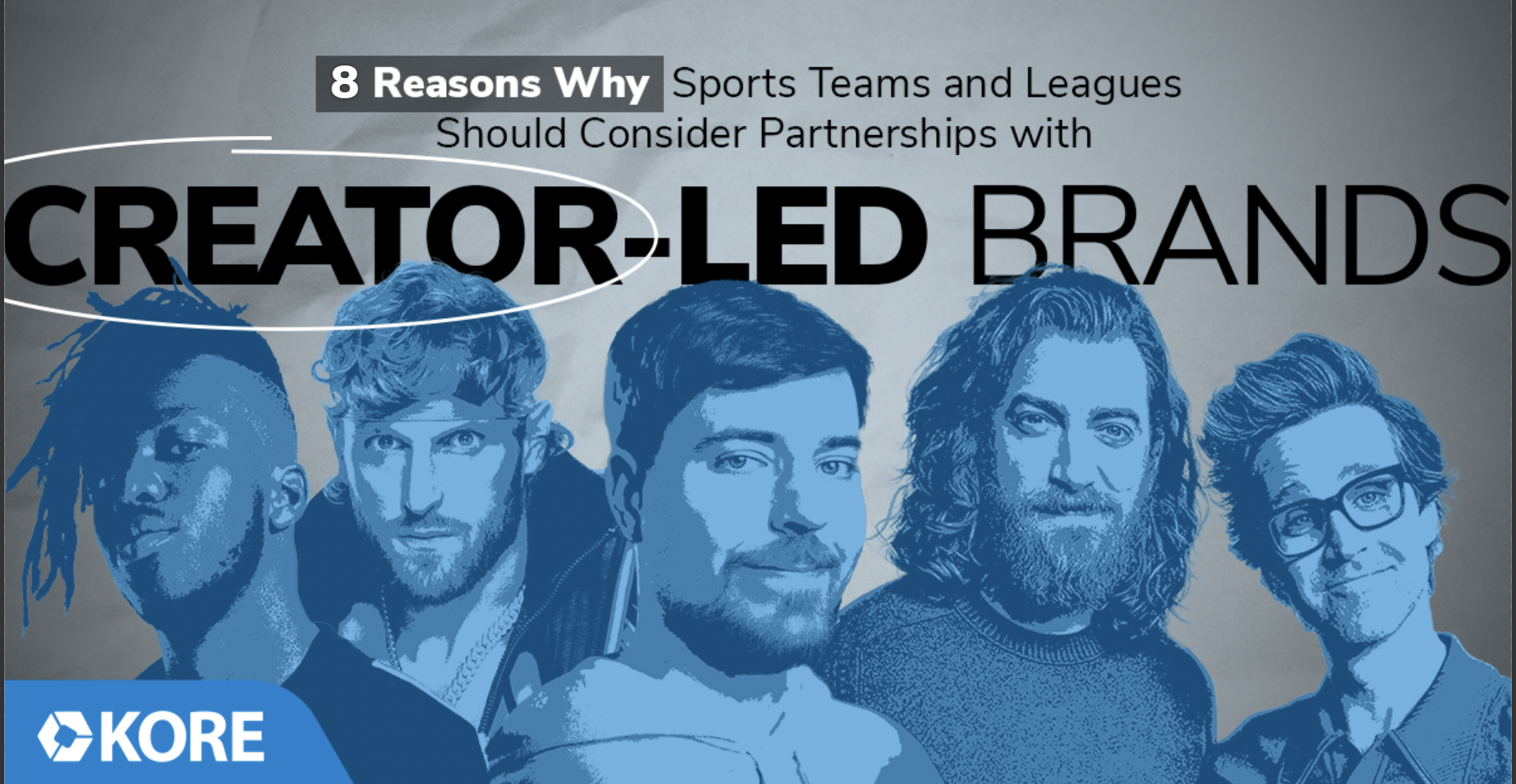
8 Reasons Why Sports Teams and Leagues Should Consider Partnerships with Creator-Led Brands
In the ever-evolving world of sports marketing, teams and leagues are constantly seeking new and innovative ways to connect with fans, enhance their organization’s presence,
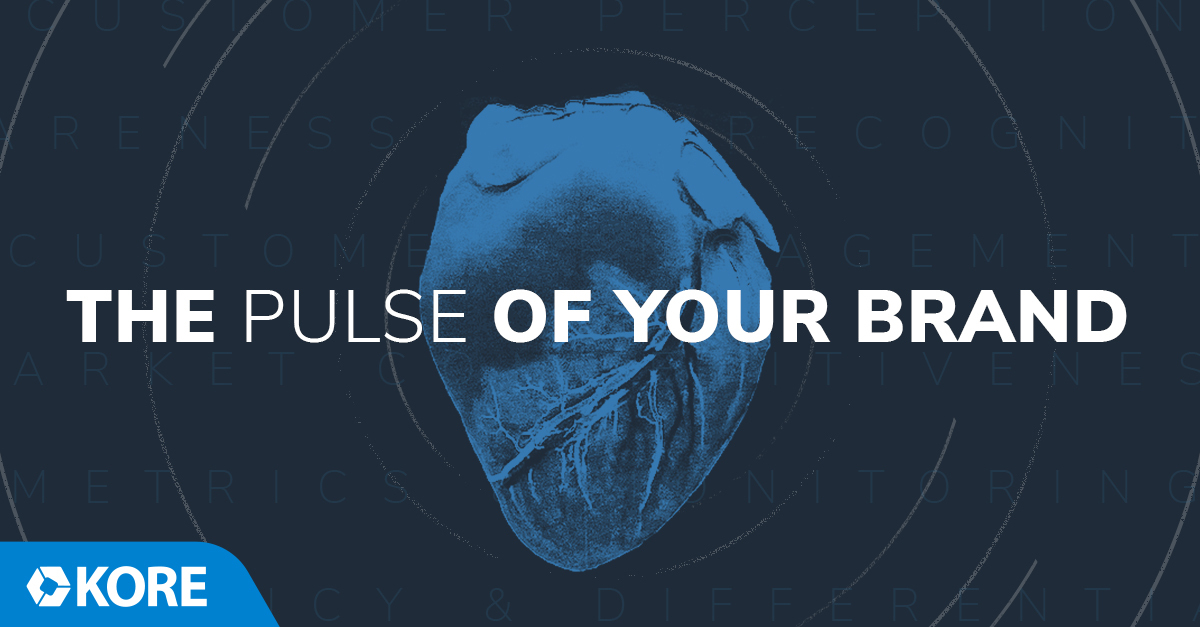
The Pulse of Your Brand: Why Monitoring Sponsorship Performance is Essential for Brand Health
In today’s fast-paced digital era, brand image and reputation can change in a heartbeat. Amidst this ever-changing landscape, sponsorships have emerged as a significant tool
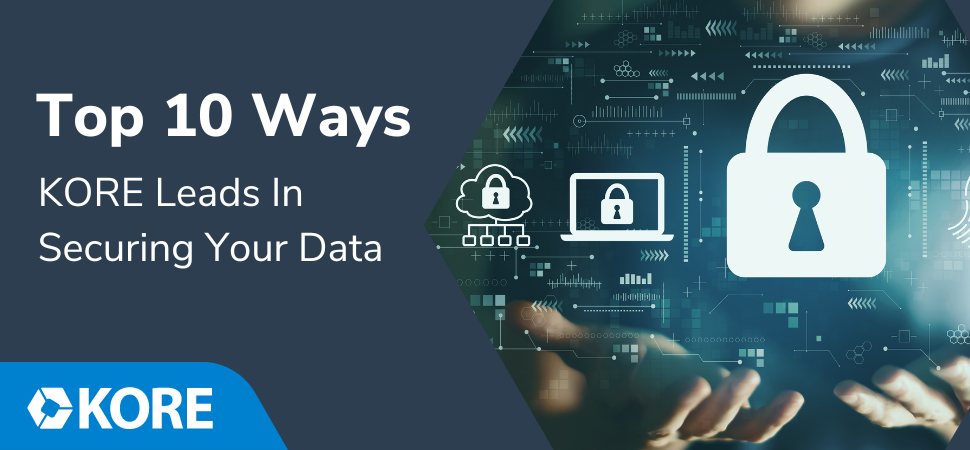
Top 10 Ways KORE Leads In Securing Your Data
How to Keep Your Organization’s Data Safe In today’s digital landscape, safeguarding your data is non-negotiable. Whether you’re a global enterprise or a growing startup,
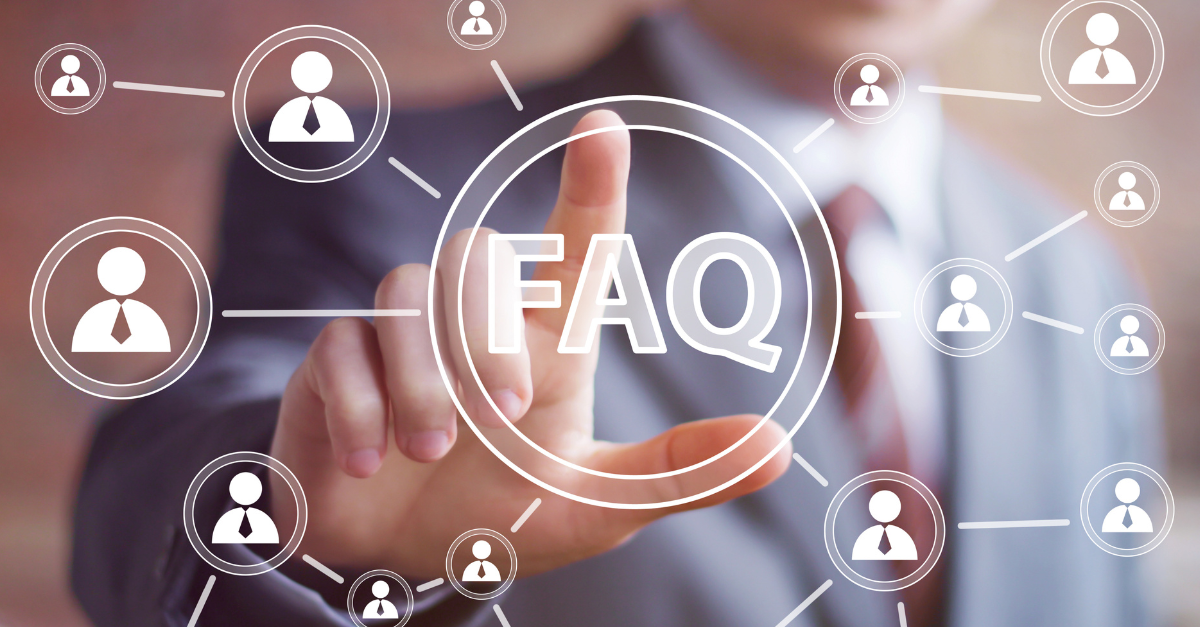
2023 Sponsorship Governance Series: Part 3; FAQs & Key Components
FAQs to Understanding Sponsorship Governance Use these FAQs and key components to gain a clear understanding of the basics of Sponsorship Governance. What is the
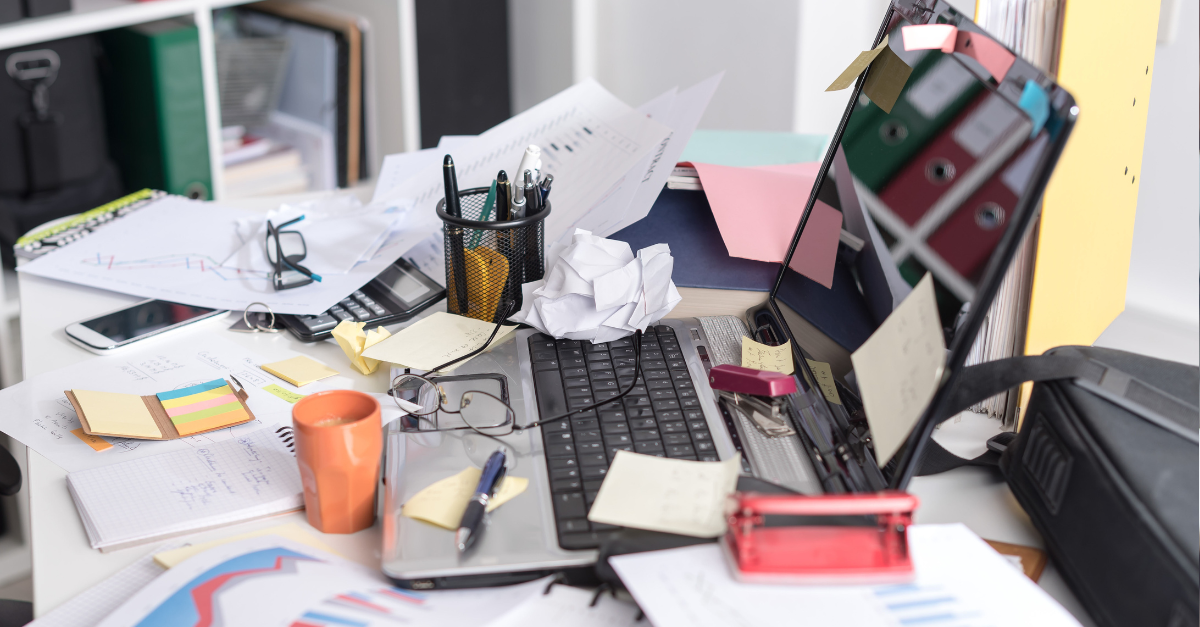
2023 Sponsorship Governance Series: Part 2; How to Steer Clear of Chaos & Maximize Partnership Success
Partnerships never go out of style We can all recall instances where an artist, influencer, or athlete’s personal life turns into a brand partner’s nightmare
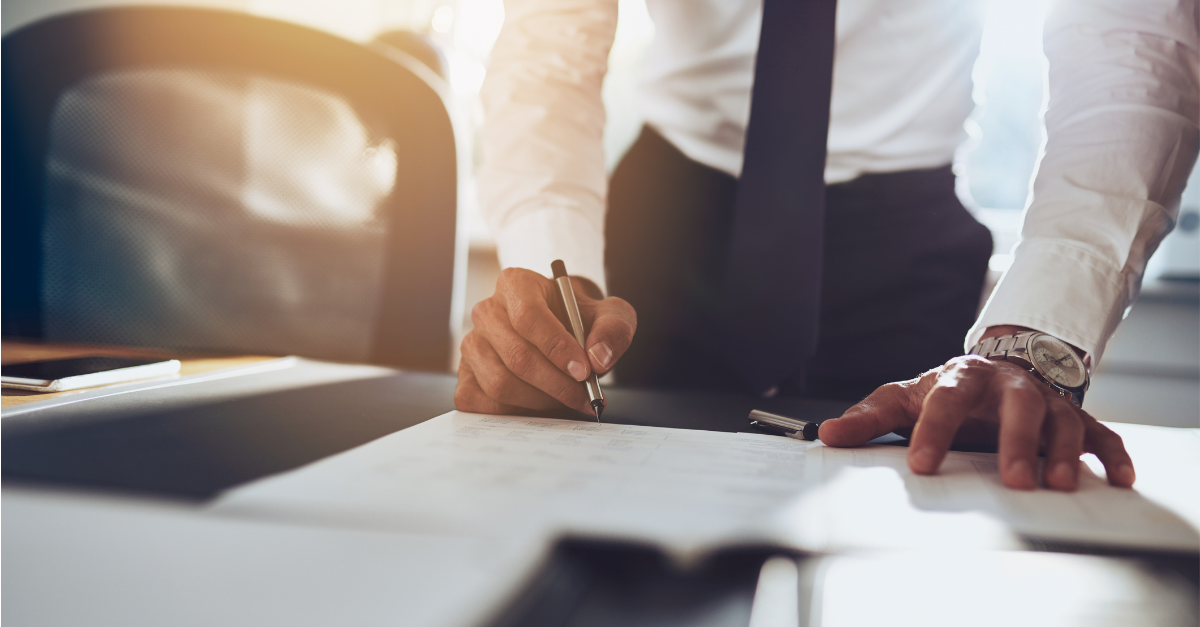
2023 Sponsorship Governance Series: Part 1; Why Governance is Essential for Your Sponsorship Strategy
What You’ll Learn The concept and significance of sponsorship governance for brands How brands can minimize risk, and safeguard their reputation while maximizing sponsorship
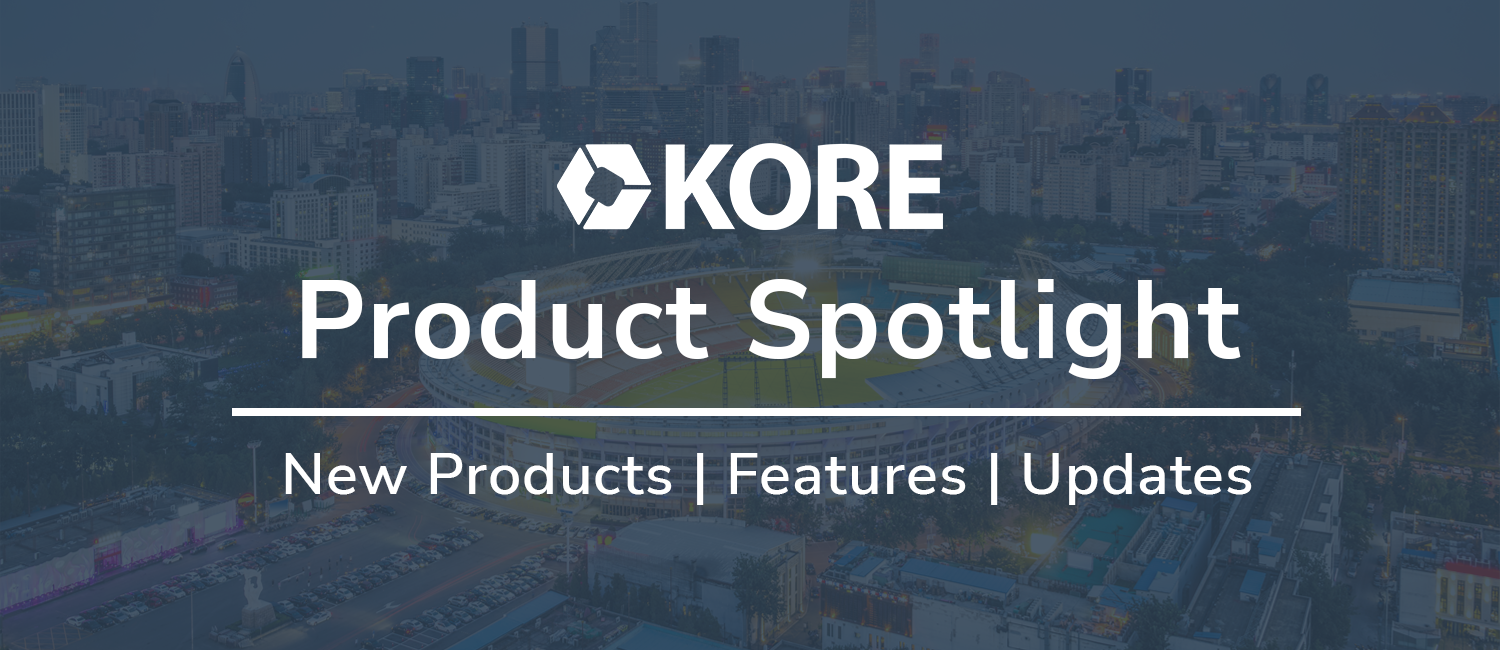
3 Steps to an Airtight Partnership Application Review Process: Gain Transparency & Find the Right Partners
4 Reasons to Automate Your Partnership Application Review Process ASAP Organizations are struggling to keep up with the manual process of reviewing and approving hundreds
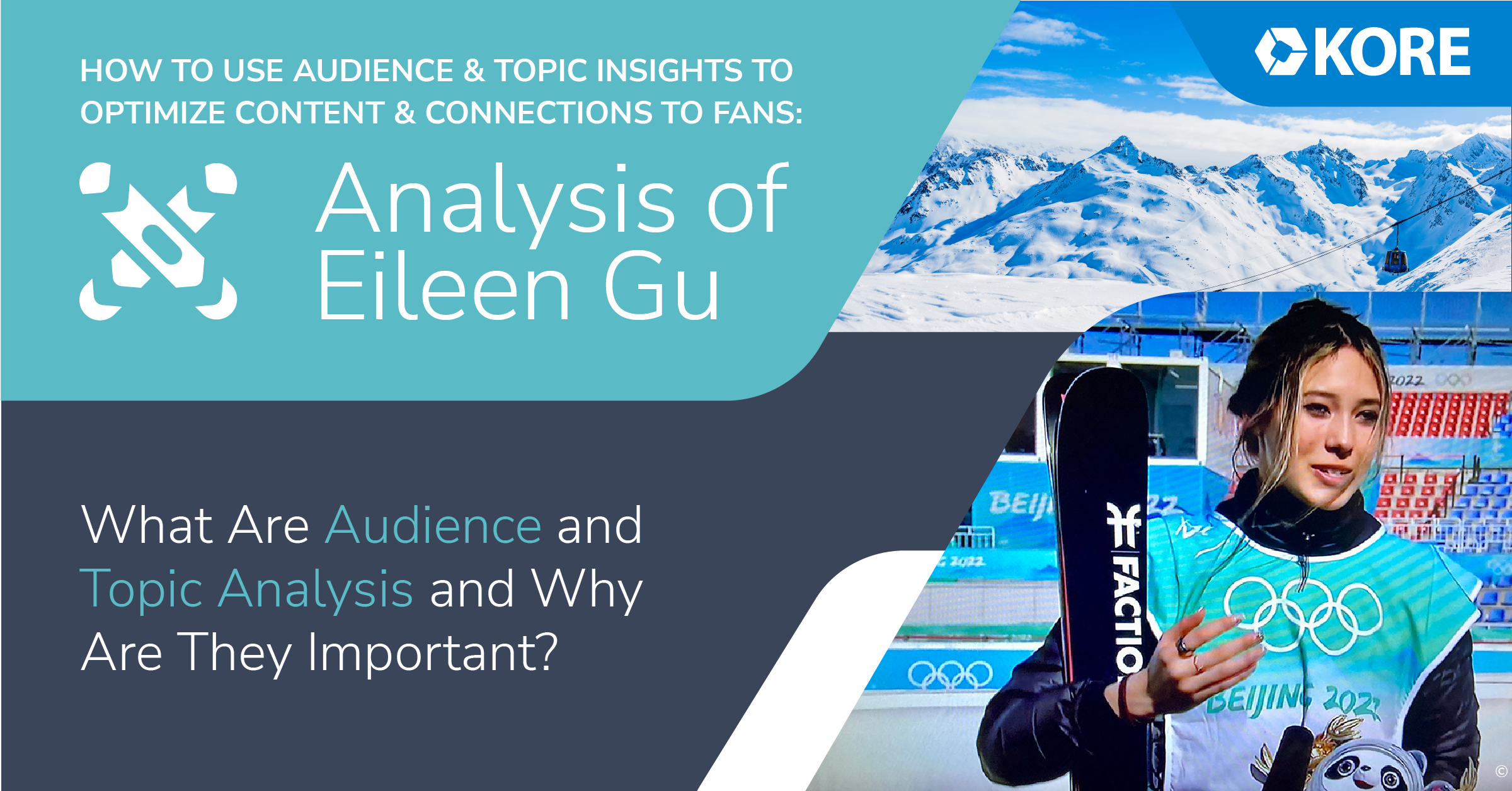
How to Optimize Social Content & Connections with Fans Using Audience & Topic Insights
Audience analysis allows brands to learn about who they’re reaching on social media platforms, like Instagram, TikTok, and Twitter. Topic analysis enables brands to discover
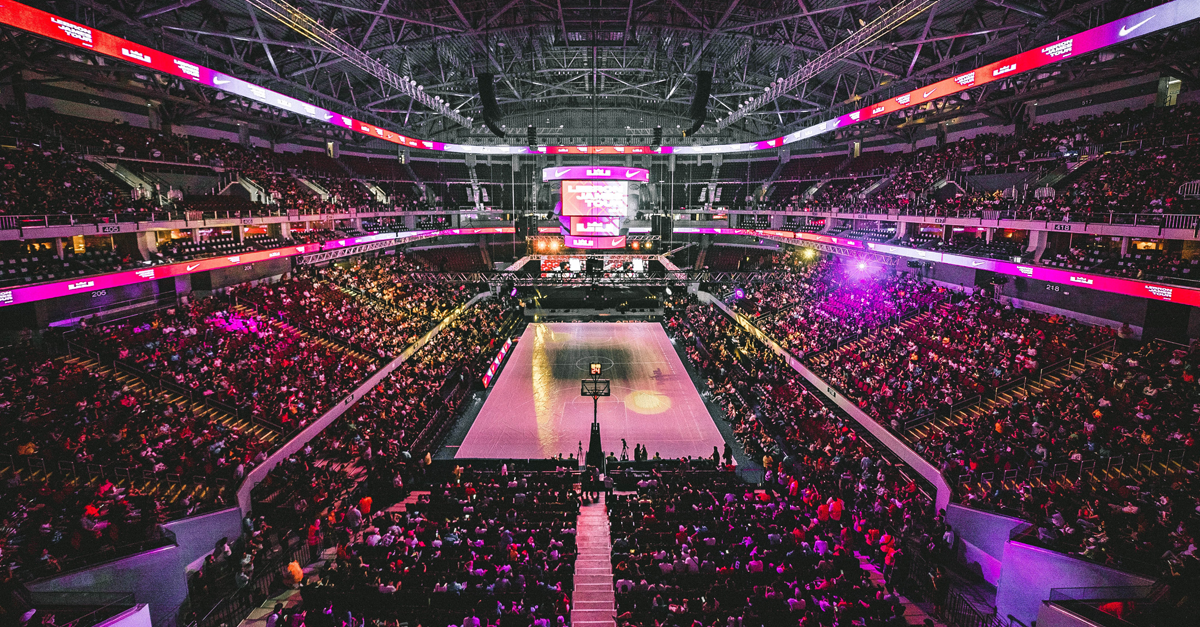
NBA Sponsorship Trends Uncovered: Across Social, NBA Steals the Show in Video Content
While global football is generally in its own universe when it comes to world-wide fandom, the NBA is in a league of its own regarding
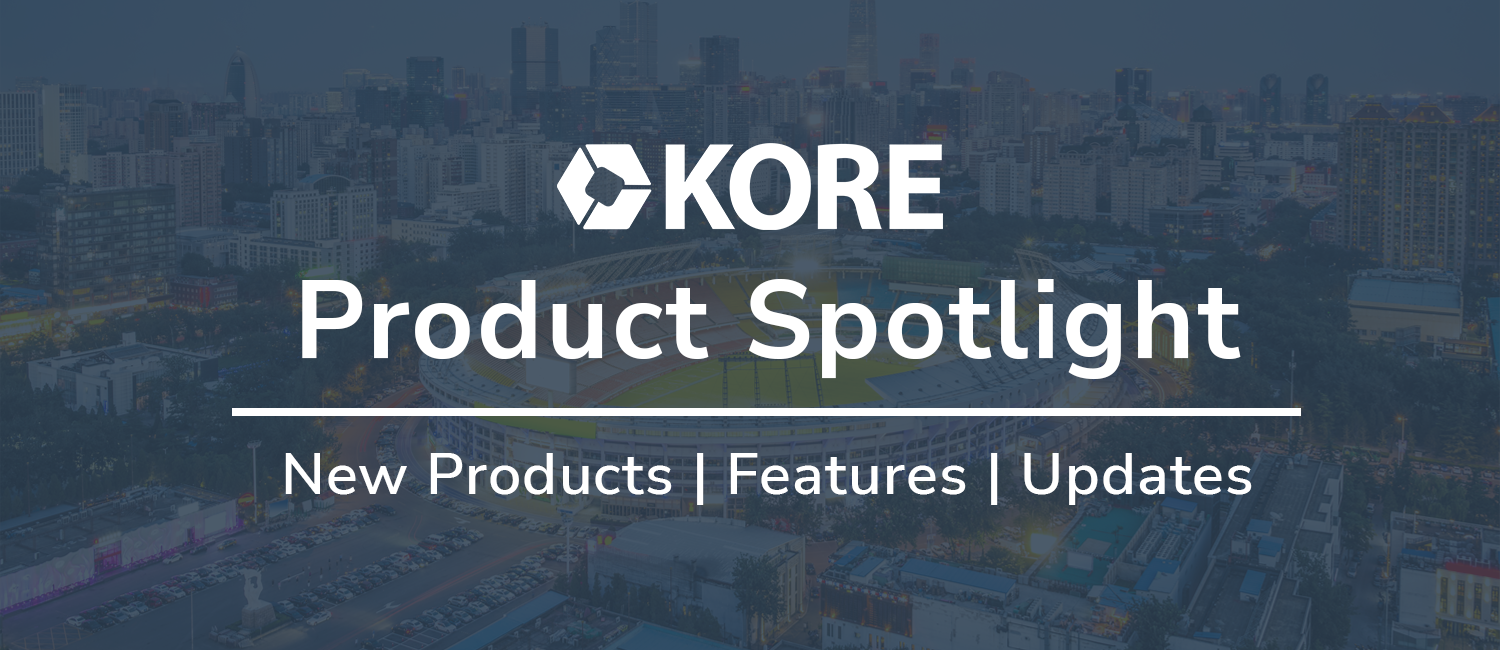
Sponsorship Reporting: How to Track Related Partnership Assets within a Deal for Easy Breezy Reporting
Overview: Agree on an “Activation Budget”, Specify Assets Later From our work with sports teams, we noticed that often in partnerships asset types are not
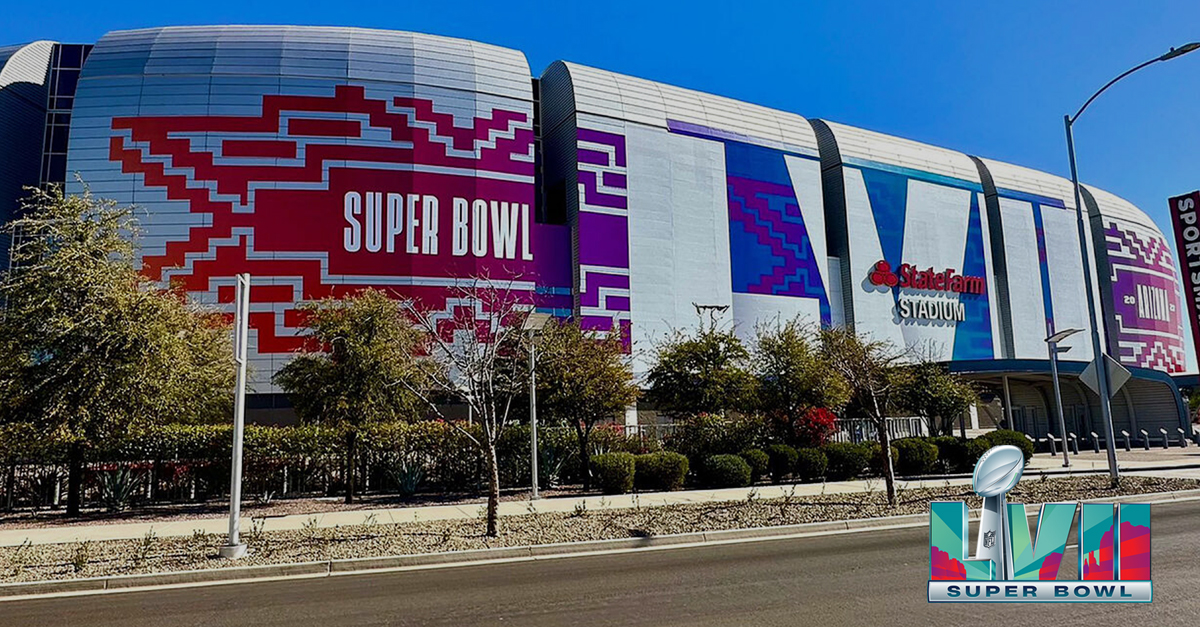
Insider Insights: Sponsorship & Activation Spotlights Surrounding Super Bowl LVII on Social Media
The impact of Pepsi’s Super Bowl ad teaser campaign featuring Steve Martin and Ben Stiller continues to gain traction across the social scene. Lean into
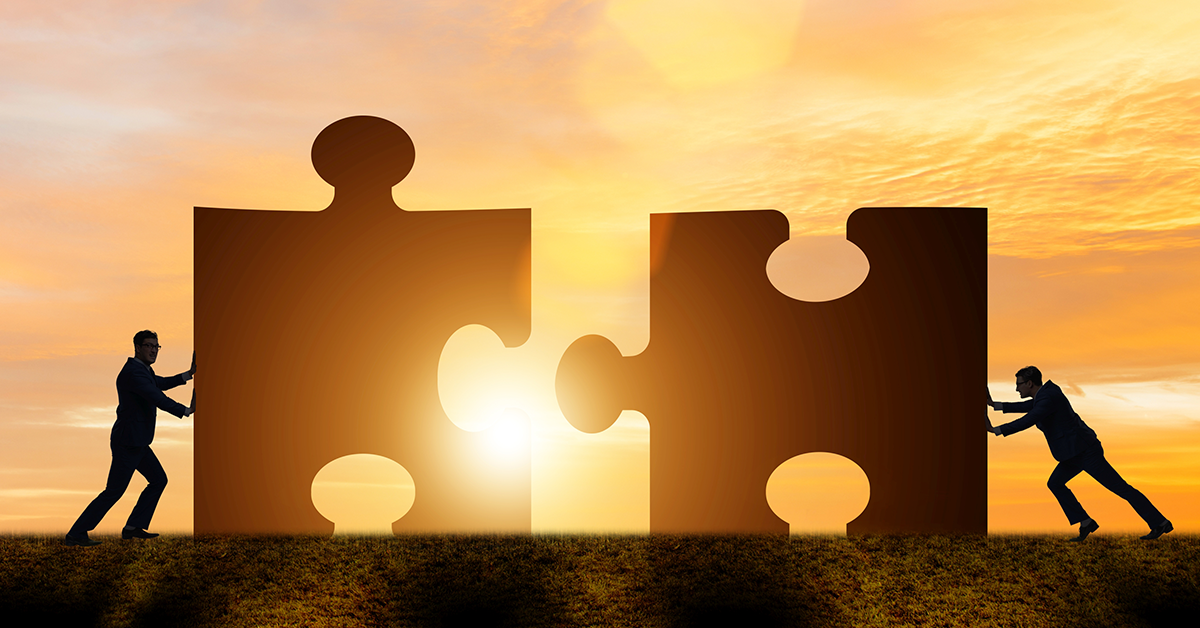
How to Ask Your Sponsorship Partners What Their Objectives Are
One of the most important pieces of the partnership puzzle is figuring out what your partners and sponsors want from their investment. Not only does
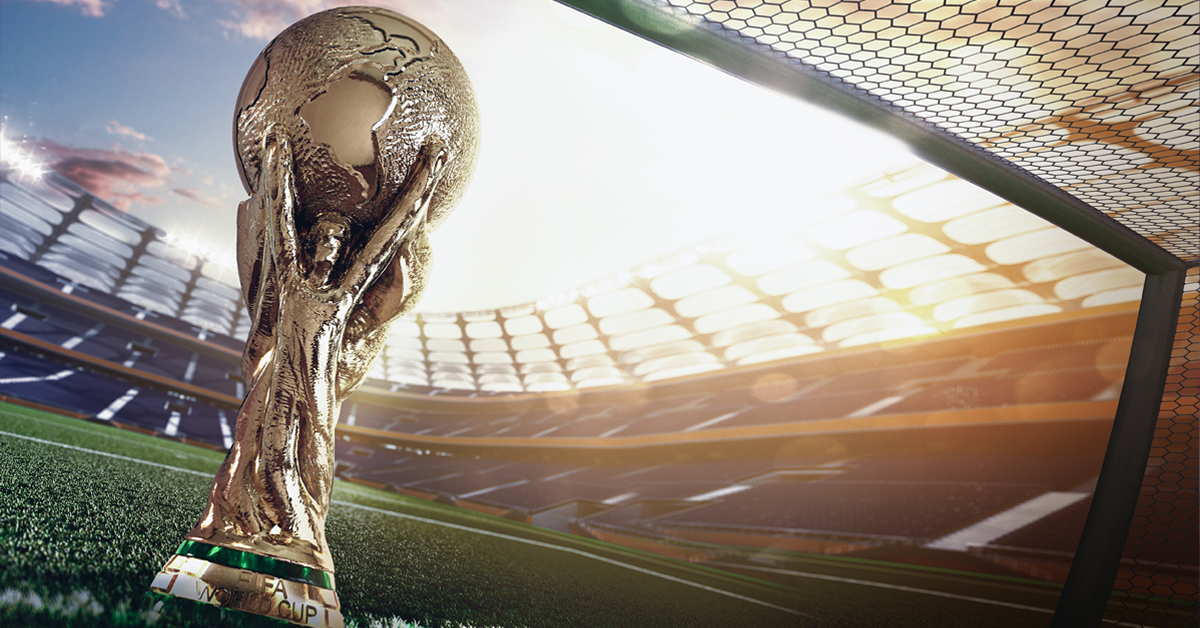
Global Football Market Intel Recap: The sponsorship impact of the most engaged sport across social media
KORE’s market intel explores Leo Messi’s record-breaking year, women’s football, why the swoosh dominates, and how Louis Vuitton unexpectedly stole the show at the biggest global football tournament.
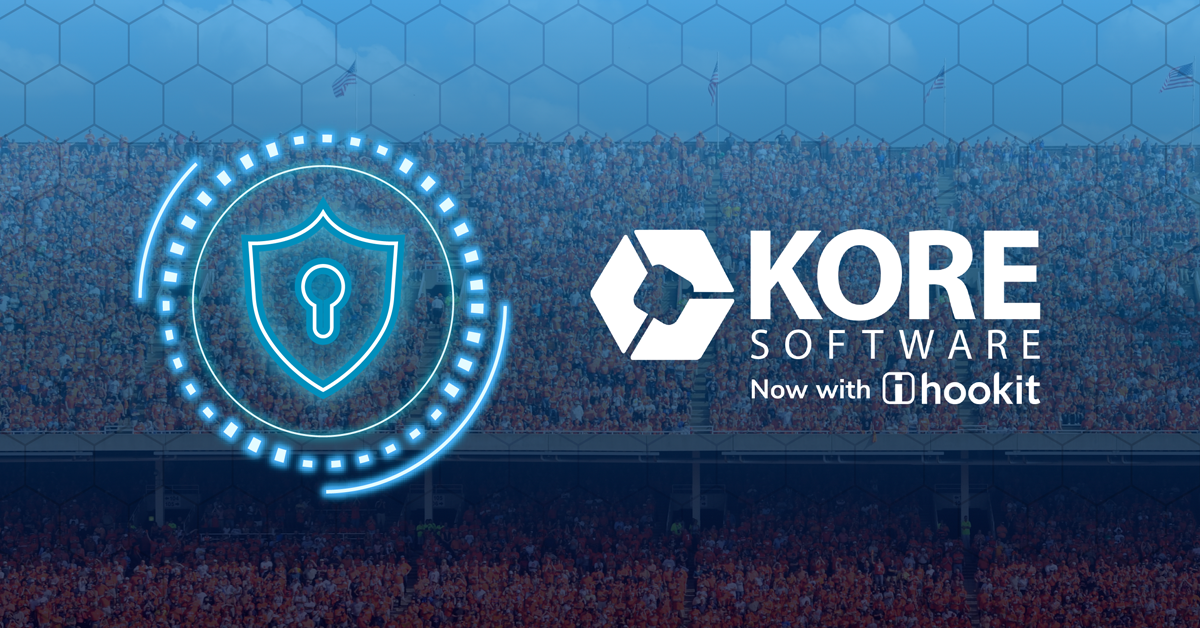
Unlock the Power of KORE in 2023
Heading into the first quarter of a new year is the perfect time to reflect on your team or organization’s objectives and goals, and how
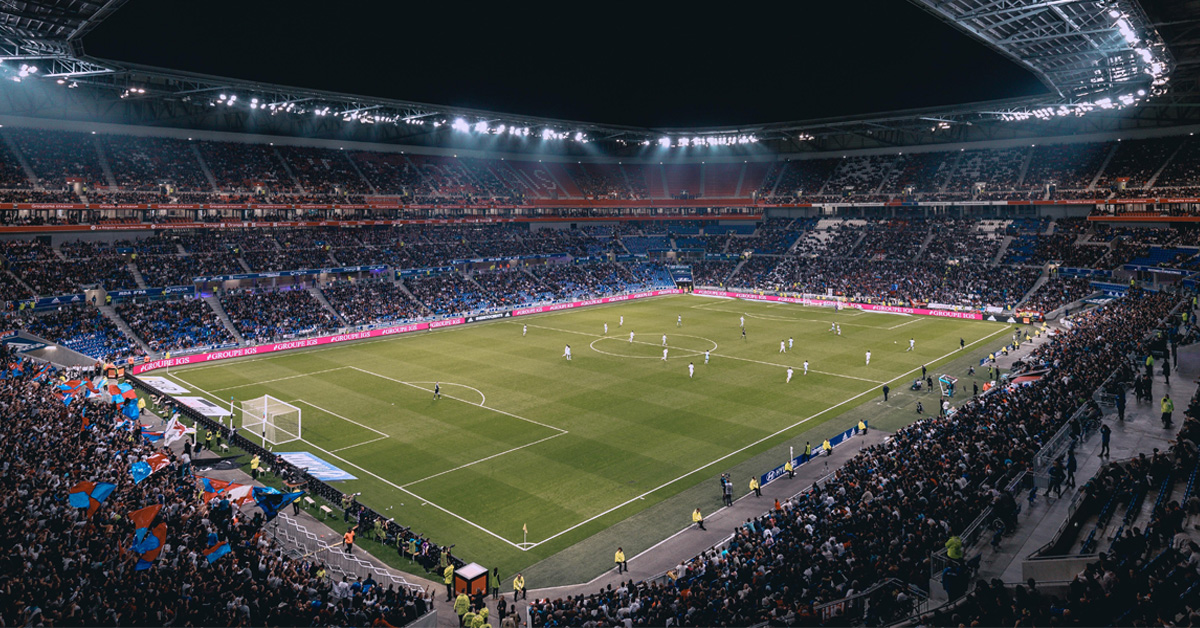
Unlocking the Power of Data-Driven Sponsorship Workshops: 4 Ways to Leverage Market Intel on Global Football
In our data-driven world, partnerships are more powerful than ever before. If you work for a brand in the sports or partnership landscape it’s important
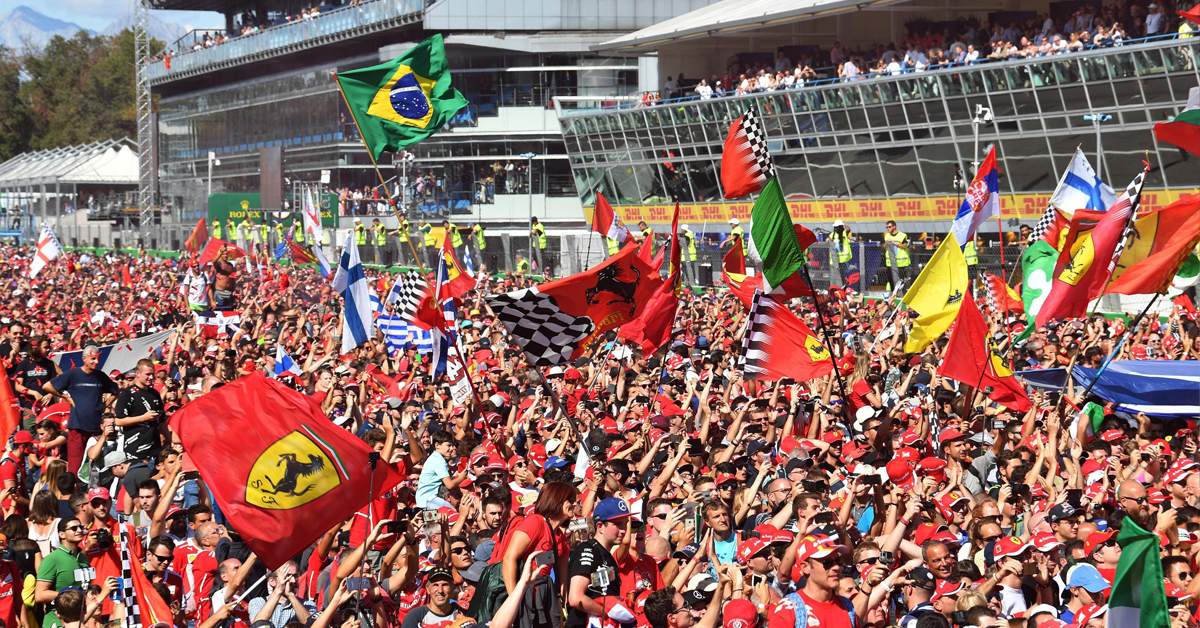
KORE’s 2022 Formula 1 Market Intel Recap: The State of the F1 Opportunity
Formula 1 (F1) has been a rising star in the global sports market for some time now, but its recent growth across digital channels and
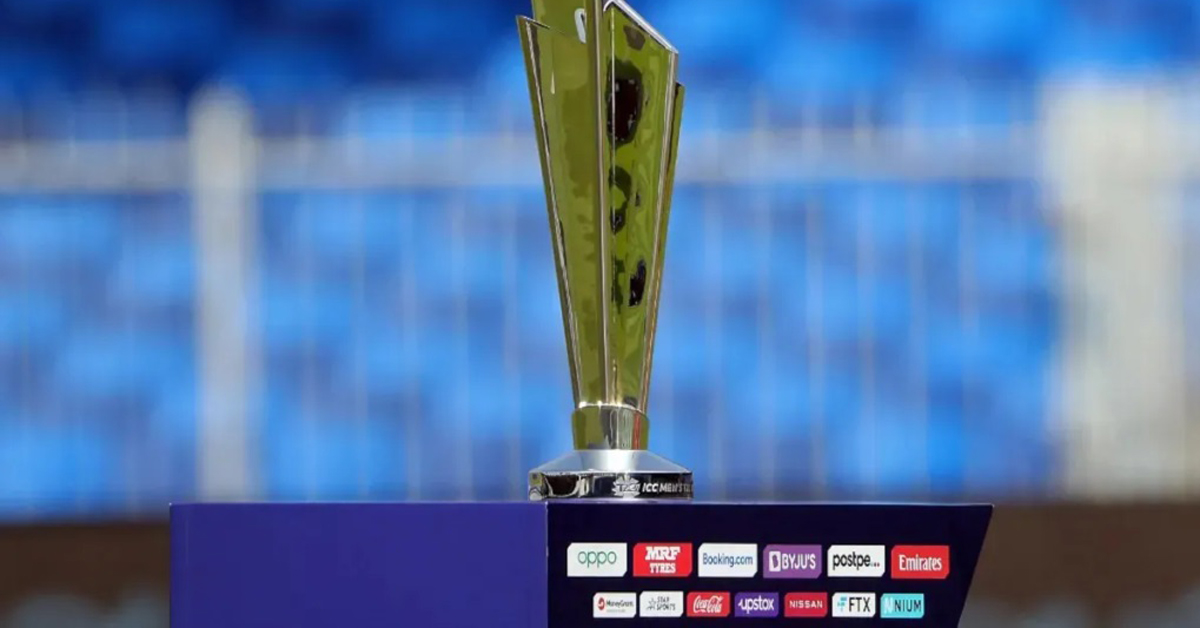
2022’s Cricket World Cup Social Engagement Kept Pace with the Last Olympics: A Short Twenty20 Social Recap
Cricket had one of its most engaged audiences ever. A quick comparison of 2022’s Cricket Twenty20 World Cup social data to the 2021 Olympics in
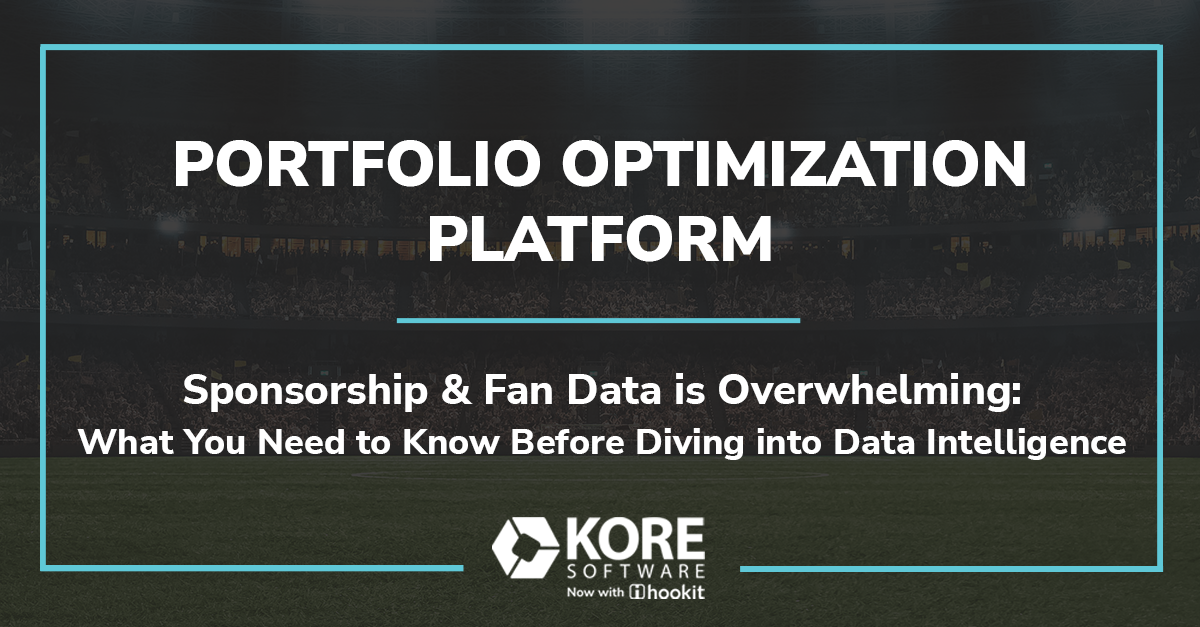
Sponsorship & Fan Data is Overwhelming: What You Need to Know Before Diving into Data Intelligence
When it comes to sponsorships, it’s important to take things slow and steady in order to get the most out of them. This means taking
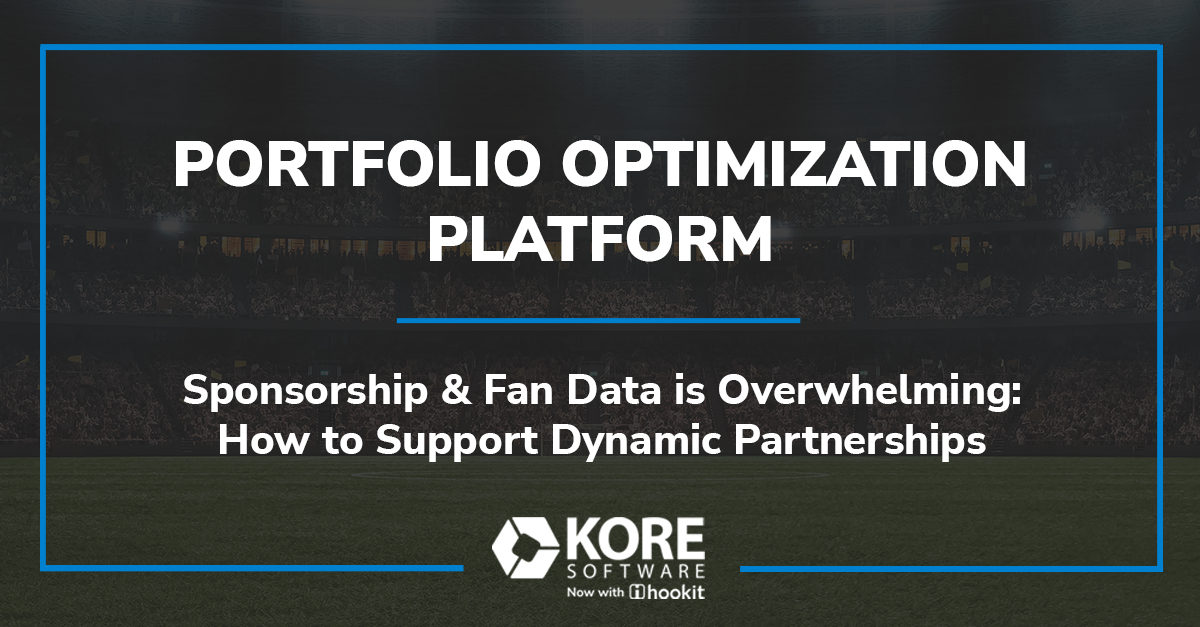
Sponsorship & Fan Data is Overwhelming: How to Support Dynamic Partnerships
Introduction The sponsorship world is evolving as fast as technology. It’s hard for anyone to keep up. This means it’s critical for brands to learn
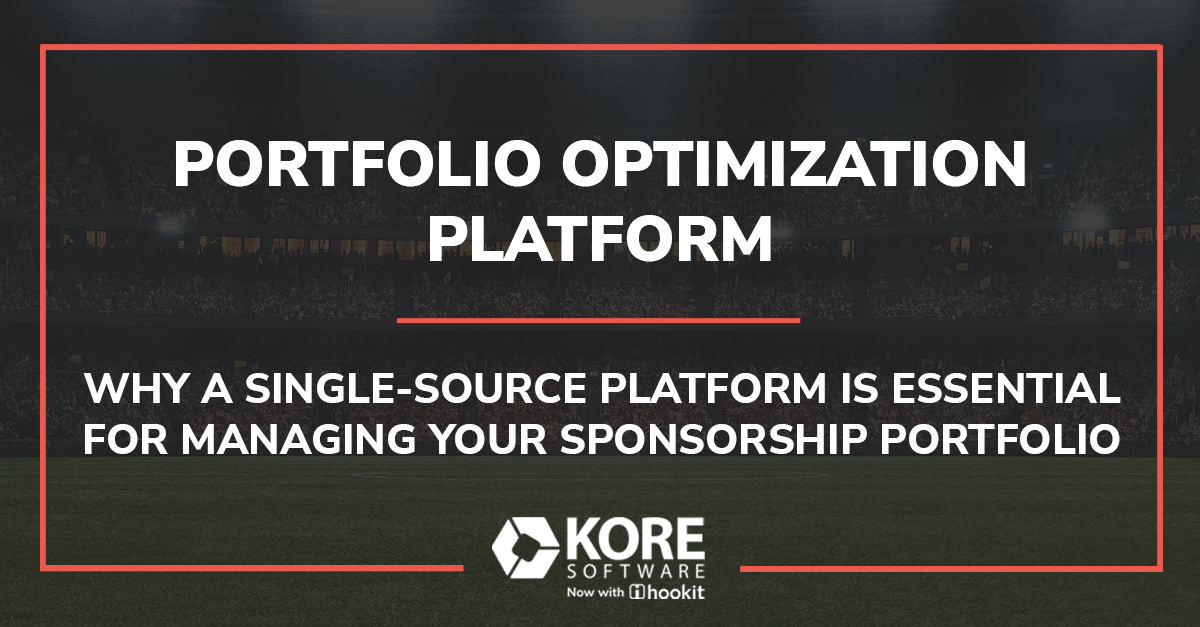
Why a single-source platform is essential for managing your sponsorship portfolio
Introduction One of the most common complaints we hear in the sponsorship industry is from brands that are trying to measure ROI with no source
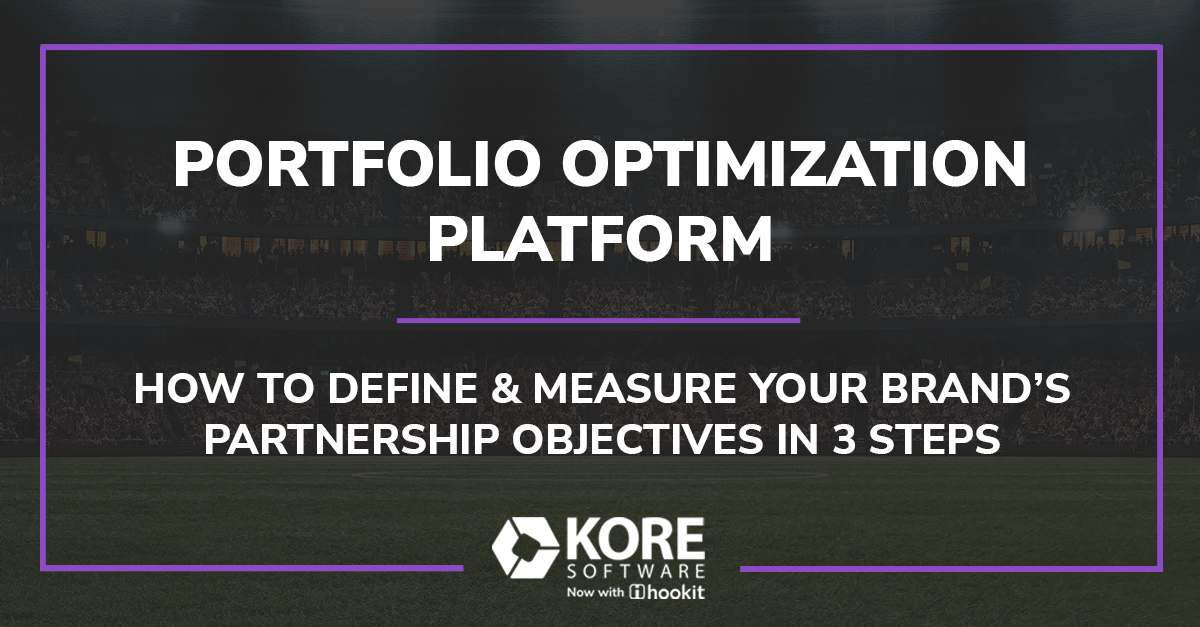
How to Define & Measure Your Brand’s Partnership Objectives in 3 Steps
Introduction If you’re strategizing how to get the most out of your partnership, you’re not alone. With $57B being spent globally on sponsorships, forecasted to
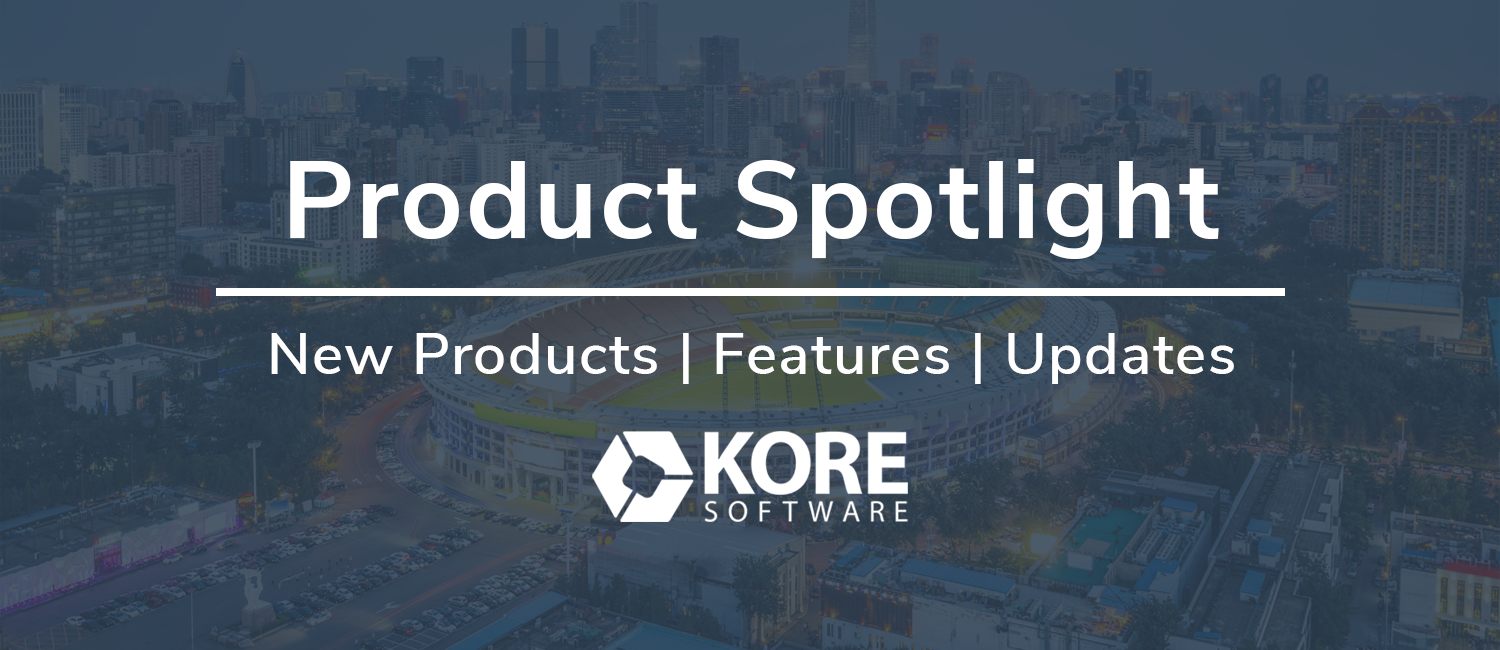
Empower Automated Customer Journeys By Syncing Golden Records to Your CRM
We’re making Helix even more powerful— integrate Helix with your CRM so that golden records are synced daily and available where you need them most.
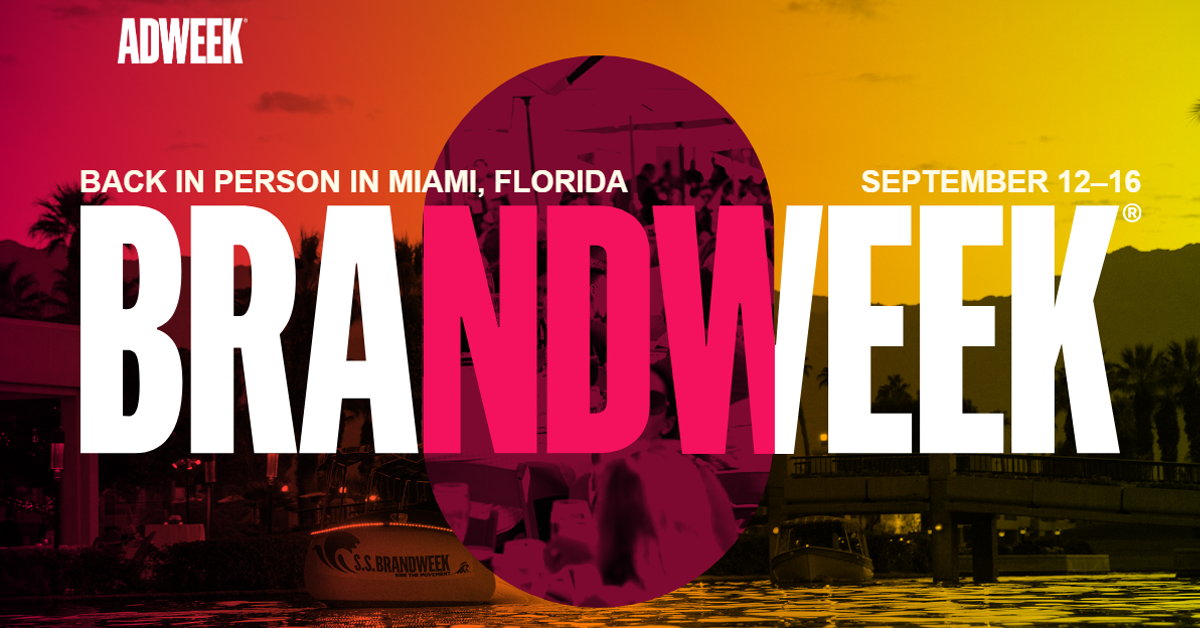
Brandweek Retrospect: The Pressure of Justifying Partnership Investment, Explained
We understand that the unique value of an investment in sports and entertainment sponsorships is gigantic, and we understand that it’s hard to measure. From
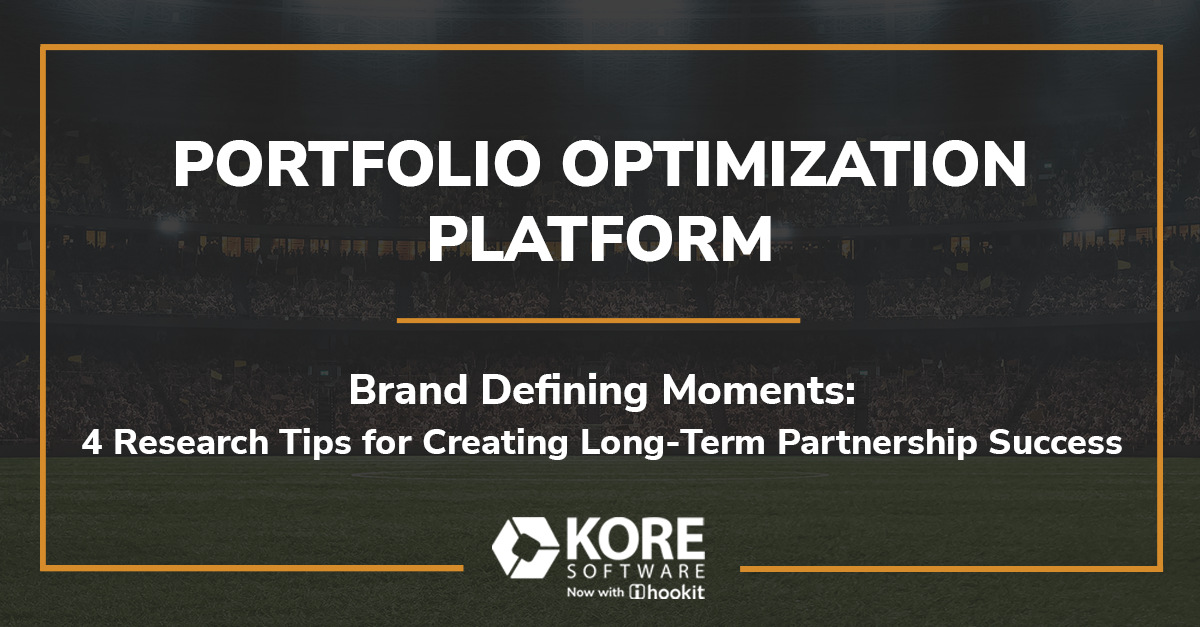
Brand Defining Moments: 4 Tips for Creating Long-Term Sponsorship Partner Success
One of the most common planning frustrations we hear from brands is that instead of researching partnership best fits, they heavily depend on the timing
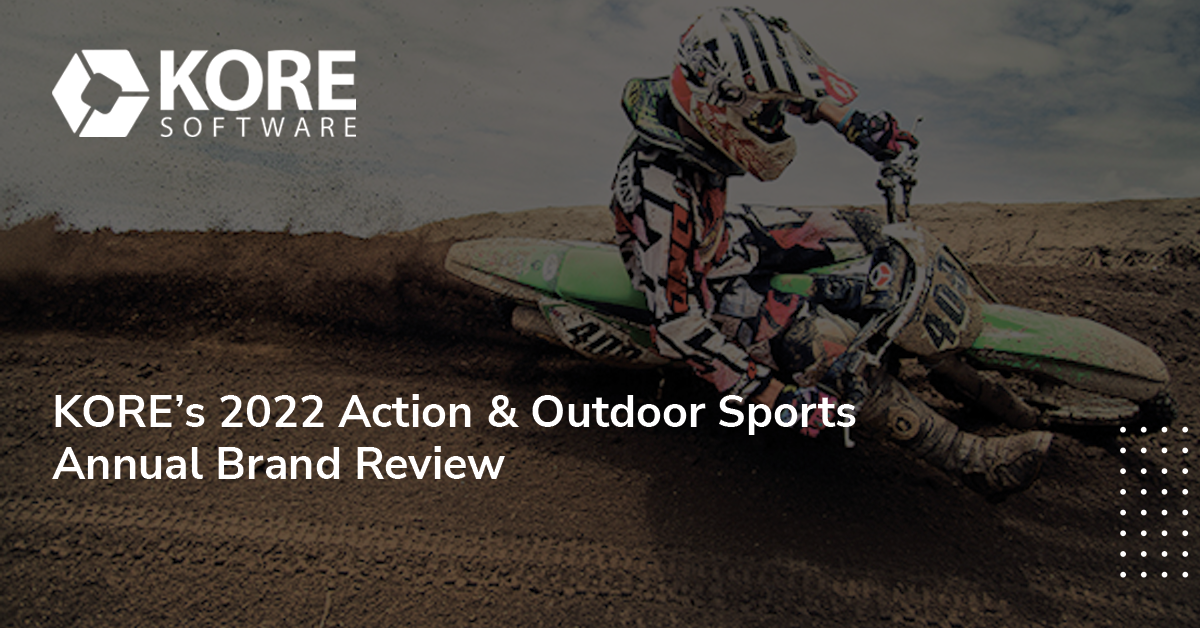
KORE’s 2022 Action & Outdoor Sports Annual Brand Review
Learn which brands are being promoted the most across action sports, who’s creating the highest sponsorship value and fan engagement, and what topics their fans
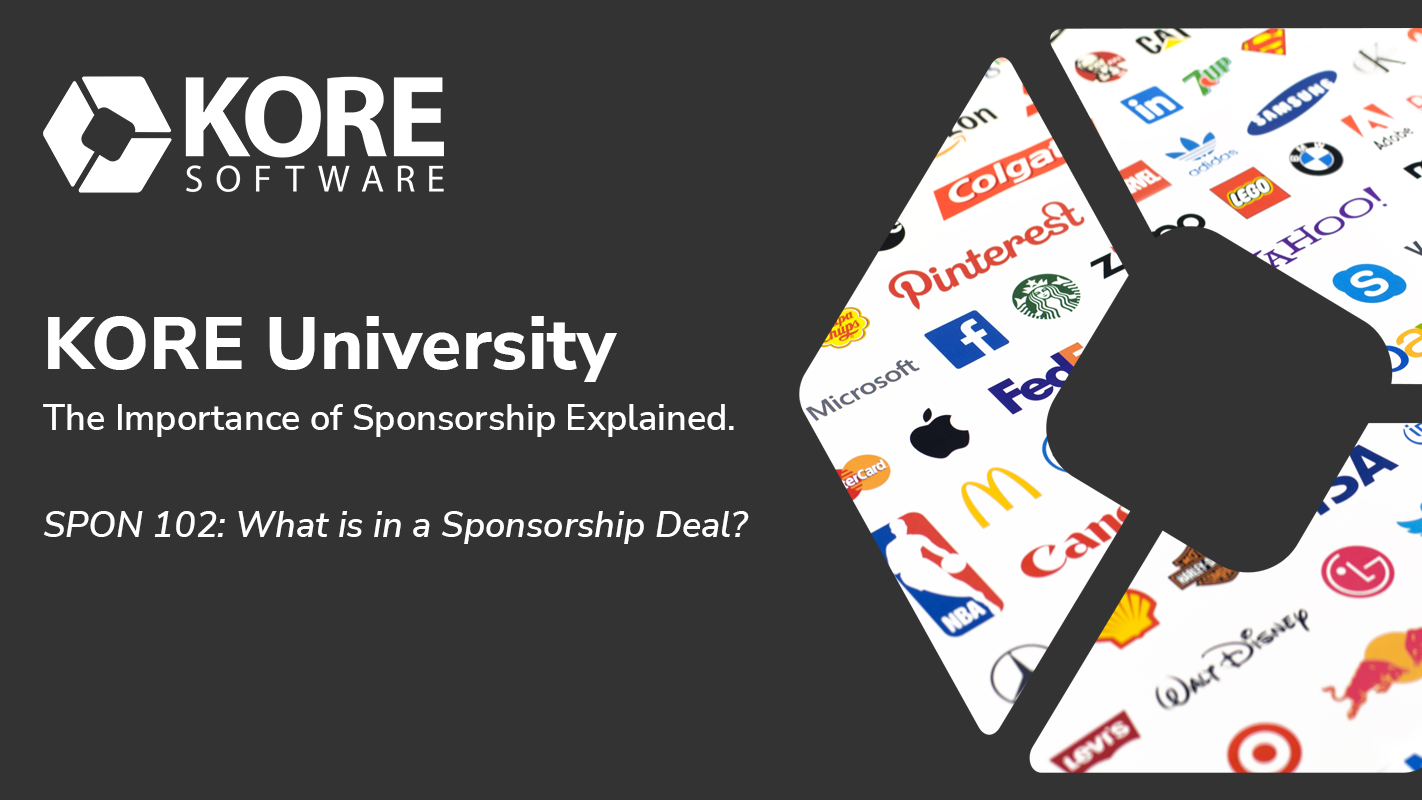
KORE University: What is in a Sponsorship Deal?
If you work in sponsorship, marketing, brand, sports, media, or a similar role, then our sponsorship series is for you. Come along as our sponsorship
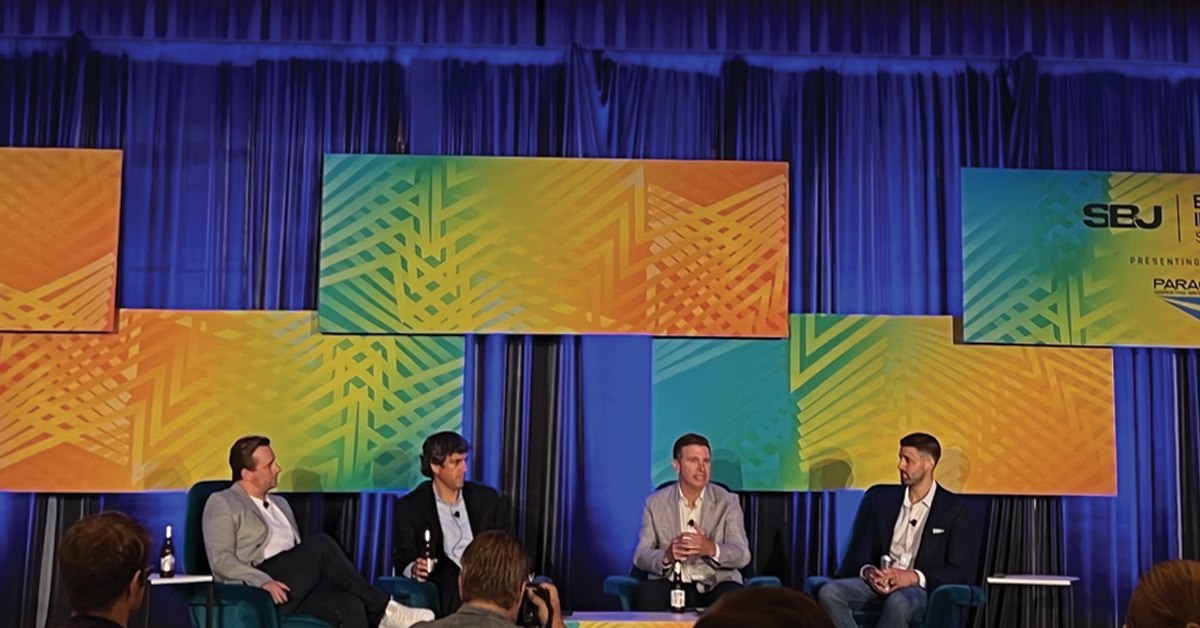
Top 3 Key Takeaways from Sports Business Journal’s Brand Innovation Summit 2022
Here, we lean into the most buzzworthy topics at the 2022 Sports Business Journal’s Brand Innovation Summit. From data predictions, ideation and innovation constantly in
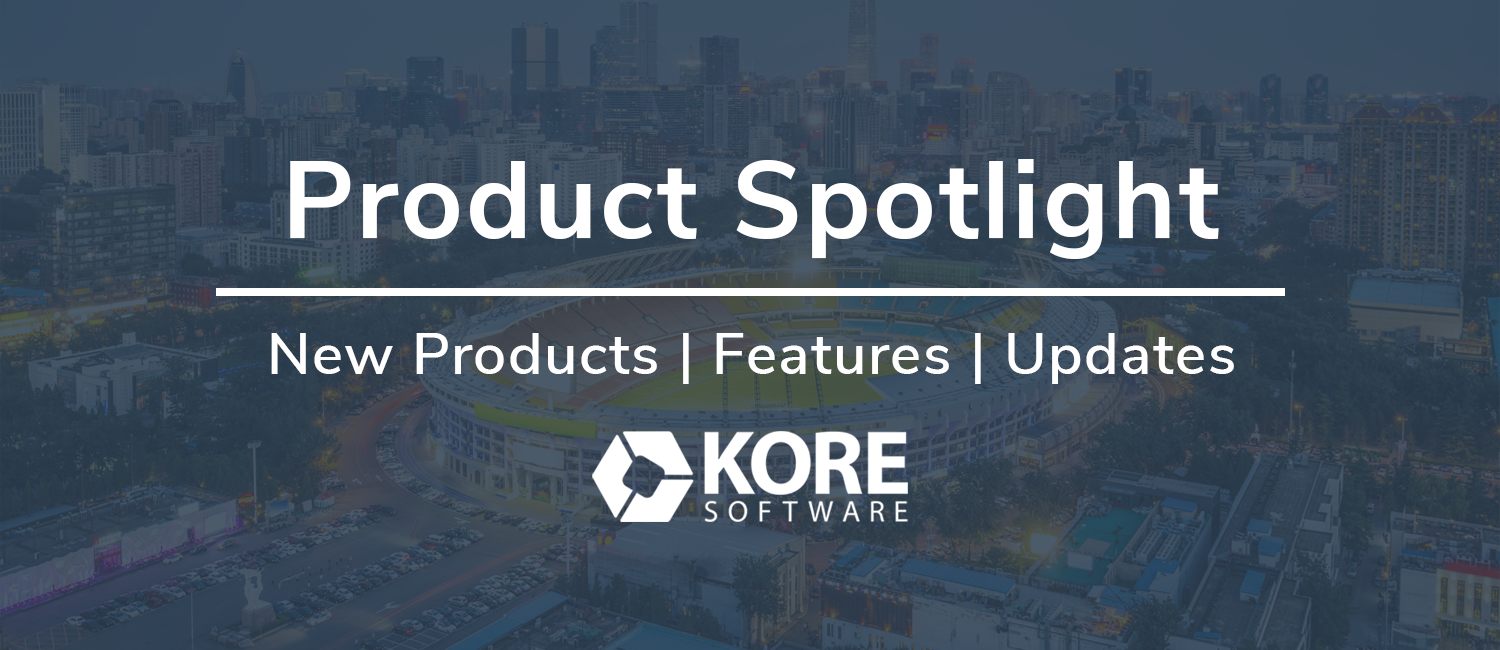
Capture & Analyze Historical Data to Make Smarter Business Decisions
Did we put you to sleep with “analyze historical data” in the title? We know it sounds boring but hear us out – data snapshotting
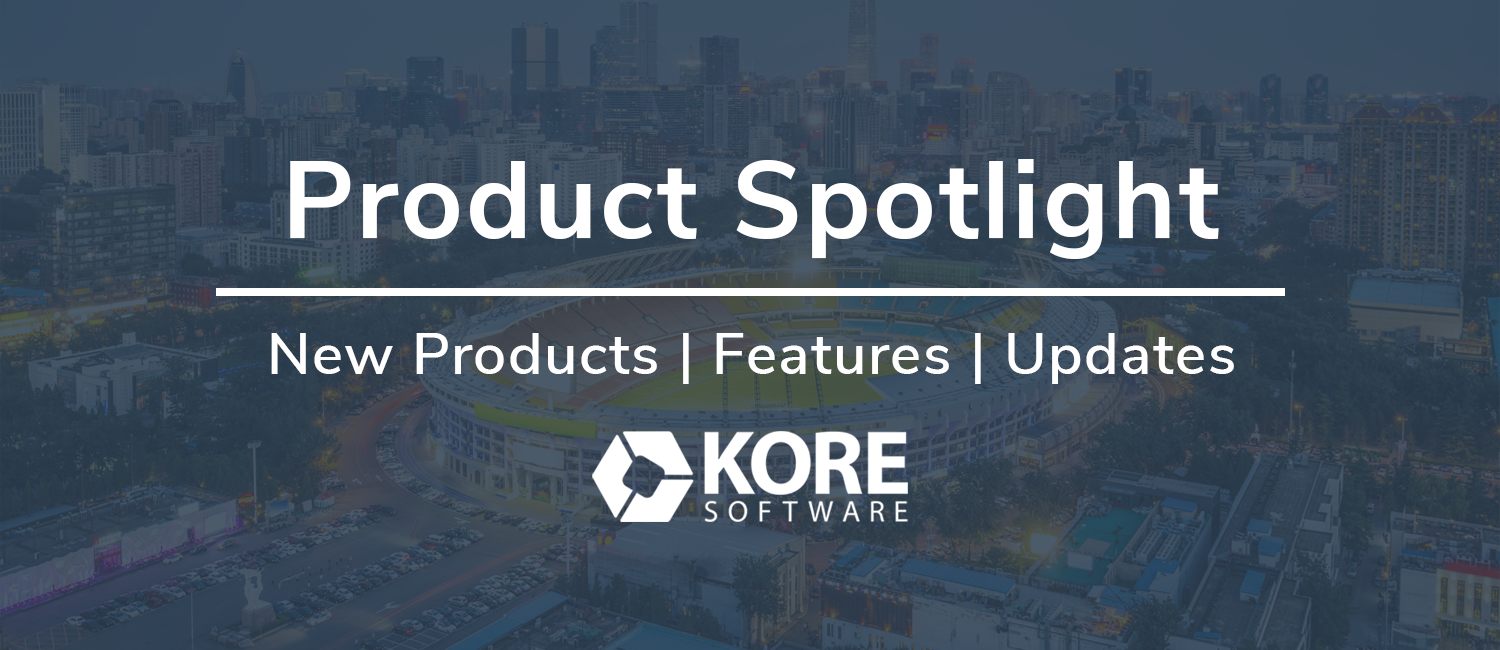
Introducing Helix: KORE’s Cutting-Edge Fan Intelligence Platform
Fans are your biggest asset, so how do you leverage their data? Fans are the biggest asset for teams, leagues, and venues. Not just because
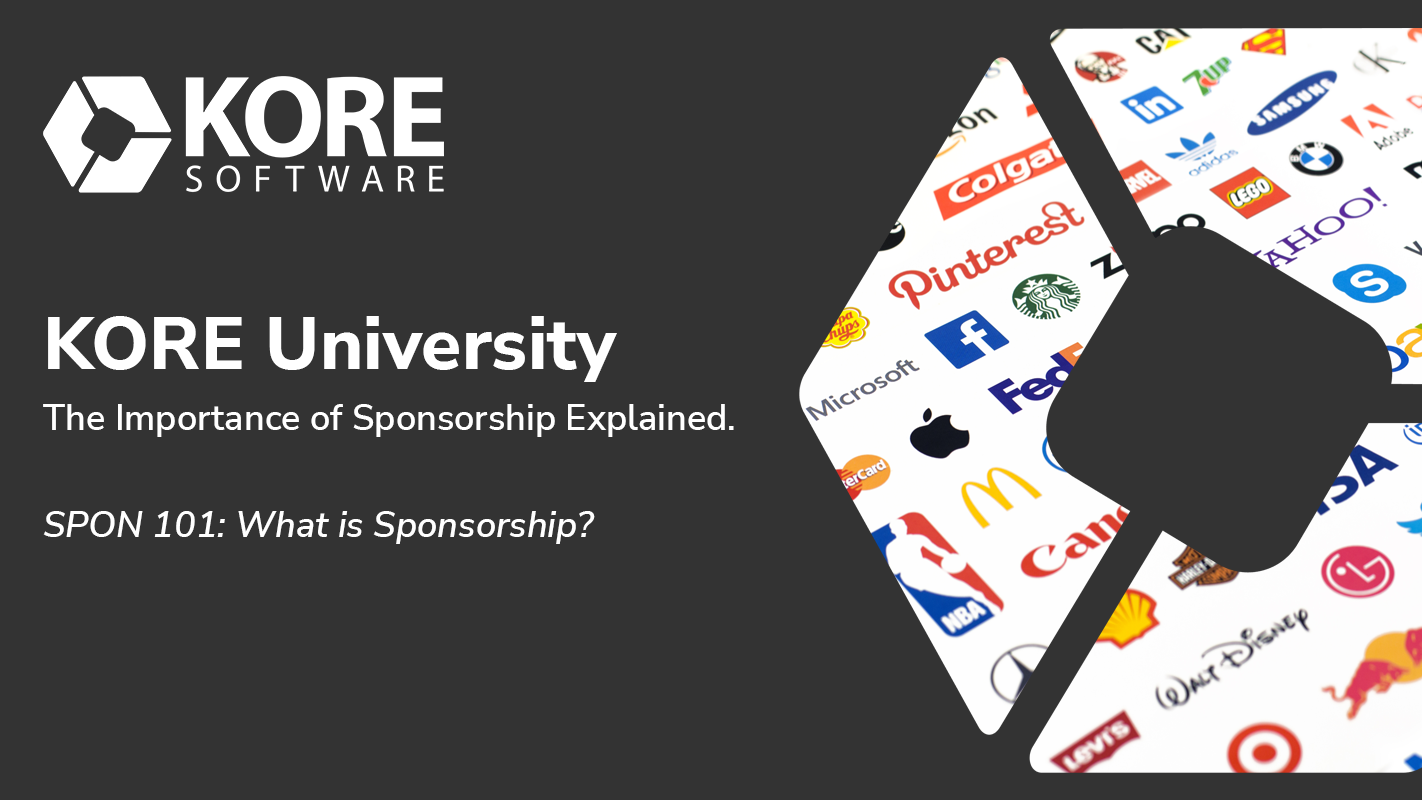
KORE University: What is Sponsorship?
Here at KORE, we geek out about sponsorship analytics, data, and ROI. Often, we can get really into the weeds about performance metrics, objective setting,
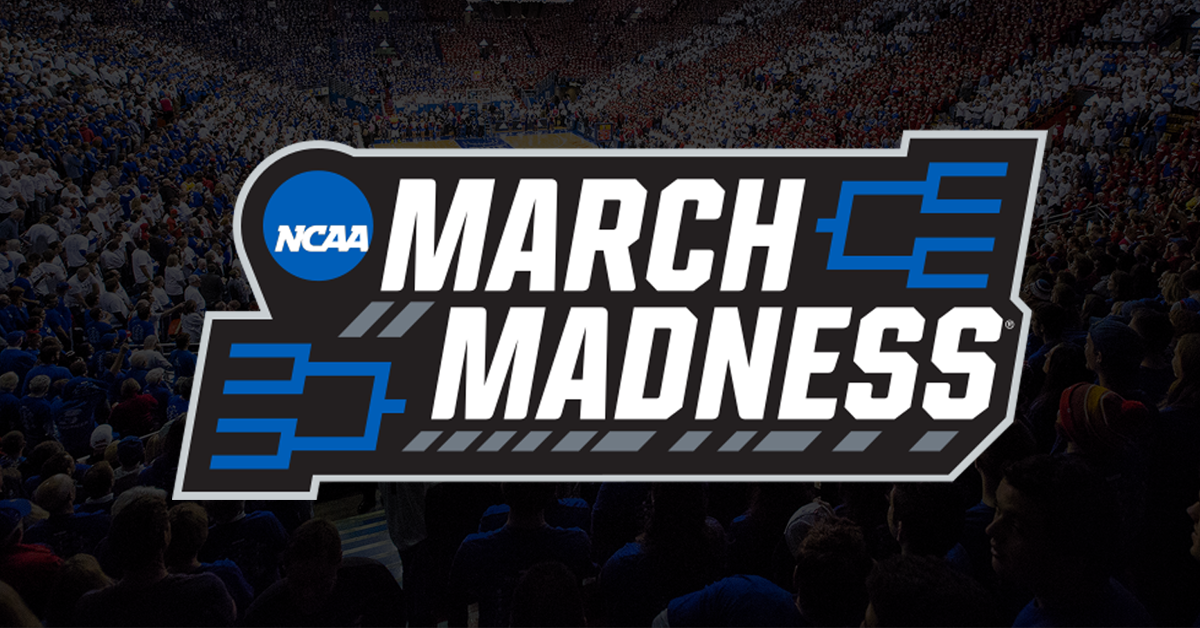
2022 March Madness
‘Tis the season, and by season we are of course referring to the season of March. To the outside world this might be considered just
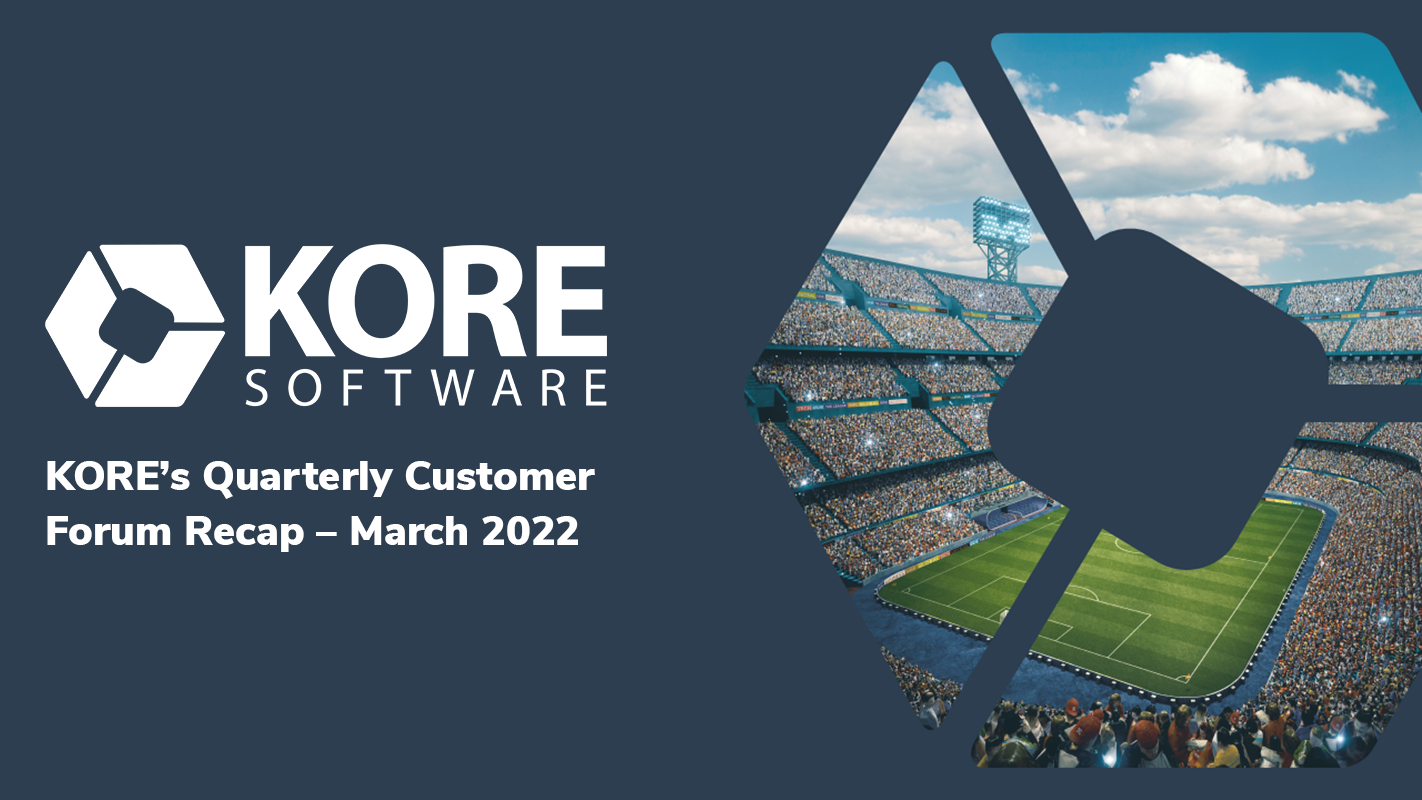
KORE’s Quarterly Customer Forum Recap – March 2022
In our first quarterly customer forum of 2022, our new Hookit colleague shared exciting product updates, the Boston Celtics showed us how they’re using Helix
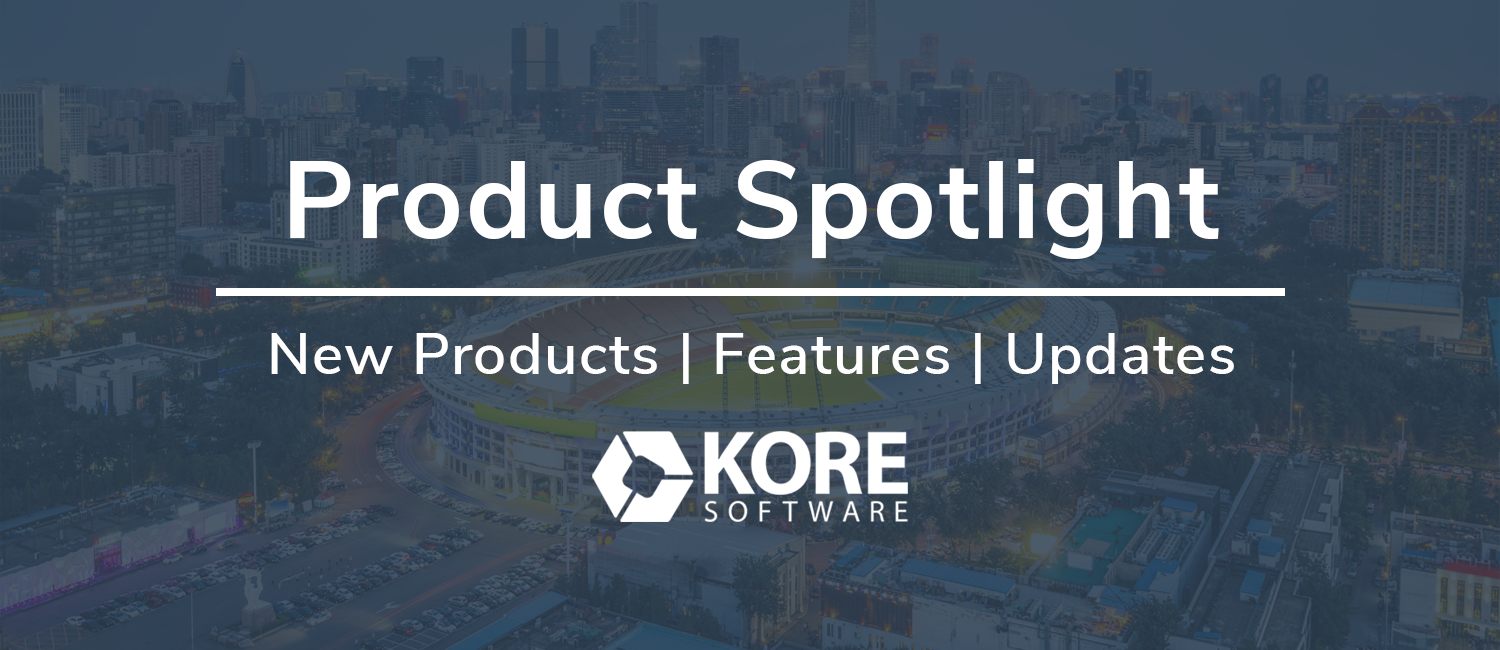
Sponsorships: Maximize Performance & Invest Smarter with the Partnership Data Submission Portal in Evaluate
Are you still waiting on end-of-year recaps 6 weeks after the season ended? Through our work with brands, rights holders, and agencies, we noticed a
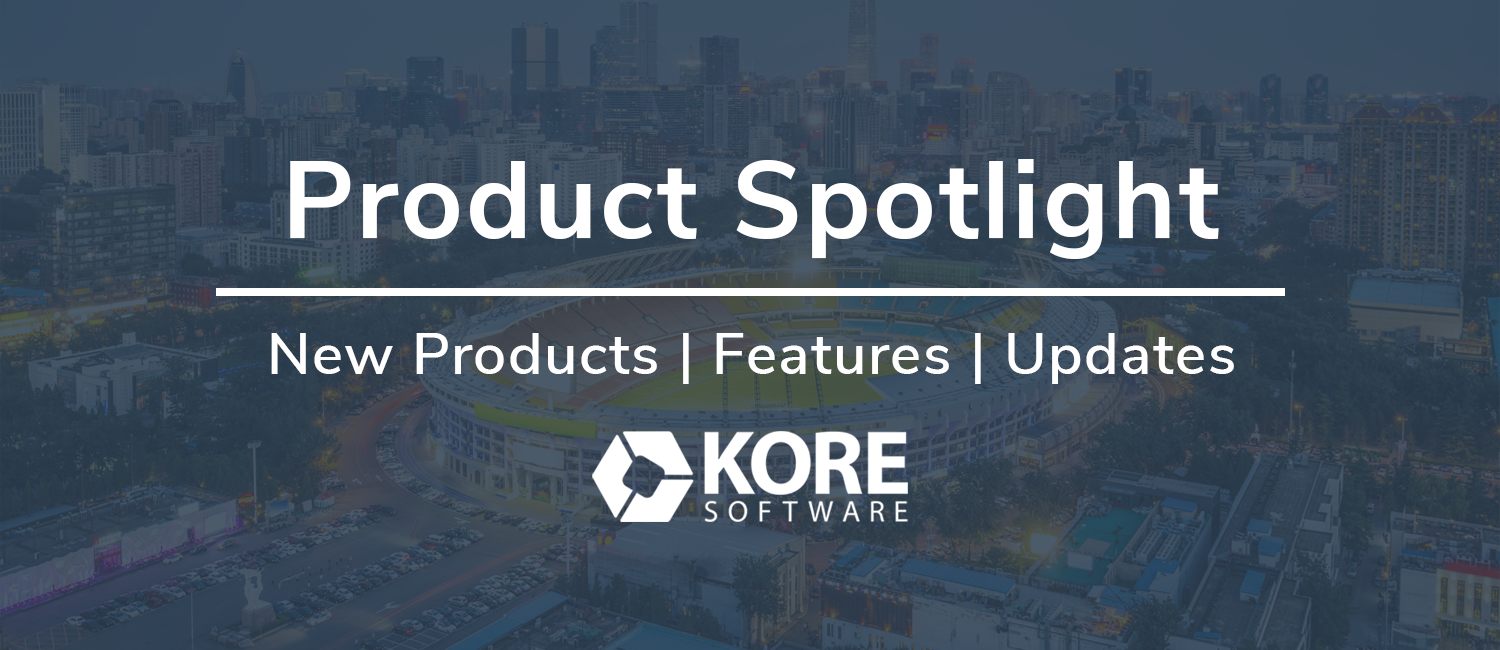
Activate Enhancements: Manage Every Partnership Detail in a User-Friendly Activation Platform
Last year, we shared the rebranding of KONNECT to Activate. Activate is a best-in-class activation platform that improves partner relationships by modernizing the way you
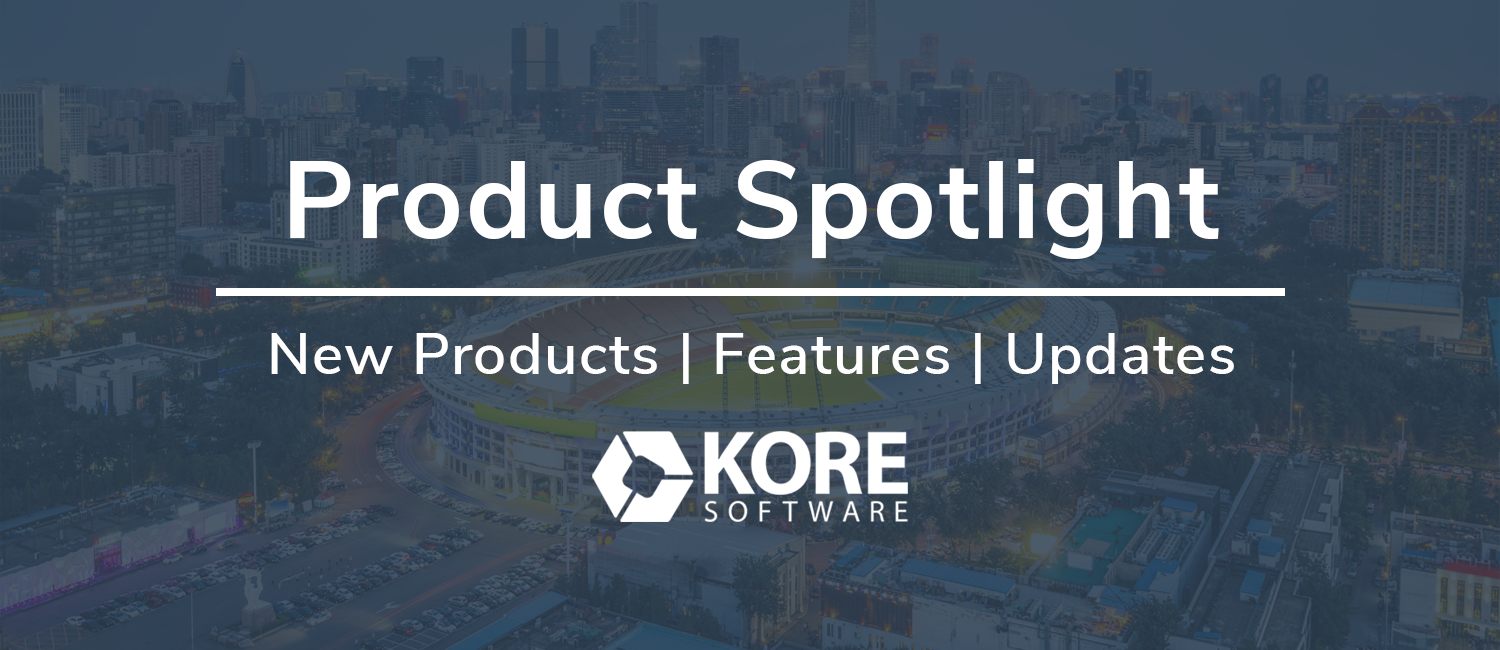
Cut Through the Noise to Find the Right Sponsorships with the Latest Intake Features
Way Too Many Sponsorship Applications in Your Email We’ve heard the horror stories. Email inboxes inundated with hundreds of sponsorship requests – 90-99% of which
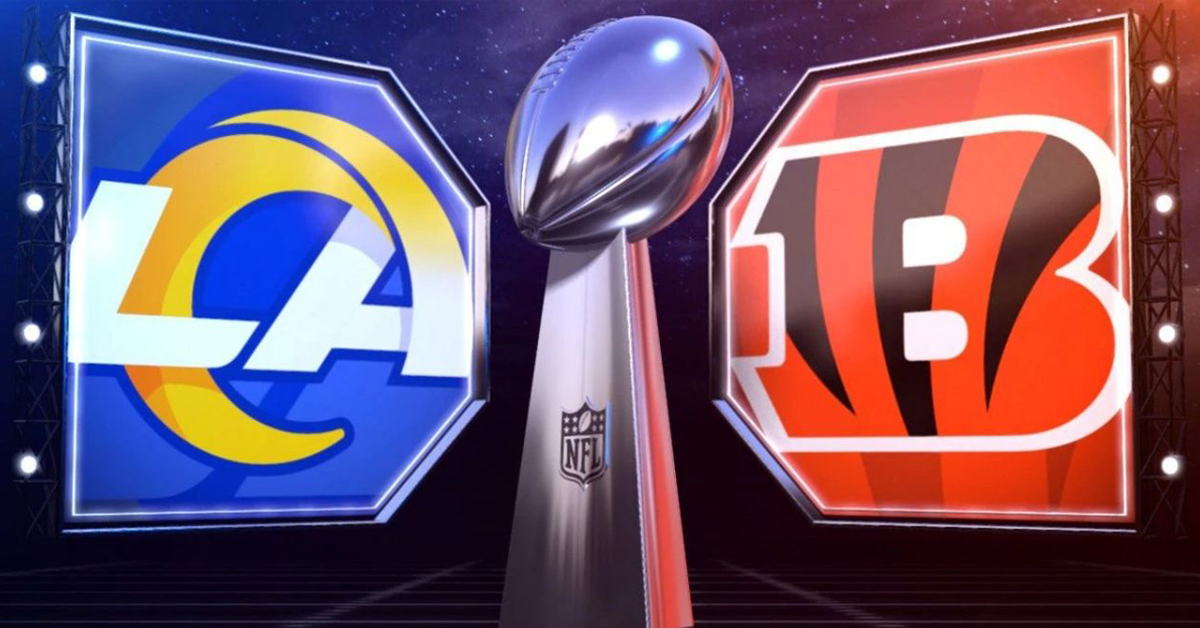
2022 Super Bowl Preview
Congratulations to the Cincinnati Bengals and the Los Angeles Rams on winning their respective conferences and facing off in the Big Game on Sunday, February
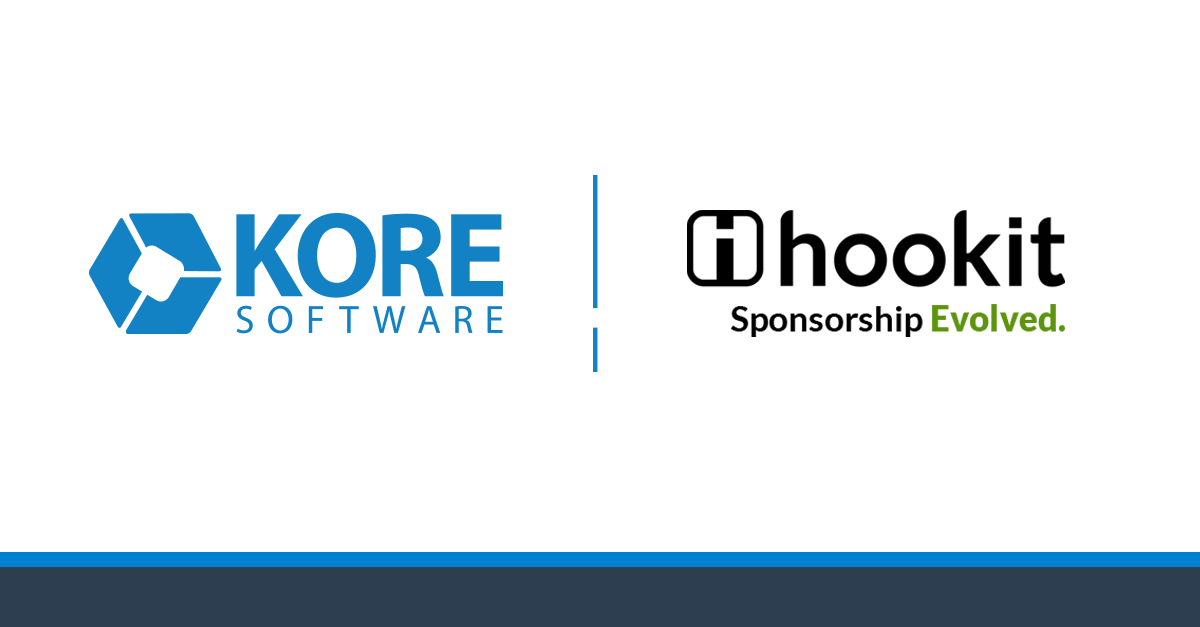
Message to Our Customers
We’re excited to share some important news with you. Today we announced that KORE Software is acquiring Hookit, the leading AI-powered sponsorship analytics platform, and
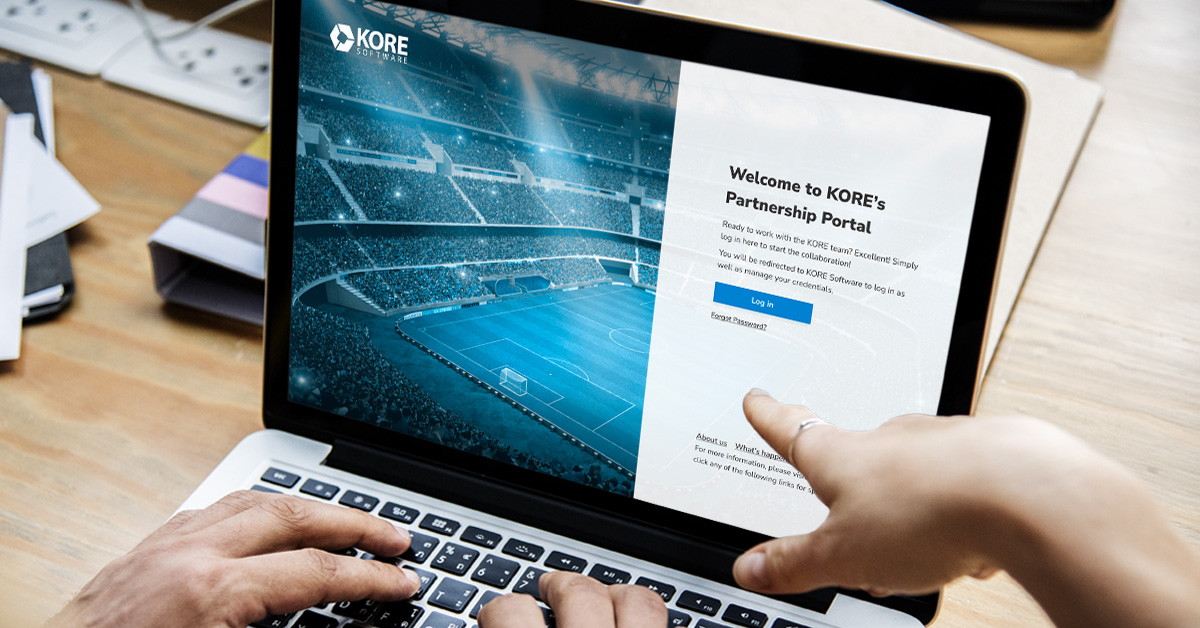
4 Essential Steps to Selecting the Right Partnerships
To assemble a winning partnership portfolio, brands must first choose the right partners. The right partners help to achieve key business objectives, improving both partnership
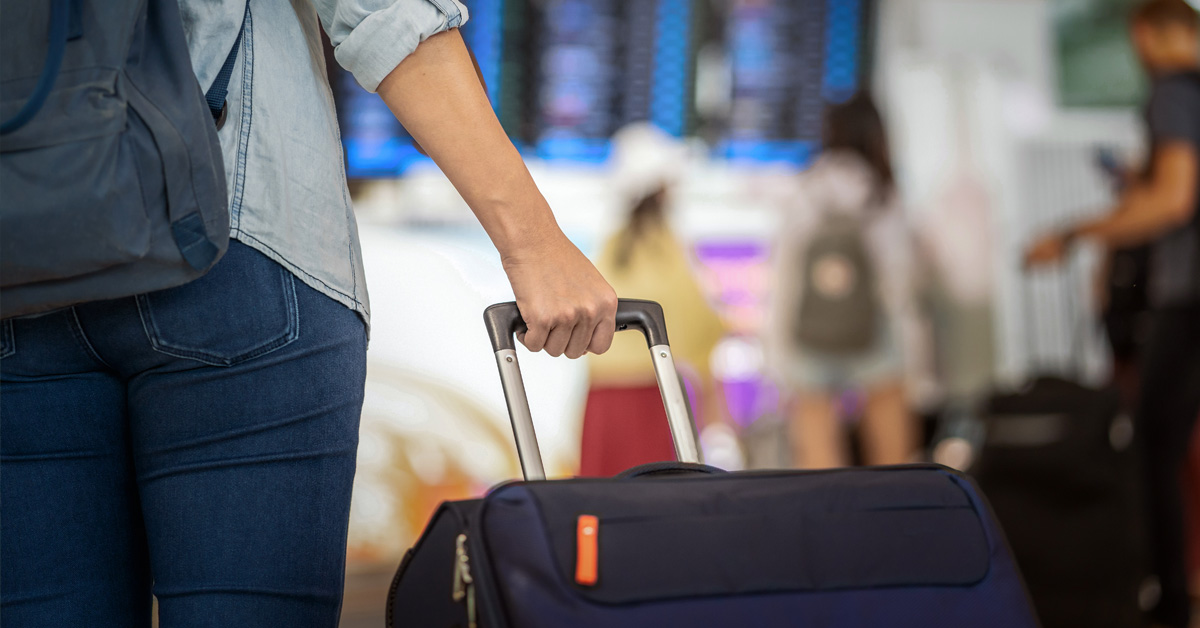
Tourism and the New Age of Engagement Marketing: How to Move Business Forward in Uncertain Times.
It’s no secret that the last two years have been challenging devastating for the travel and tourism industry. But as 2022 opens the door, this
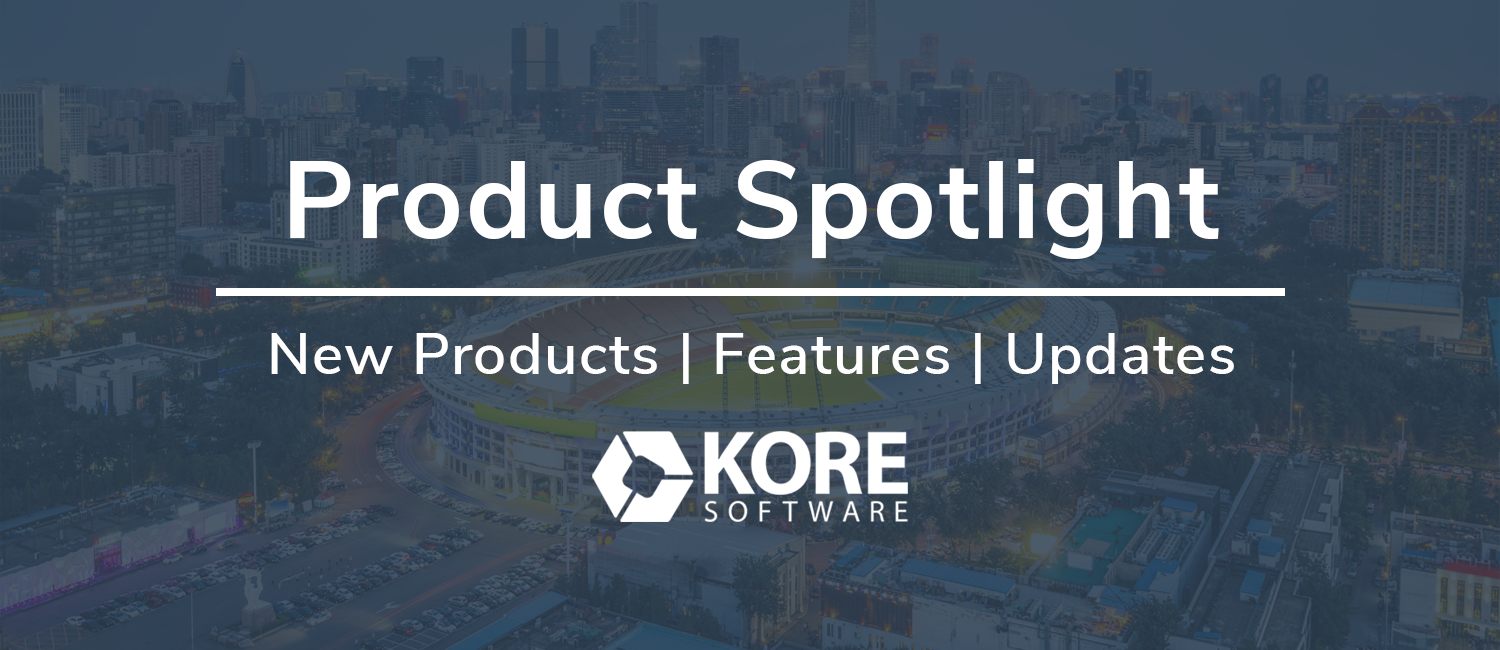
Top KORE Products of 2021
2021 was a big year for KORE – not only for our team, but for you and the hundreds of global sports organizations, brands, and
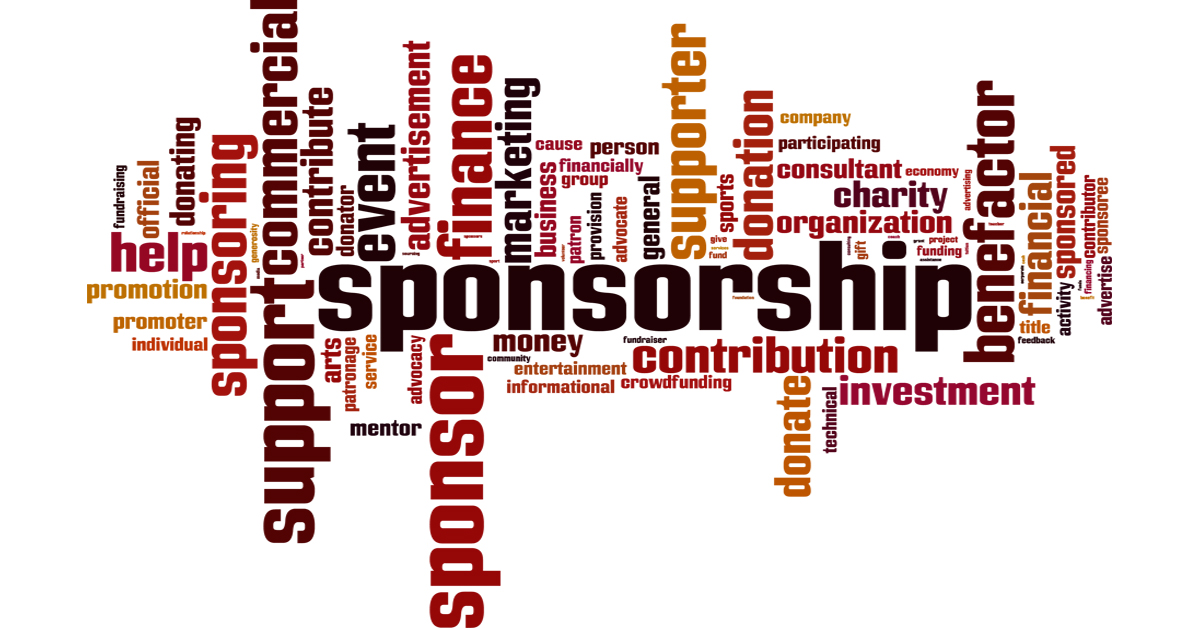
Top 4 Sponsorship Activations in 2021
Throughout 2021 we were slowly introduced to pre-pandemic times. Sporting events and other forms of entertainment opened their gates with limited capacity, all the while
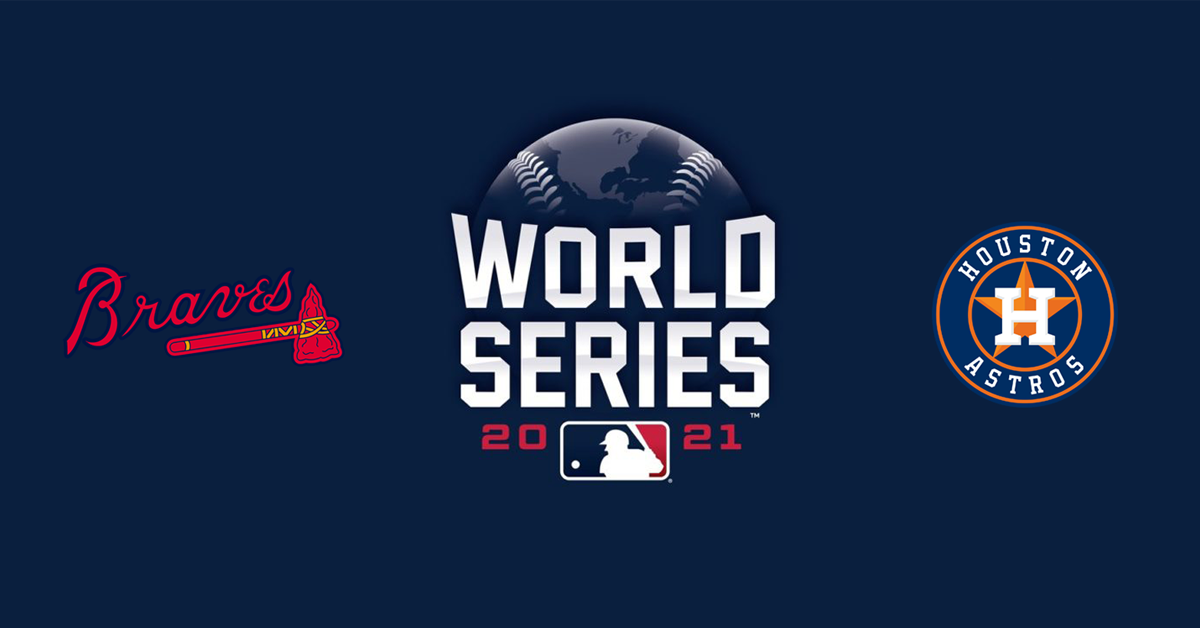
2021 World Series Review
Congratulations to the Atlanta Braves & Houston Astros on reaching the 2021 World Series! Both teams have a rich history and their respective fanbases have
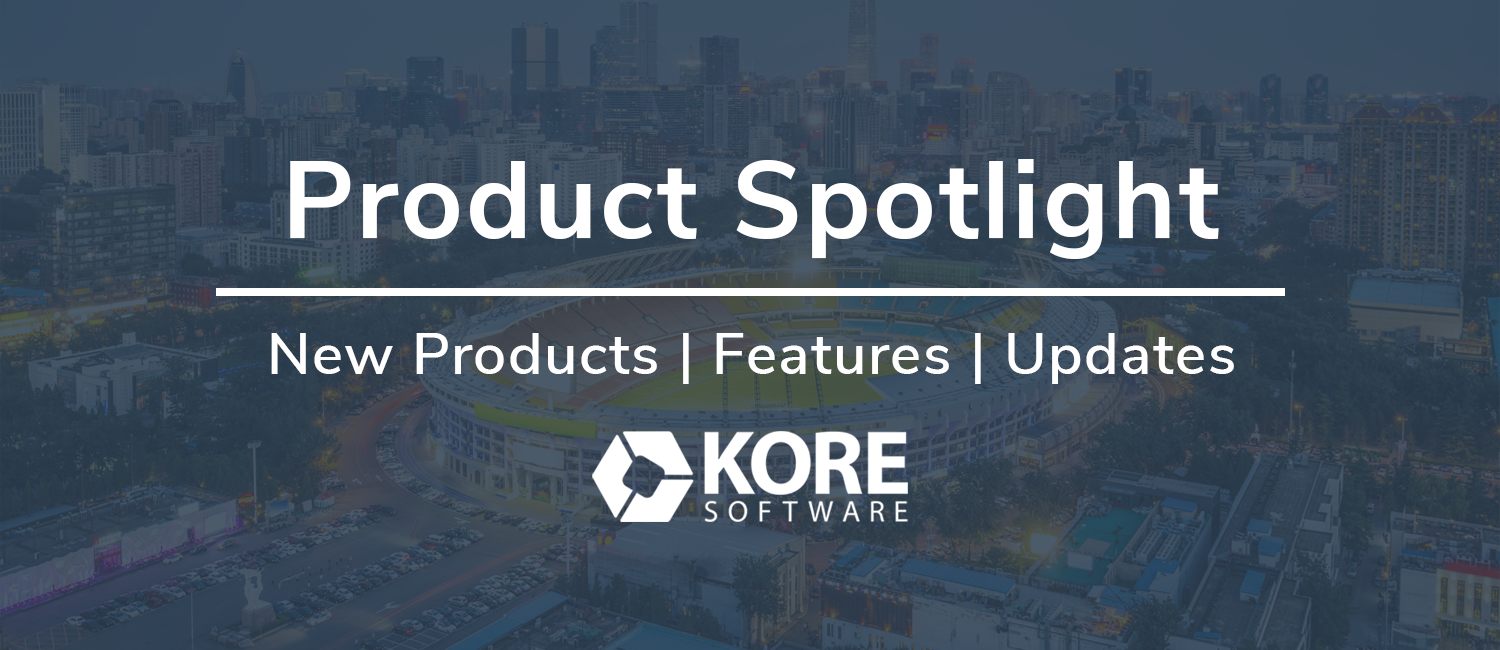
Partner Collaboration Made Easy With the New Tableau Connection
As we continue to revolutionize sponsorship recaps, we added the ability to connect your Tableau workbooks to your individual sponsorships in Activate. Connecting to Tableau
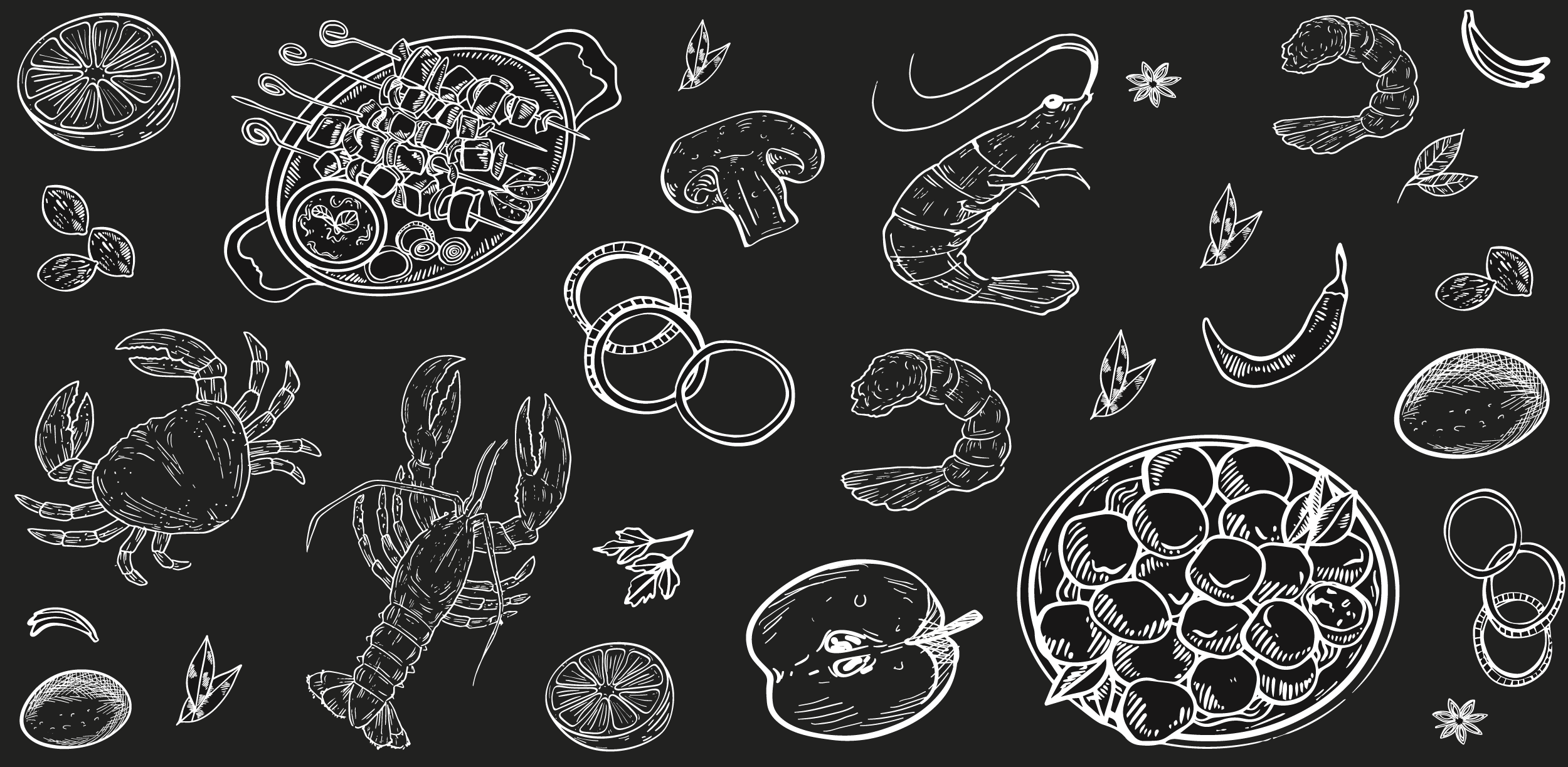
QSR Partnerships In Sports
The Fall sports calendar is as loaded as an Idaho potato at a Vandals tailgate. College football and the NFL are back, the NBA and
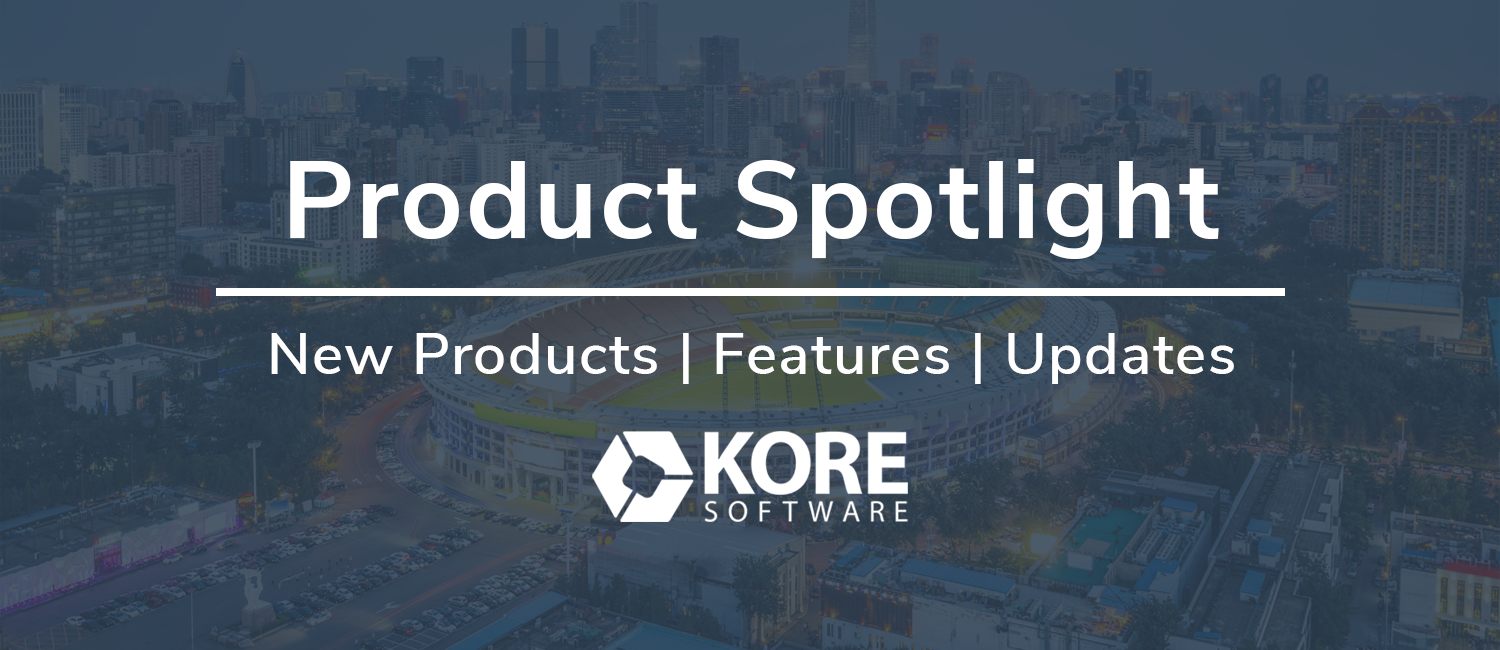
Modernize Your Sponsorship Recaps with New Features
If creating sponsorship recaps takes you countless hours, keep reading. The four new Advanced Delivery features in Activate make it easier to capture the true
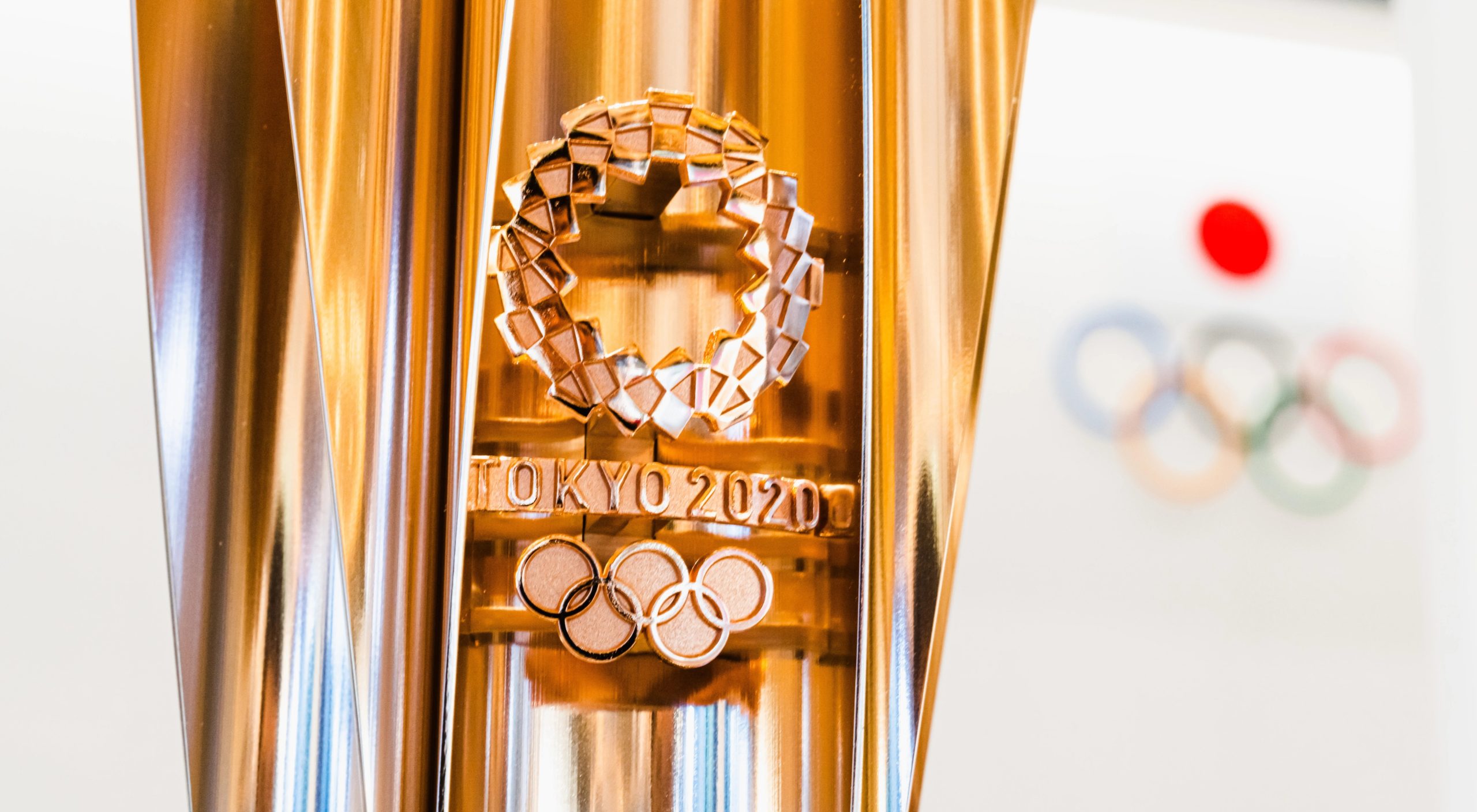
Tokyo 2020 in 2021: Broadcast and Sponsorship Breakdown
This summer’s Tokyo 2020 Olympic Games will surely be historic. Since the inception of the Modern Olympics in 1896, the Games have been cancelled only
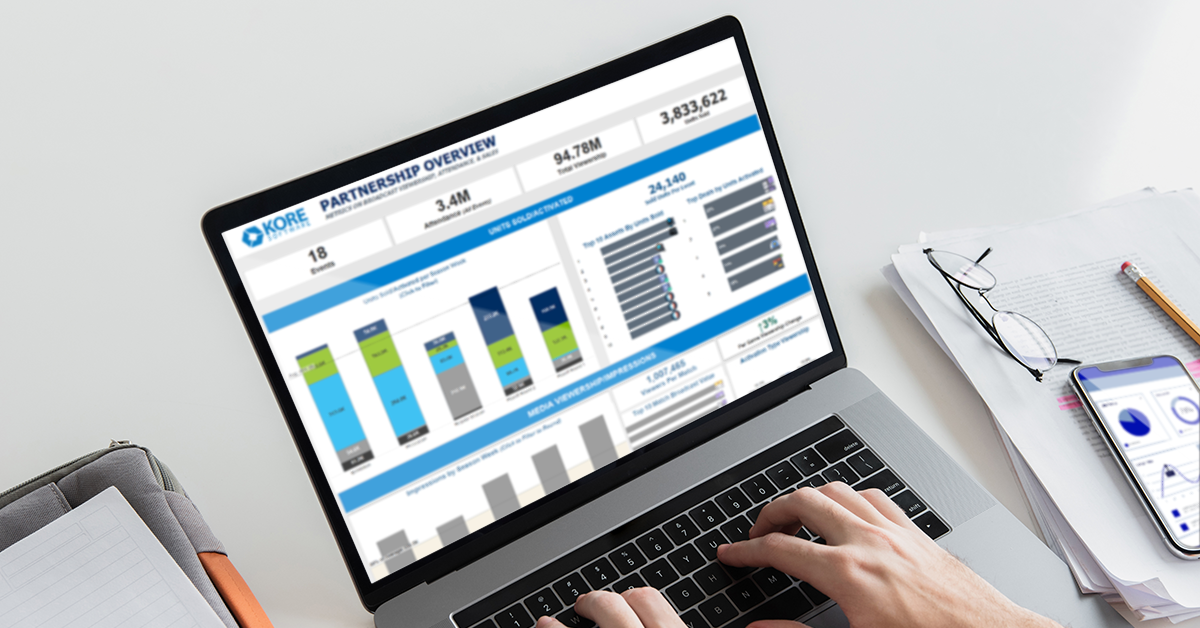
Deal Scoring – Comparing Investments Across Markets
Brands enter into multiple sponsorship deals because they want to increase brand awareness (among other objectives) across different audiences at different events. But the return
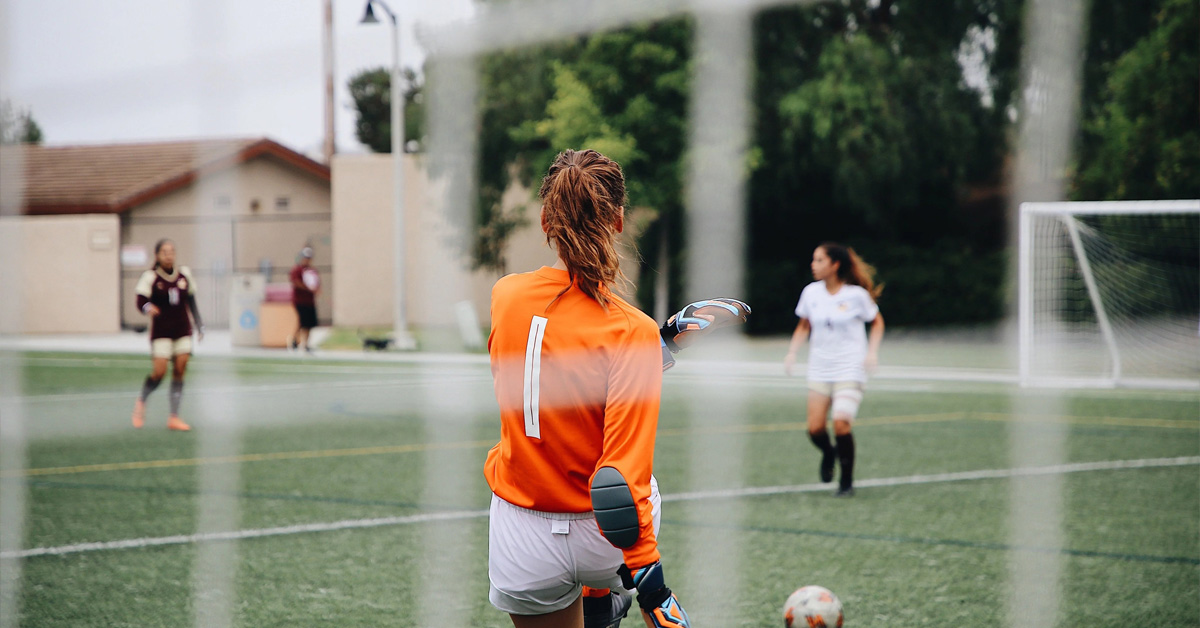
Name, Image, Likeness: New NCAA Rules Are On the Way
How companies, athletic departments, and athletes are getting ready to take advantage of the new rules. Across the many different NCAA sports and divisions, there
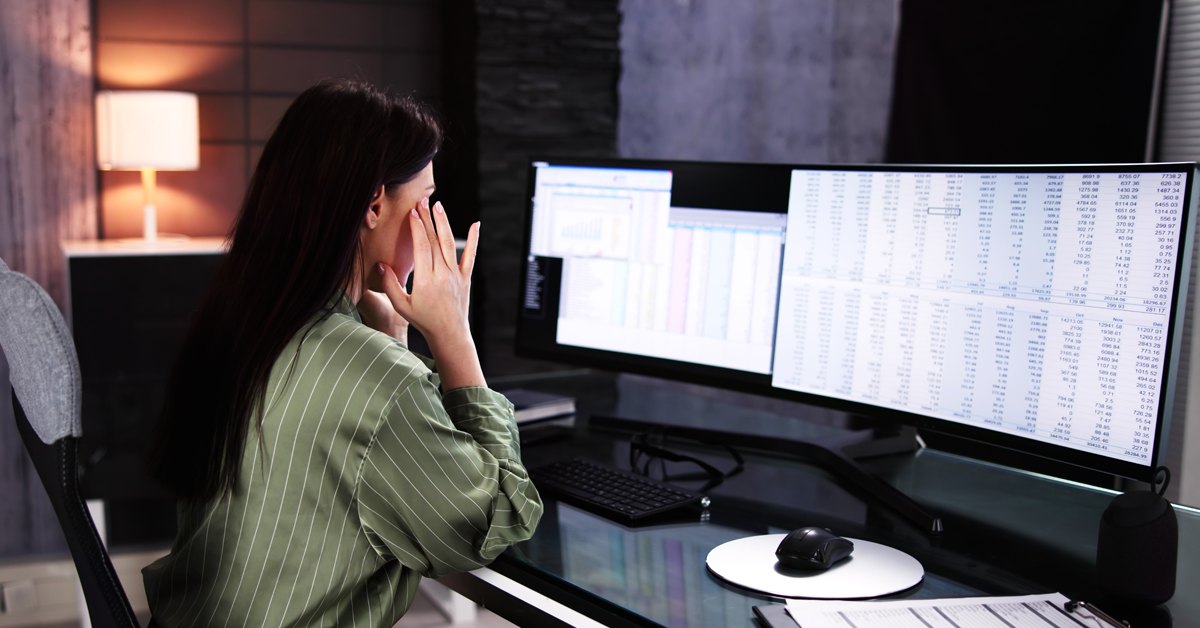
From Spreadsheets to Systems
When an organization first begins investing in sponsorships, it will most likely track the details in a spreadsheet. It’s a ubiquitous and easy way to
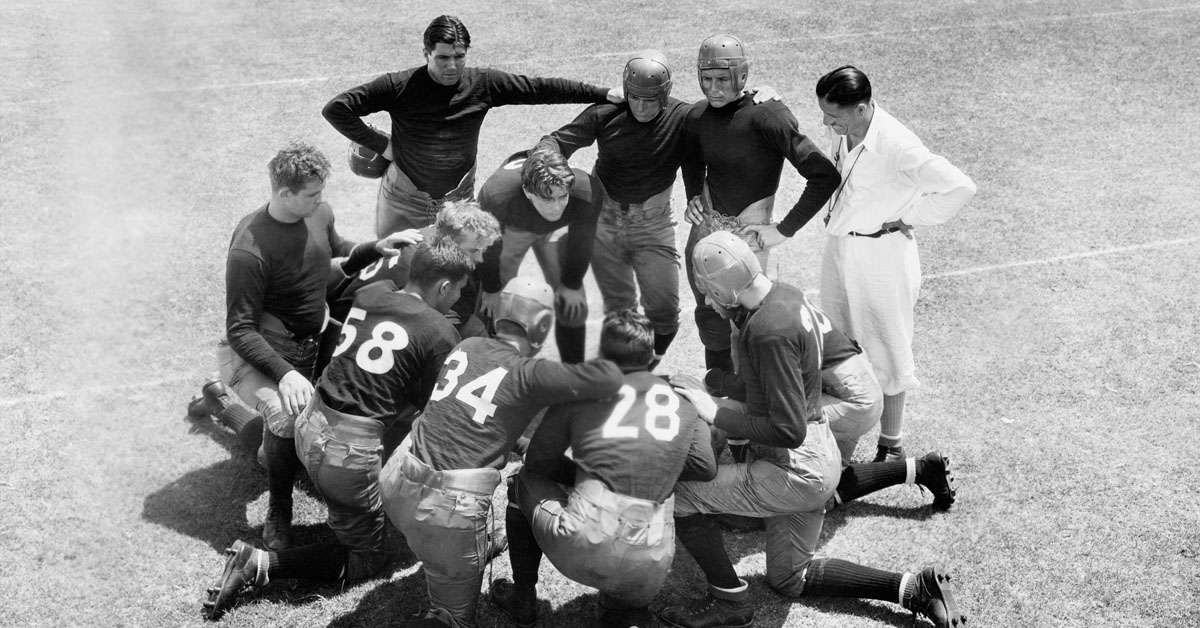
Is Your Organization Investing in the Right Partners?
Sponsorships are one of the oldest marketing tactics in the book, thanks to their long legacy of success. Since the earliest days of marketing and
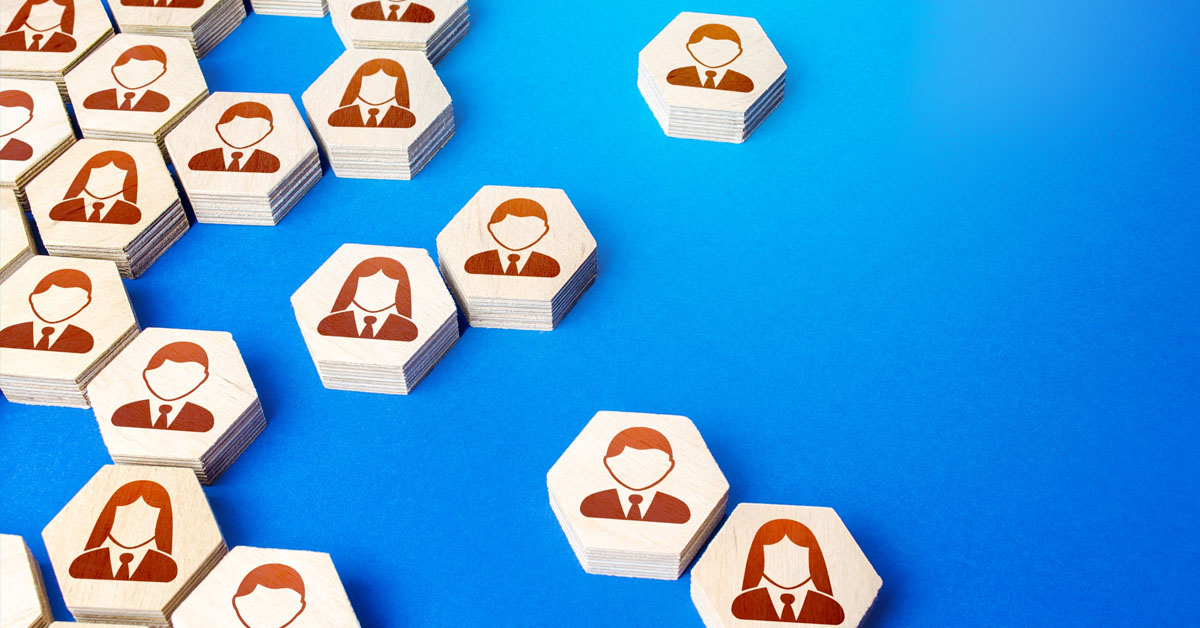
Building a Sustainable Sponsorship Department
Throughout a business’ lifecycle, there come times when the company needs to experiment and creatively change their practices and structure. Sports and entertainment companies are
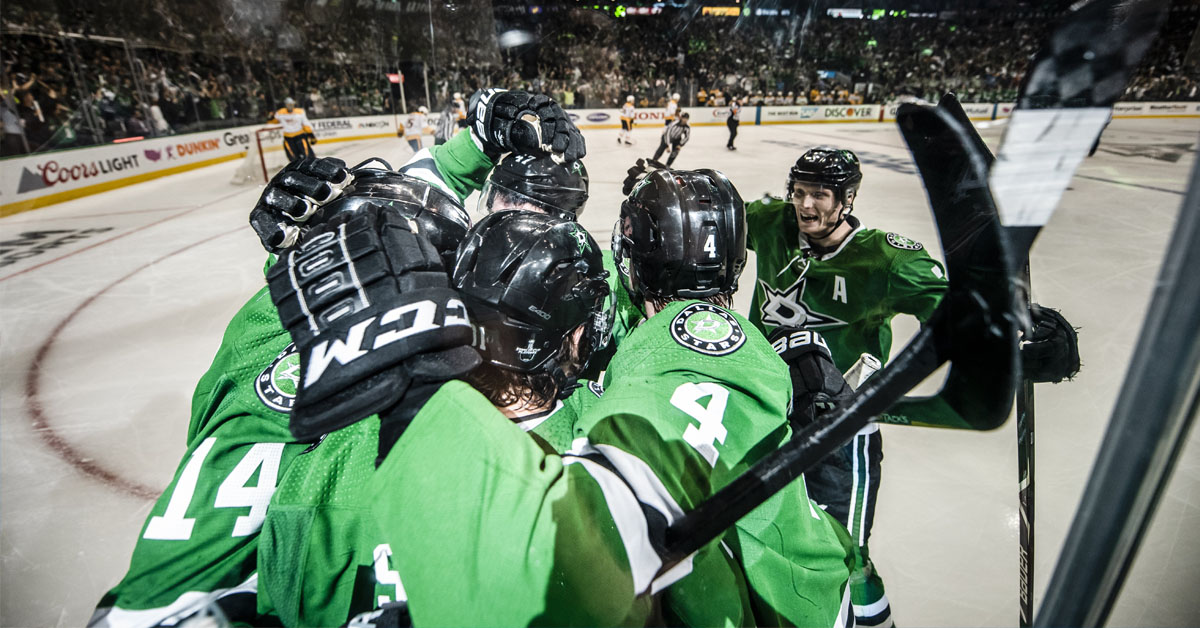
Dallas Stars Automate Social Reporting, Saving Time and Improving Partnerships
Overview Like many professional sports teams, the Dallas Stars needed a way to manage social content, track metrics, and publish sponsorship recaps for multiple partners. With the NHL
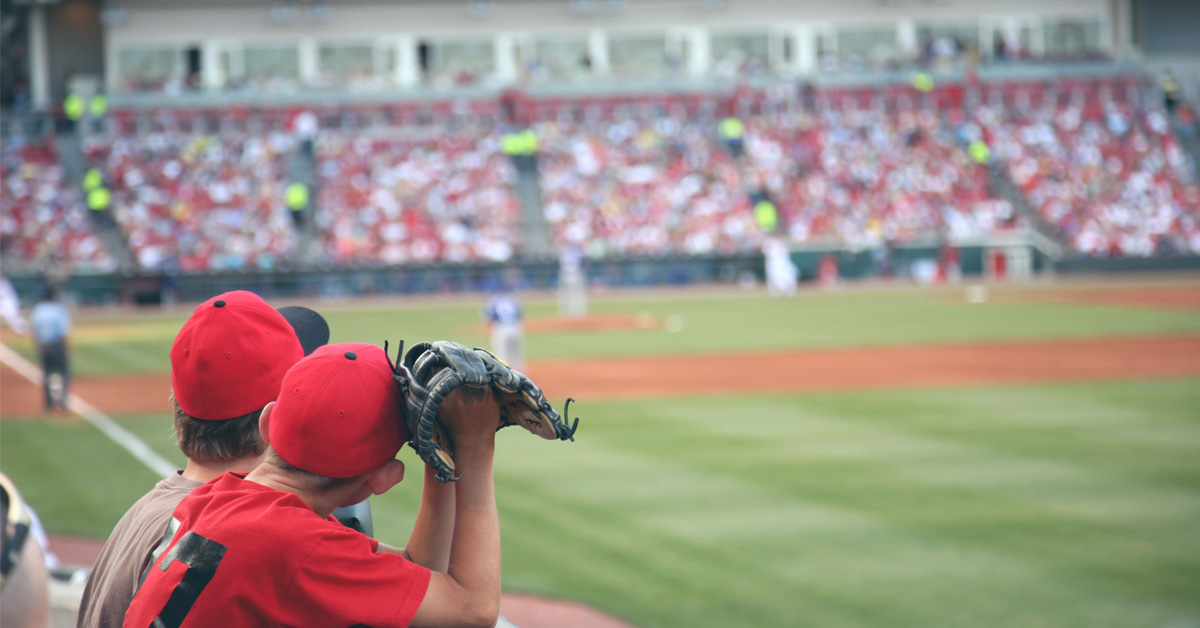
Key Takeaways: Generating Partnership ROI in a Post-Pandemic World
Last week, our panel at SportTechie’s State of the Industry shared how they are generating partnership ROI with flexible asset mixes, real-time measurement, and reporting.
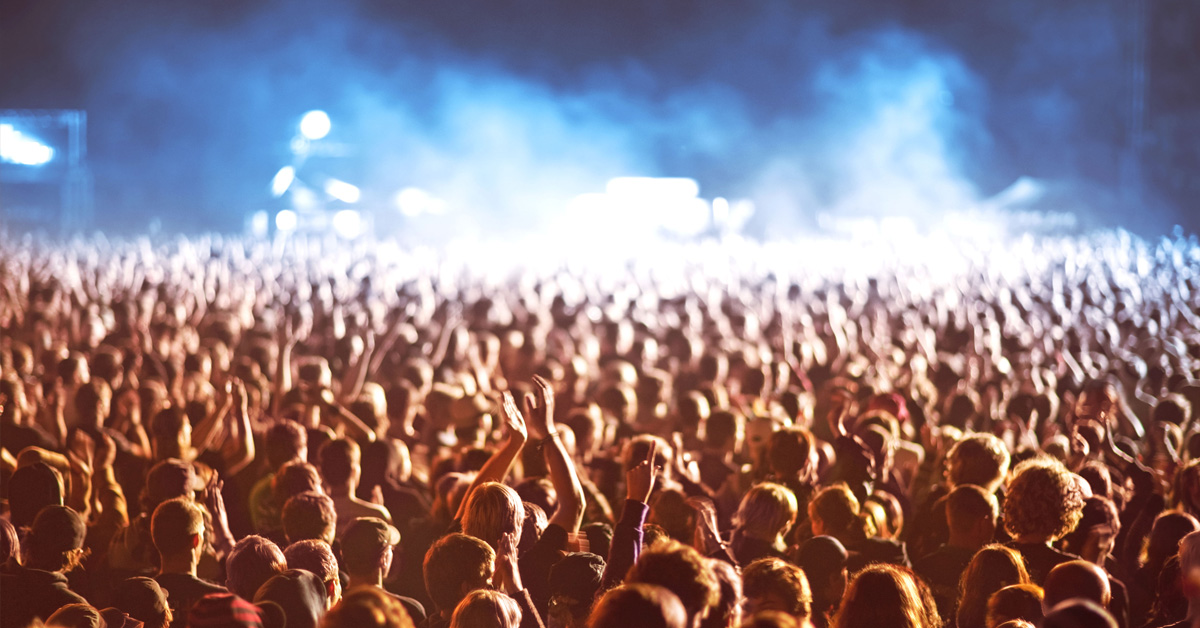
How to Modernize Your Sponsorship Strategy
At KORE, our software helps brands take a modern approach to their sponsorship strategy. KORE customers leverage these solutions to garner insights and streamline processes.
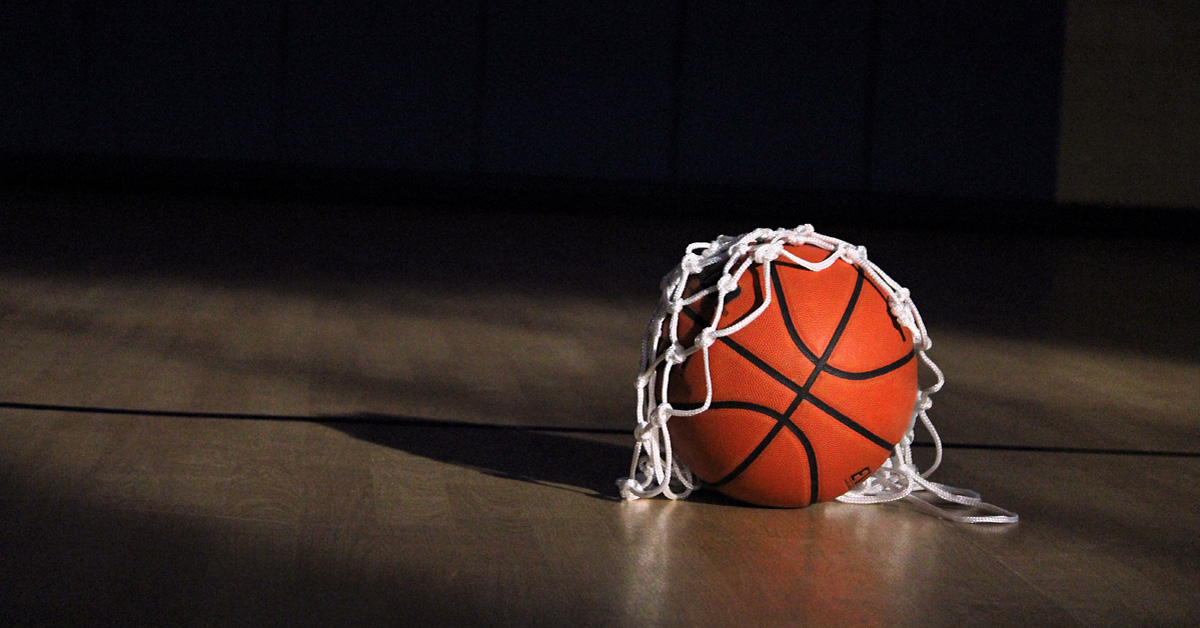
Visualizing Sponsorship Assets Across Collegiate Programs
Enter the third week of March, best known for trading an hour of sleep for some extra daylight, St. Patrick’s Day, and—of course—college basketball. If
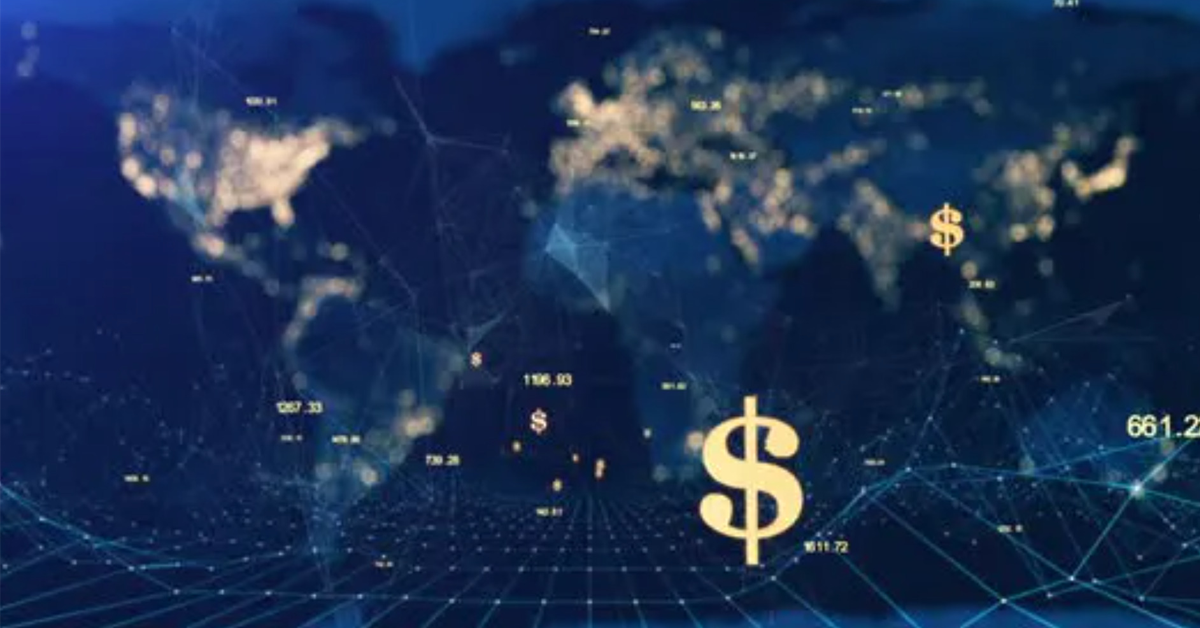
Cashing in on the Sponsorship Potential of NFTs
Memorabilia Is Going Digital on the Blockchain NFTs—non-fungible tokens—provide a way to create digital scarcity and verifiable ownership. Fans can buy and sell digital collectibles
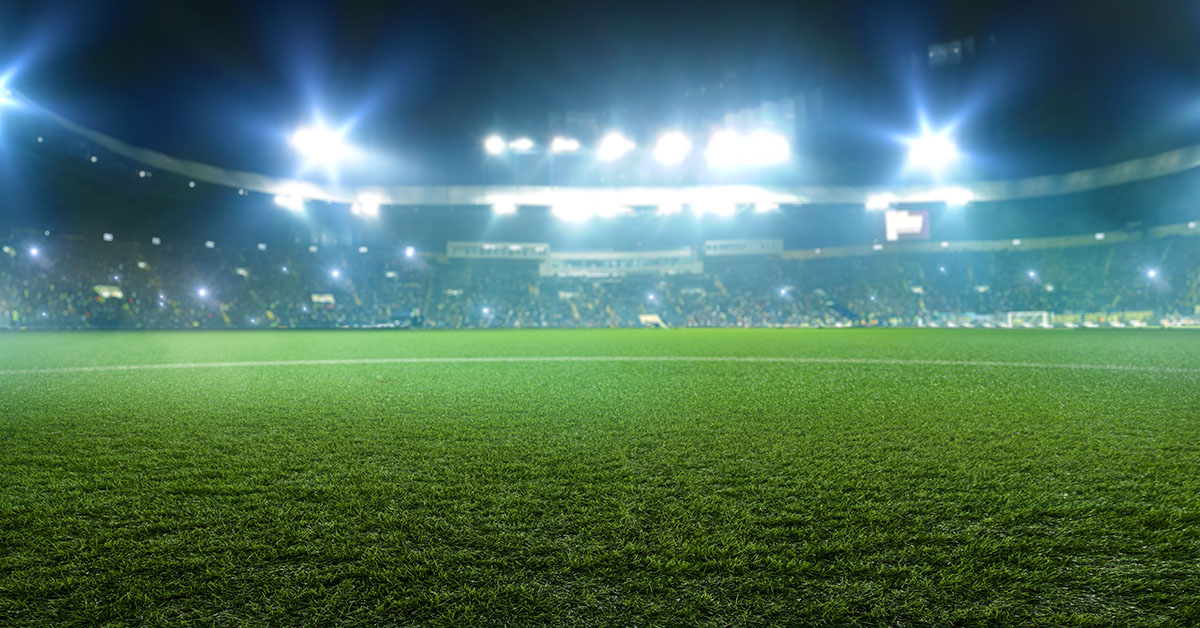
The Shift in Sponsorship Assets
As a new year begins, major changes are happening within sponsorship deals and the teams who manage them. One of the most in-demand skills is
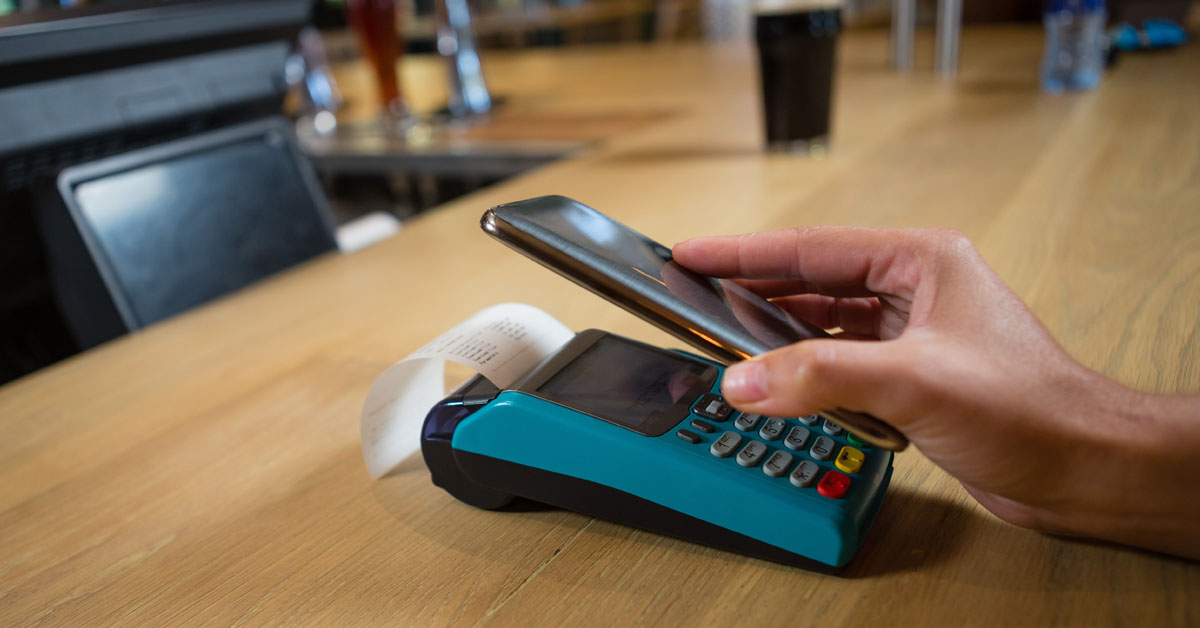
Show Me the (Digital) Money!
The NFL’s Big Game is always a leader in combining entertainment and technology. This weekend we will all experience a more virtual and futuristic feel,
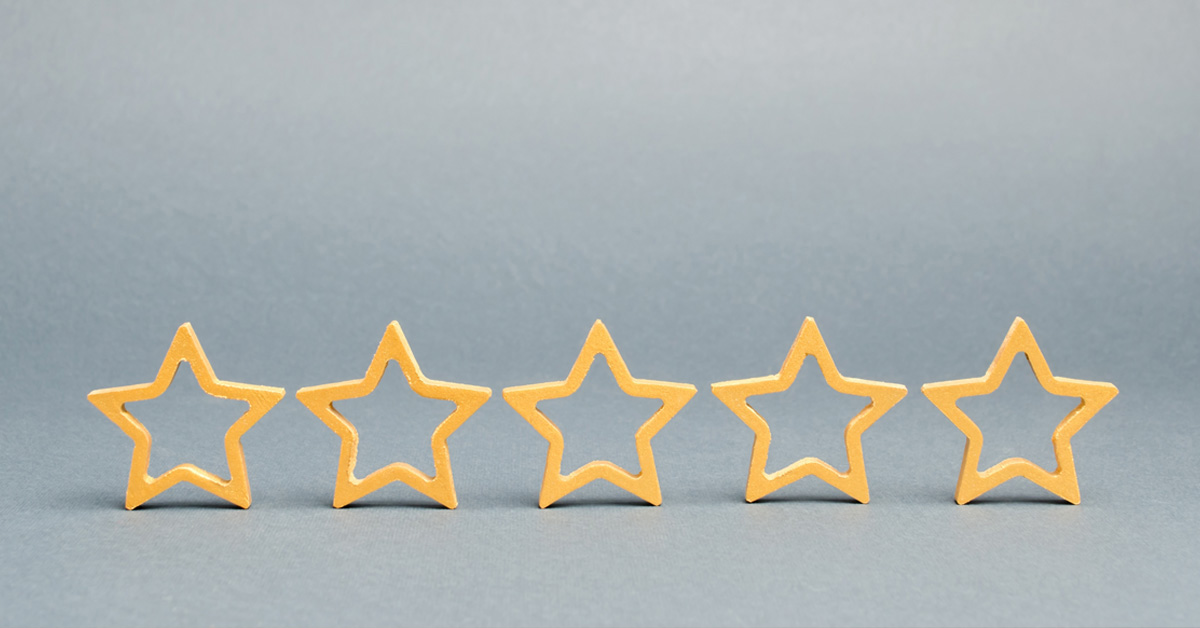
Brand Sponsors, Take Full Advantage of Your Hospitality Perks
Across North America’s “Big 5” sports, 76% of sponsorship deals include some type of hospitality at events. It’s one of the greatest assets that teams
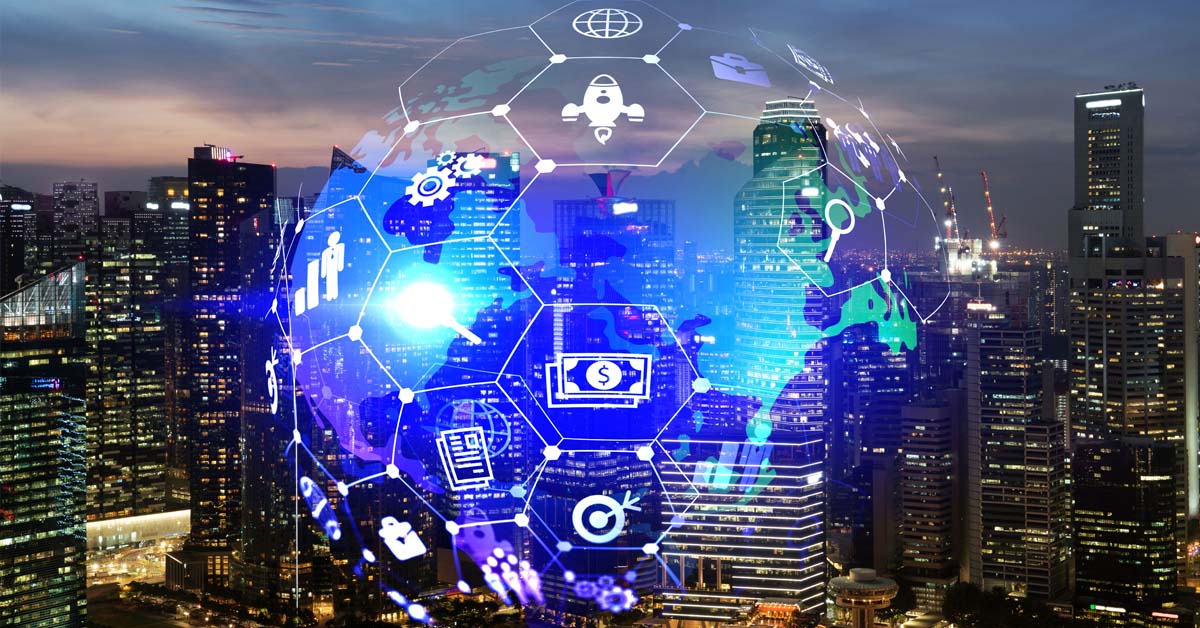
Key Takeaways: Unlocking a Data-Rich Landscape
Like many events, the Sports Tech World Series conference in Australia went virtual this year. KORE’s Cliff Unger (SVP of Worldwide Sales) and Marc Roots
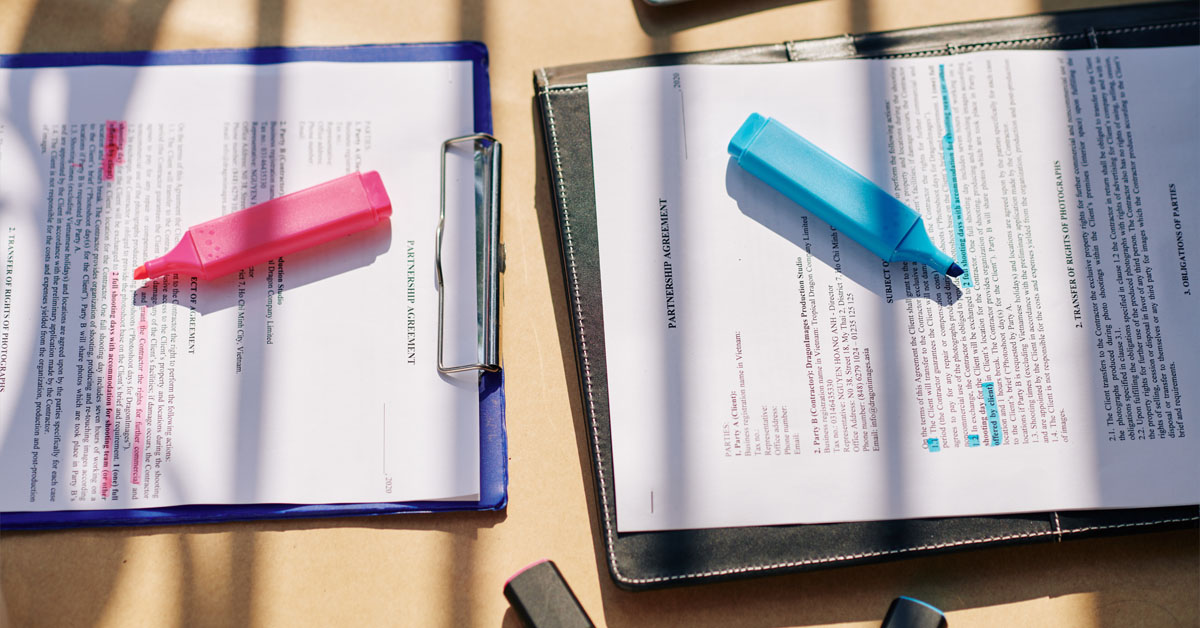
What Type of Report Will Have the Most Impact on Sponsorship Strategy in 2021?
Every strategist has a go-to way of evaluating the success of a deal – Both short term and long term. So, I wanted to unpack
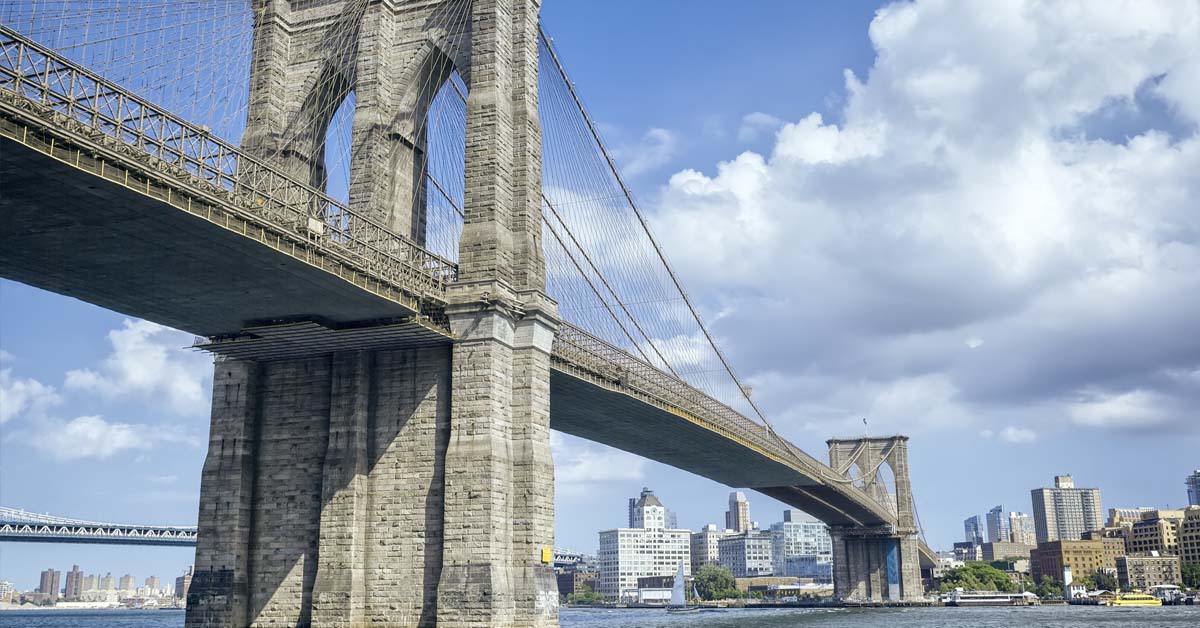
How the Brooklyn Nets Are Turning Sponsorships Into Partnerships
The Brooklyn Nets recently announced one of the NBA’s most unique sponsorship deals. Starting in the 2020-2021 season, Motorola will bring their iconic “batwing” logo
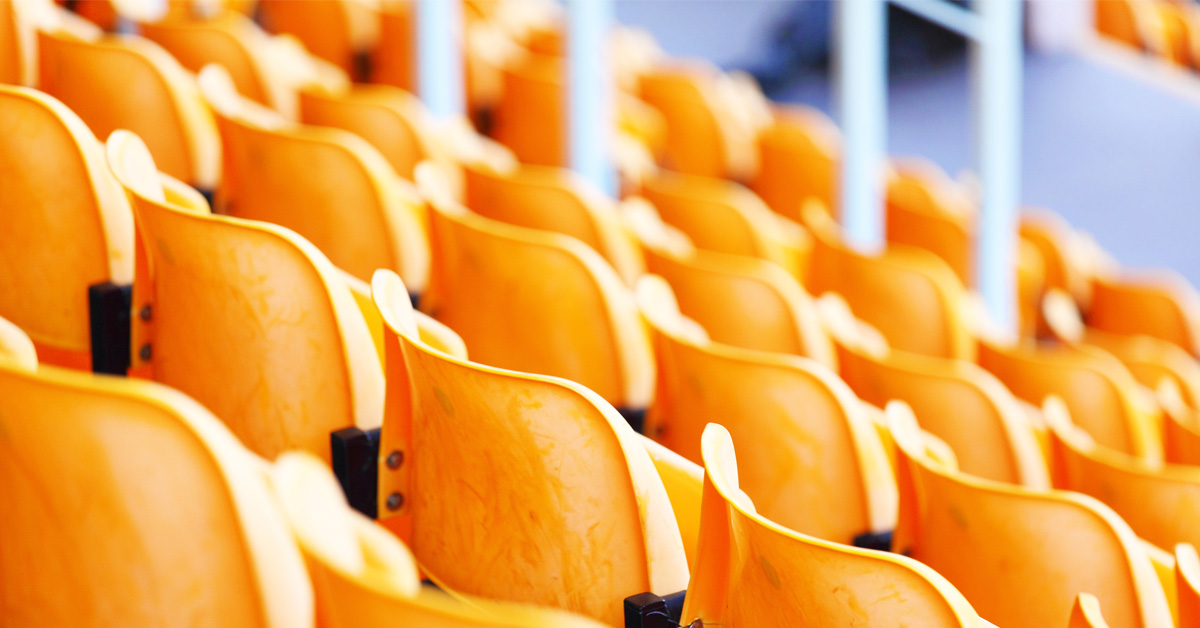
Pick a Seat, but Not Any Seat – Tips for Limited Capacity Ticketing
It’s exciting that a few different COVID-19 vaccines are nearing approval, but it will take time to distribute them and gradually build herd immunity. Many
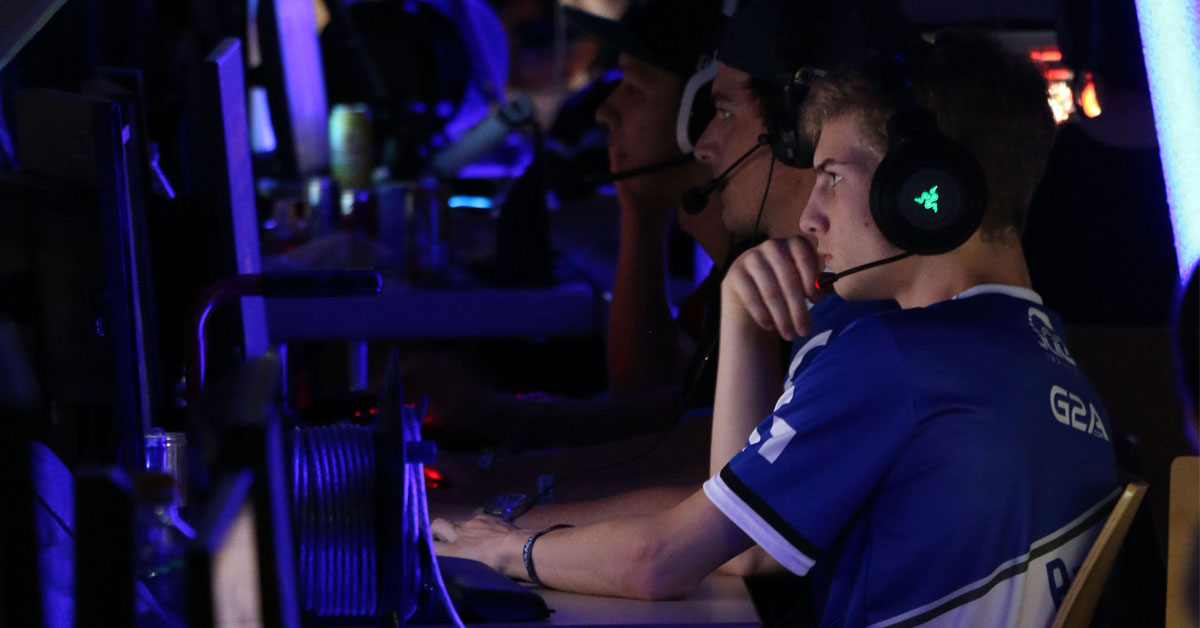
6 Reasons Brands Are Attracted To Sponsoring Esports
As more people—especially youth and young adults— shift their habits away from traditional media, it’s becoming harder for brands to reach these fragmented audiences. By
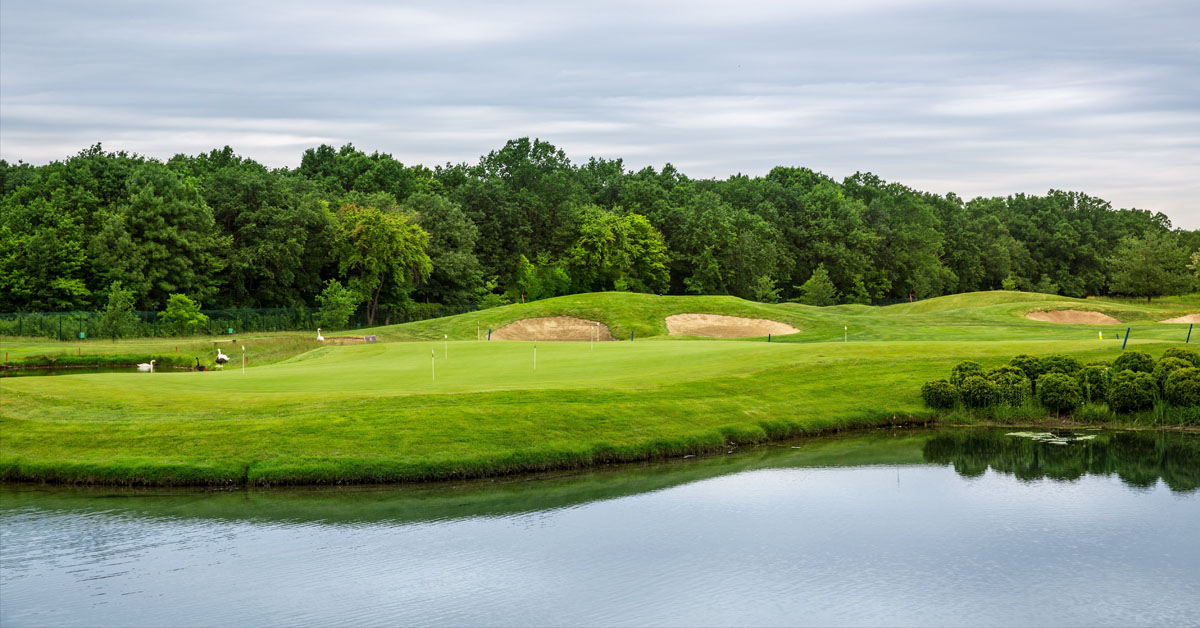
A Master Balance: Tradition x Technology
After a seven-month delay, and zero egg salad sandwiches consumed by fans this year, we can finally crown our 2020 Masters Champion, Dustin Johnson. Scoring
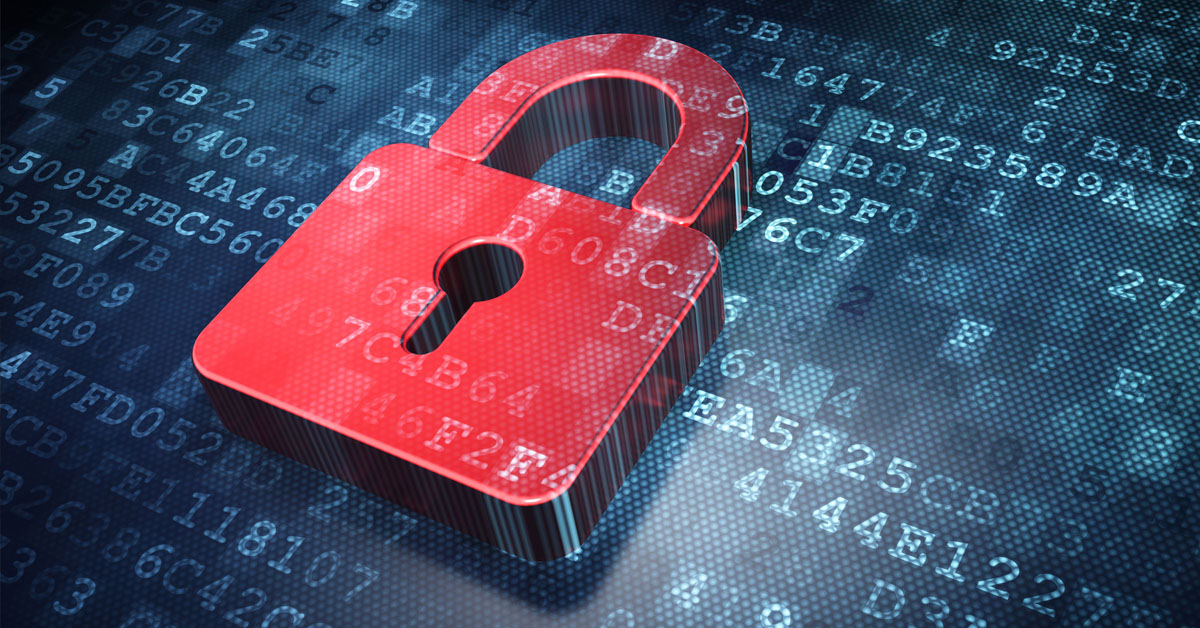
Unlocking Sponsorship Data And Beginning To Use It More
Heads up, I’ve stolen some key themes from KORE’s whitepaper, Data Warehousing and Analytics, that is largely geared towards rights holders. With so much planning and
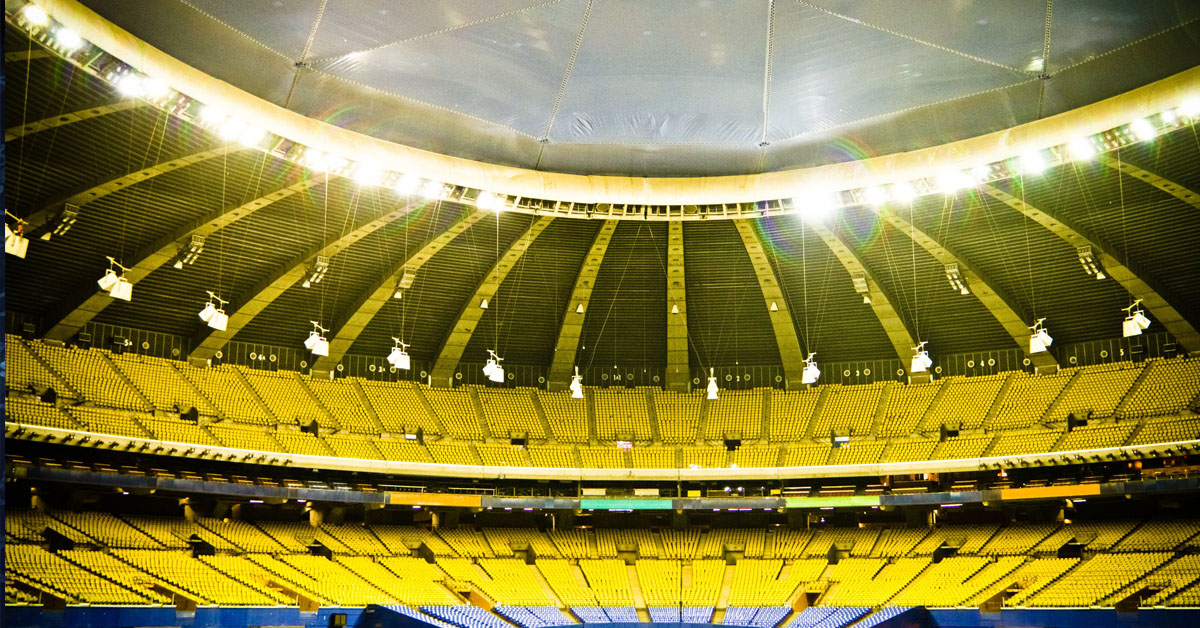
2020 World Series Preview
Welcome to the 2020 World Series. Hopefully, you read that in your best (or worst) Joe Buck impression. After an improbable season, we have finally
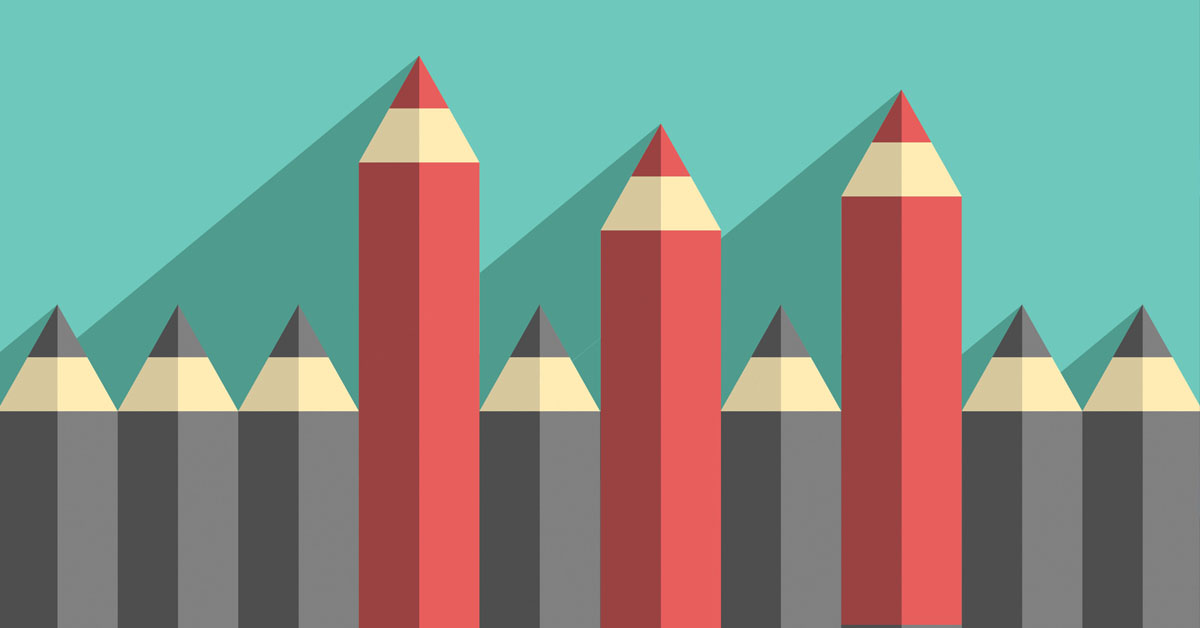
3 Things That Will Provide Both Short and Long-term Benefits to Sponsorship Managers
We have had some fairly significant shifts in the way we manage sponsorship, operationally speaking. We have had to answer progress and performance-related questions like
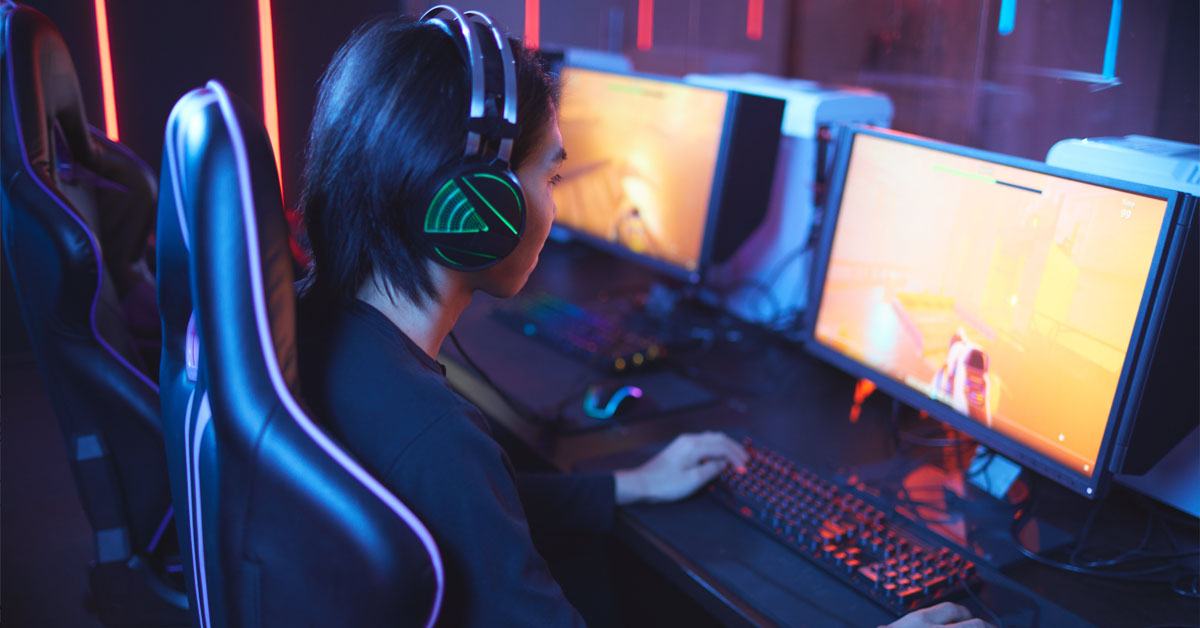
Esports Athletes: The Next College Superstars
Esports in higher education In 2017, 57.6 million unique viewers watched the professional esports League of Legends (LoL) championship match online. That number almost matches the viewership
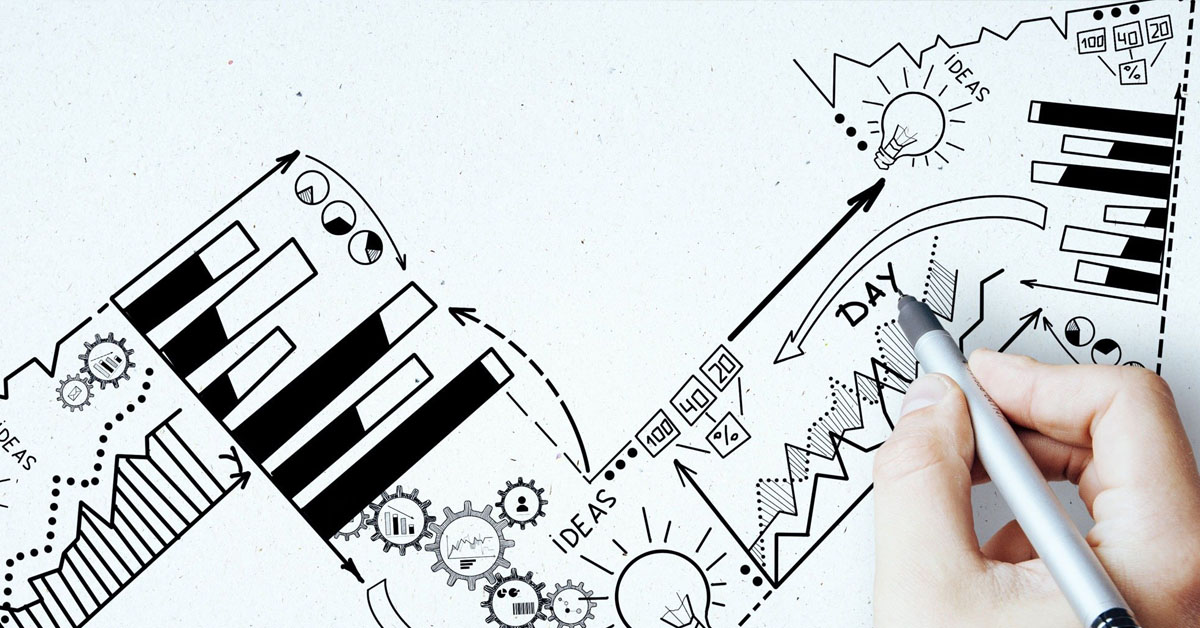
Hacking Sponsorship: Part Three – Perceived Value and Audience Segmentation
So far in our Hacking Sponsorship series, we have unpacked, in part one, Strategy and Headcount, and in part two, Asset Management and Creative, and
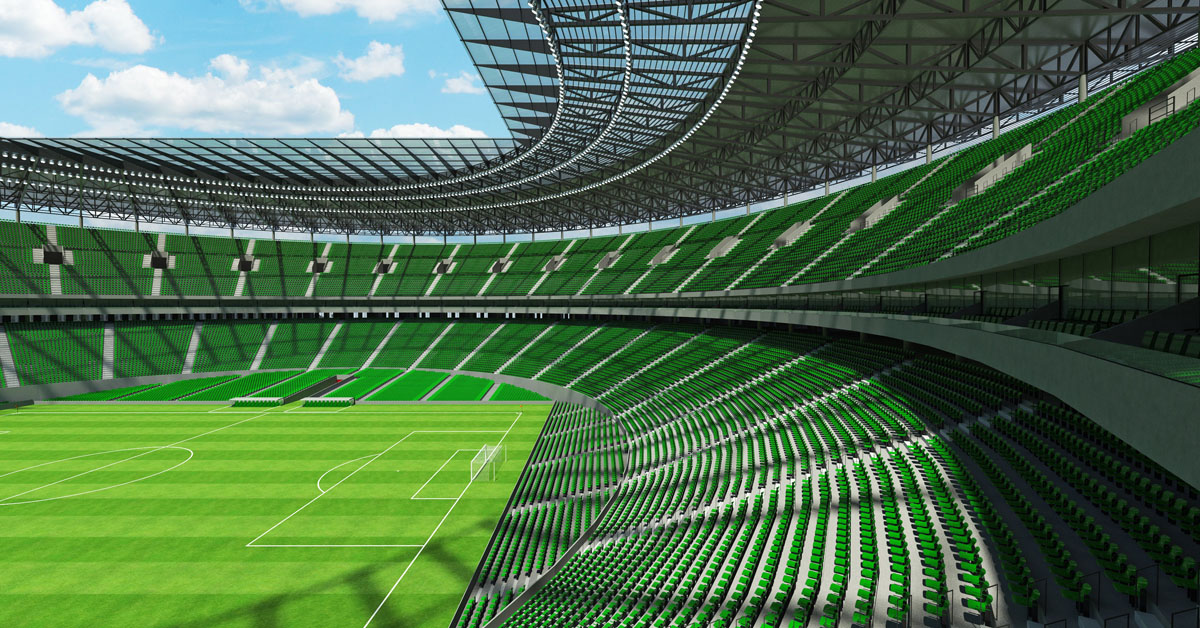
Opening Back Up: Dealing with Post-Pandemic F&B Variability
After coming to a screeching halt back in March, the sports and live events industry is slowly coming back to life; Hard Rock Stadium and
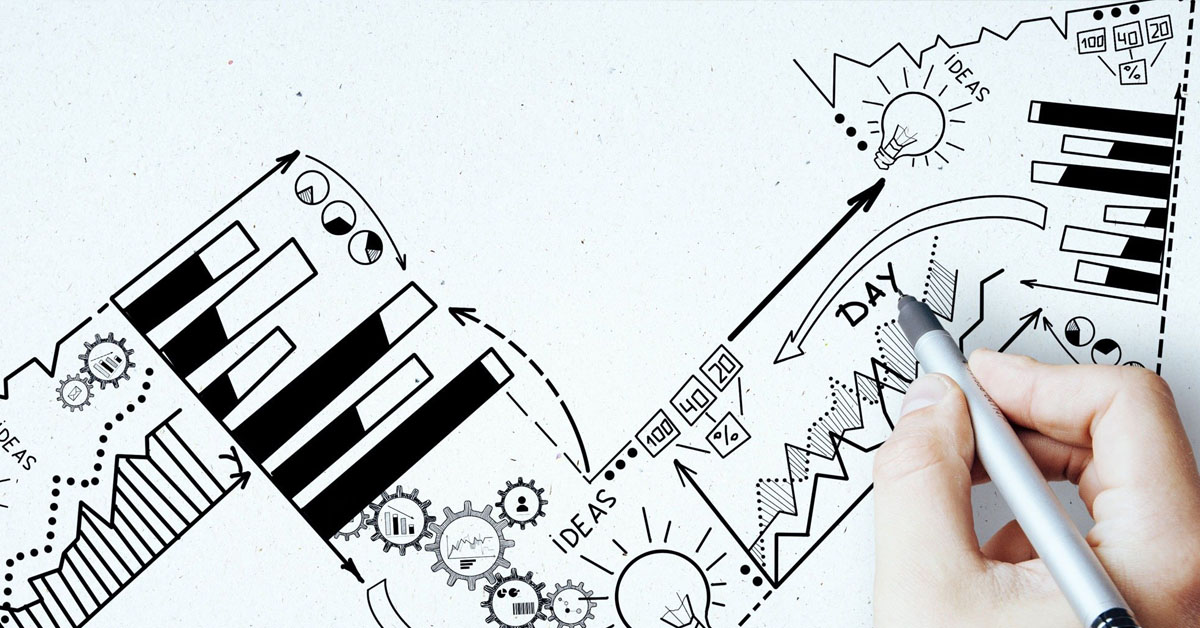
Hacking Sponsorship: Part Two – After the Chaos
Picking up where we left off with Part One – Planning for the Return, I wanted to continue taking a look at what some of
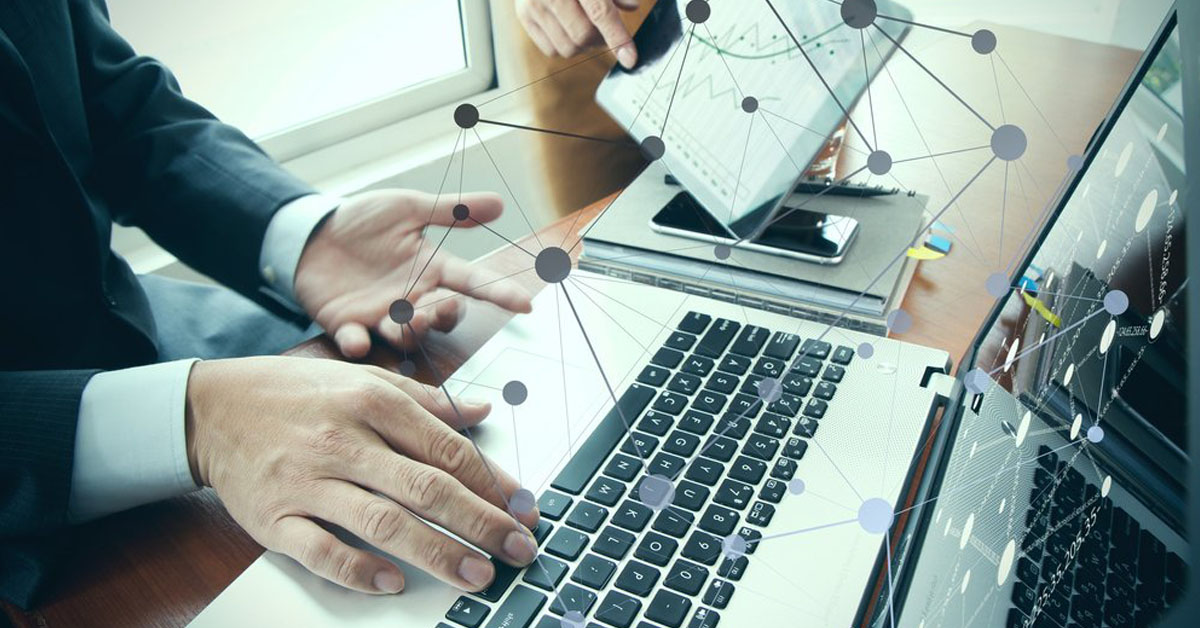
Managing Changes to Corporate Partnership Data
Did you know that data loss costs small businesses about $75 billion per year in downtime? We most often think of data loss in the
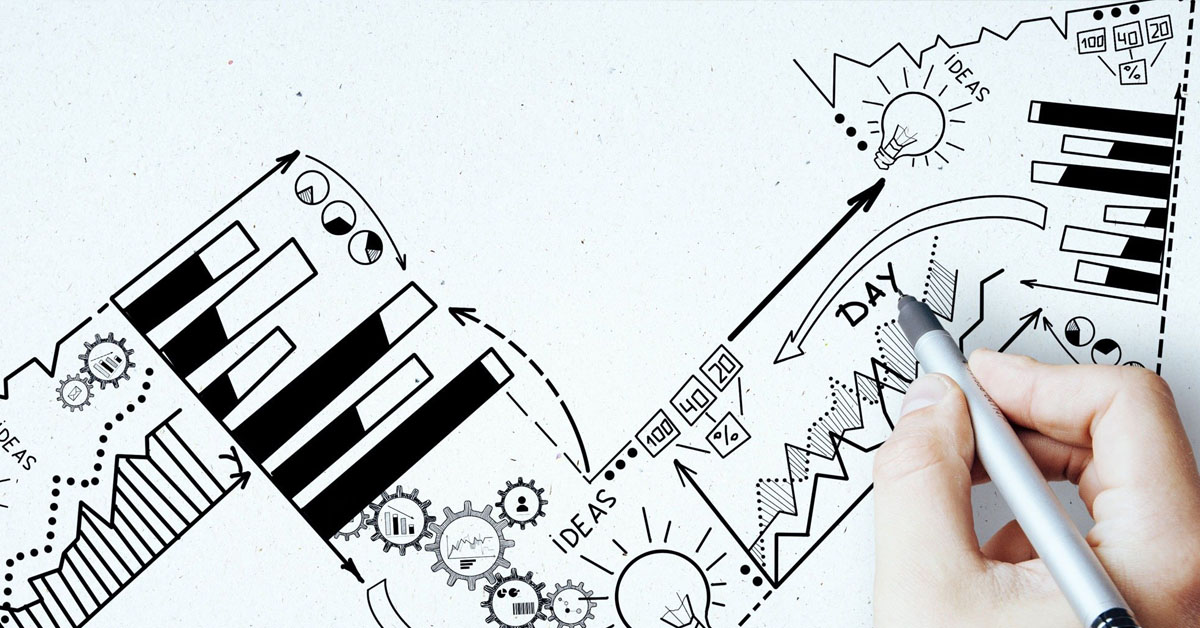
Hacking Sponsorship: Part One – Planning for the Return
We briefly touched on it in the last episode of the Inside Sponsorship podcast, however, there is going to come a point whereby strategy and
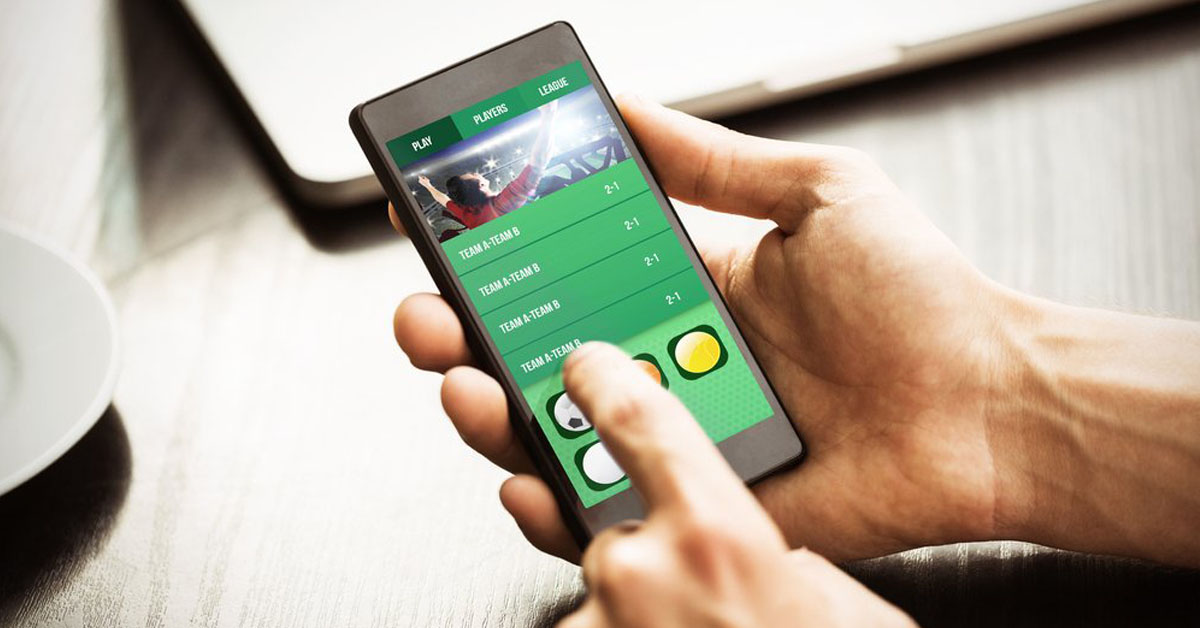
Betting on Sports’ Return
This past May, the NFL opened the opportunity for betting companies to sponsor and partner with football teams. Casino partners have been allowed since late
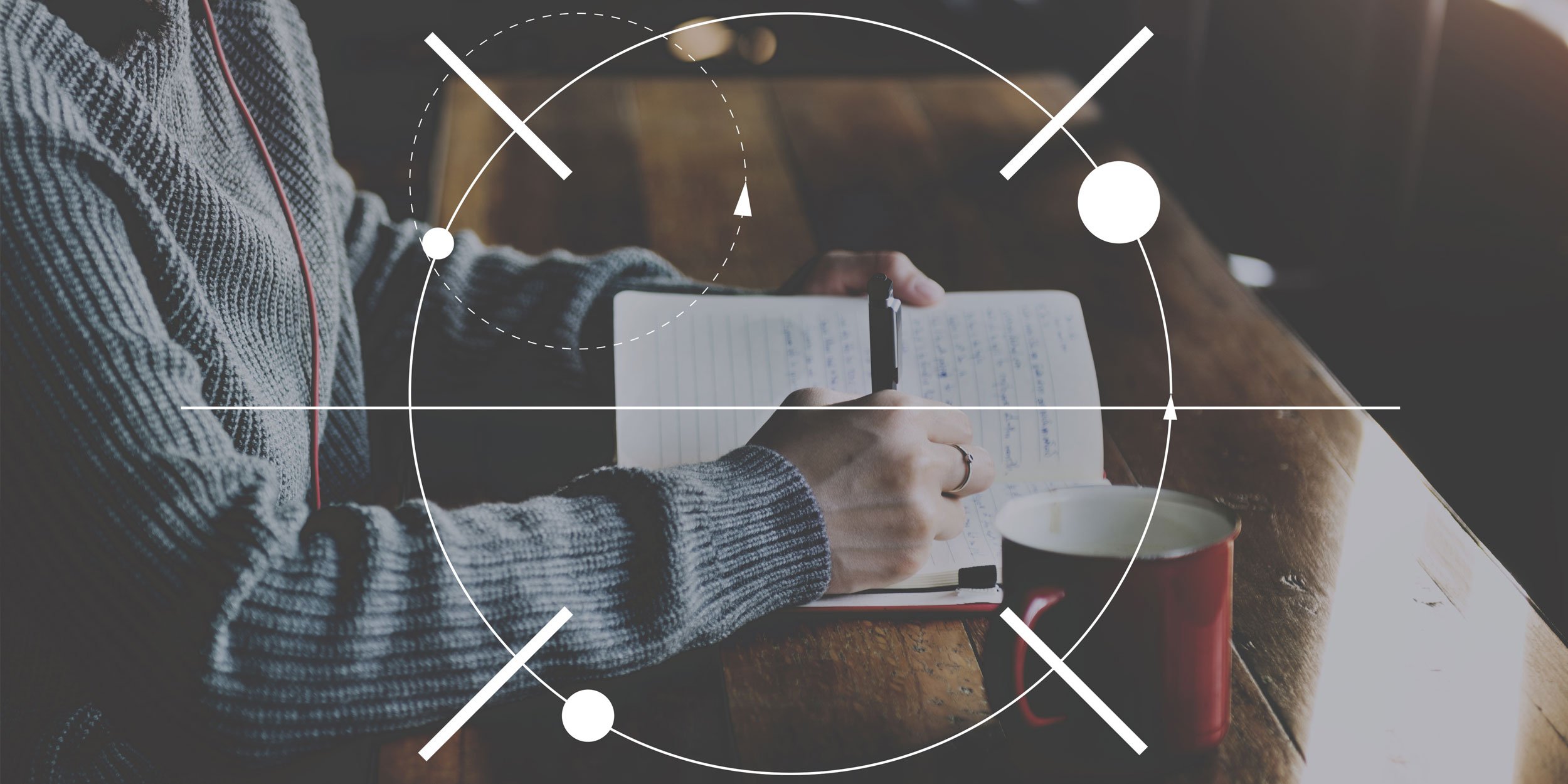
A Look At Current International Sponsorship Trends
As the world continues to come to grips with its new-normal, the sponsorship world is consequently experiencing side effects of new social norms, new engagement
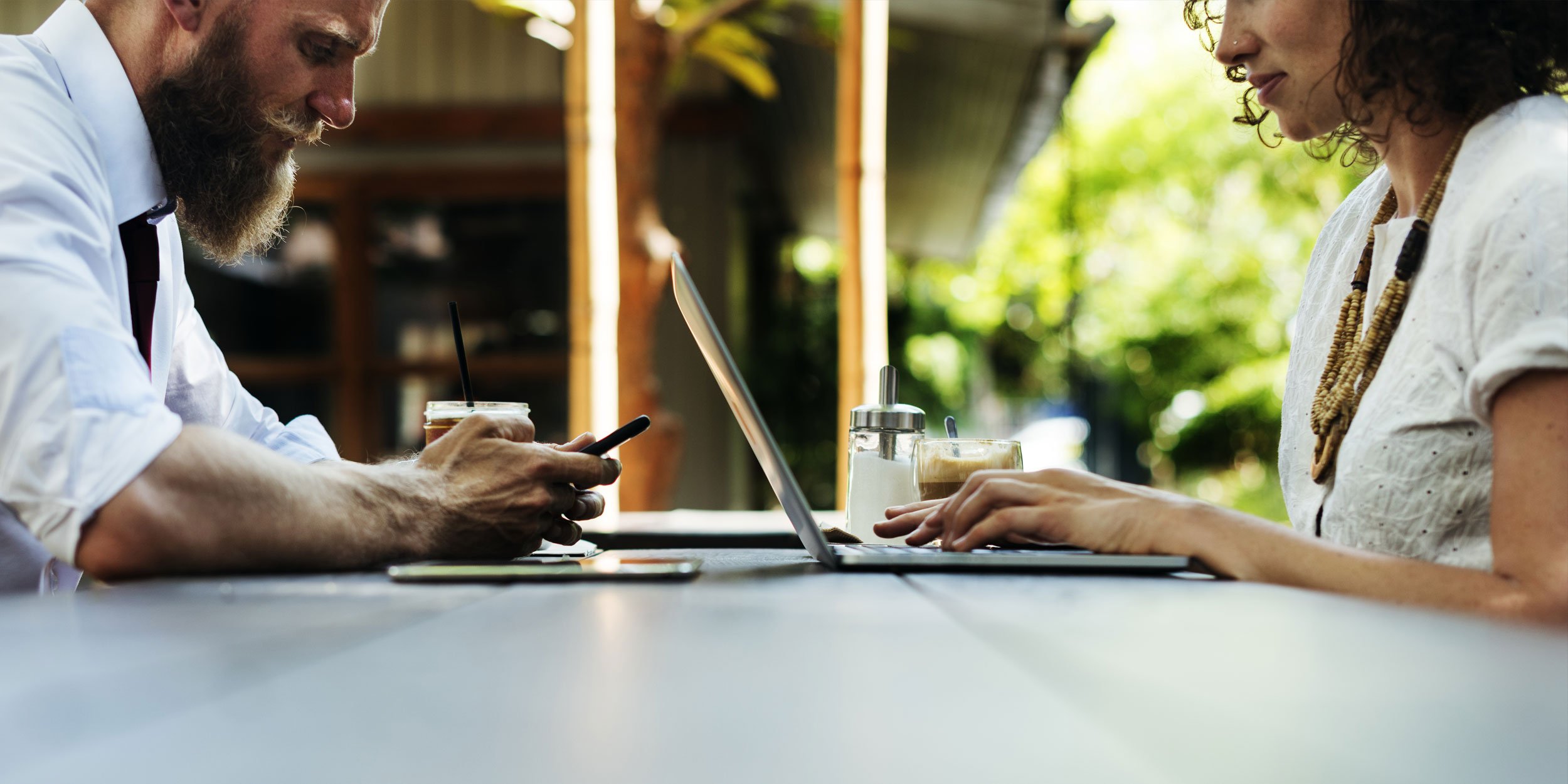
What to Consider When Returning to Sell Sponsorship
Over the past few days, we have started to see sponsorship deals happening again. Beşiktaş and Beko. Pringles and ESL. J-League and Meiji Yasuda Life
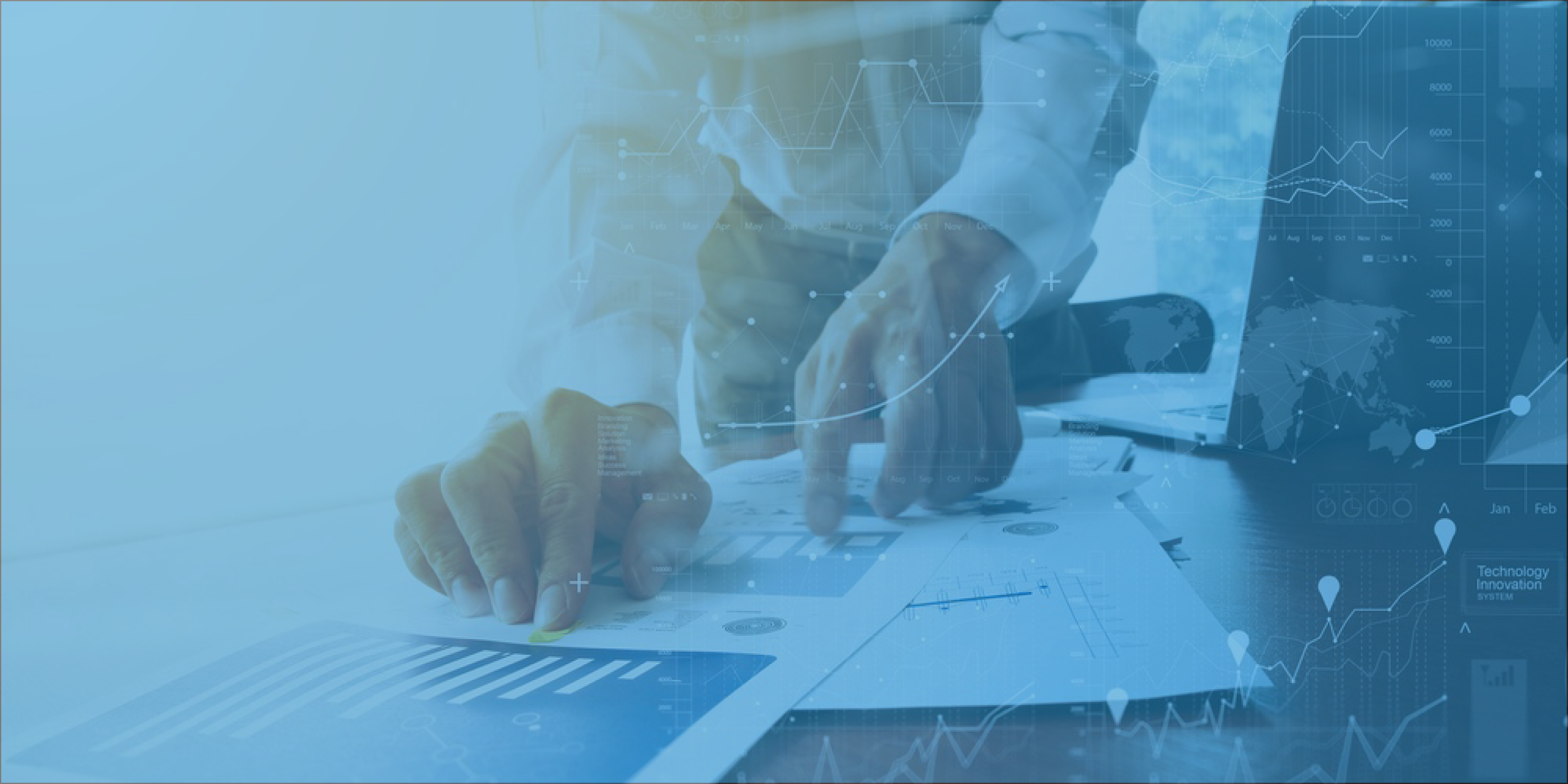
7 Tips to Optimize Your CRM Migration Project
With “regular business” in major flux due to the circumstances surrounding the COVID-19 pandemic, many organizations are using this time to tackle considerable projects previously
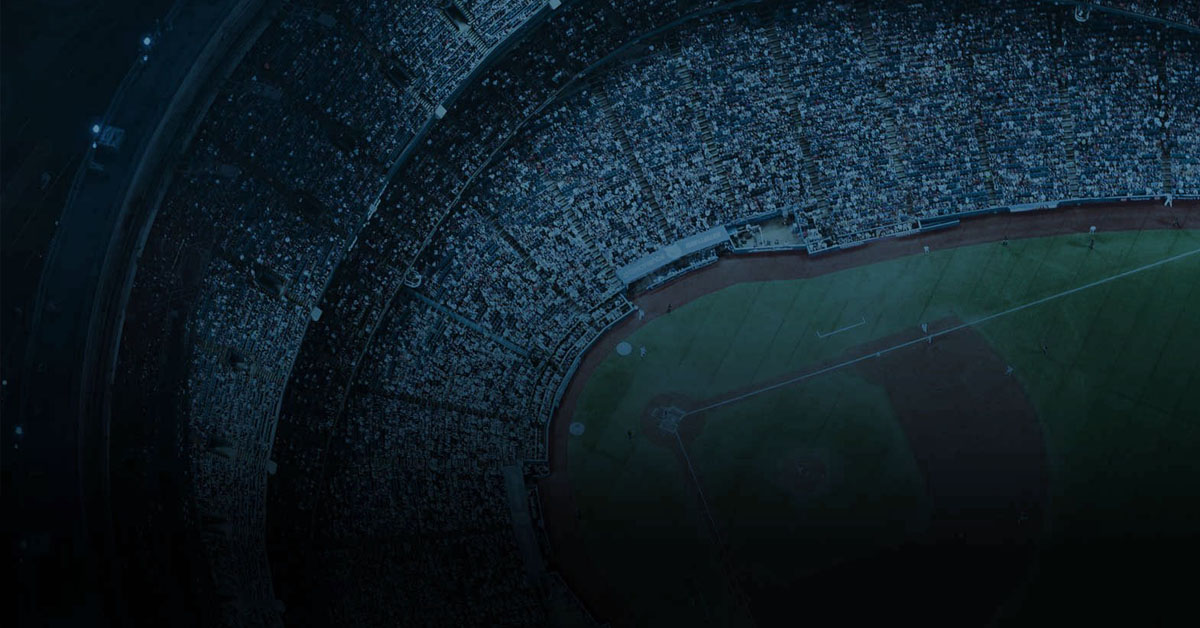
COVID-19: Sponsorship – What’s Now and What’s Next
COVID-19. I don’t need to say much more for us to get a sense of what’s happening in the sponsorship and broader marketing worlds.
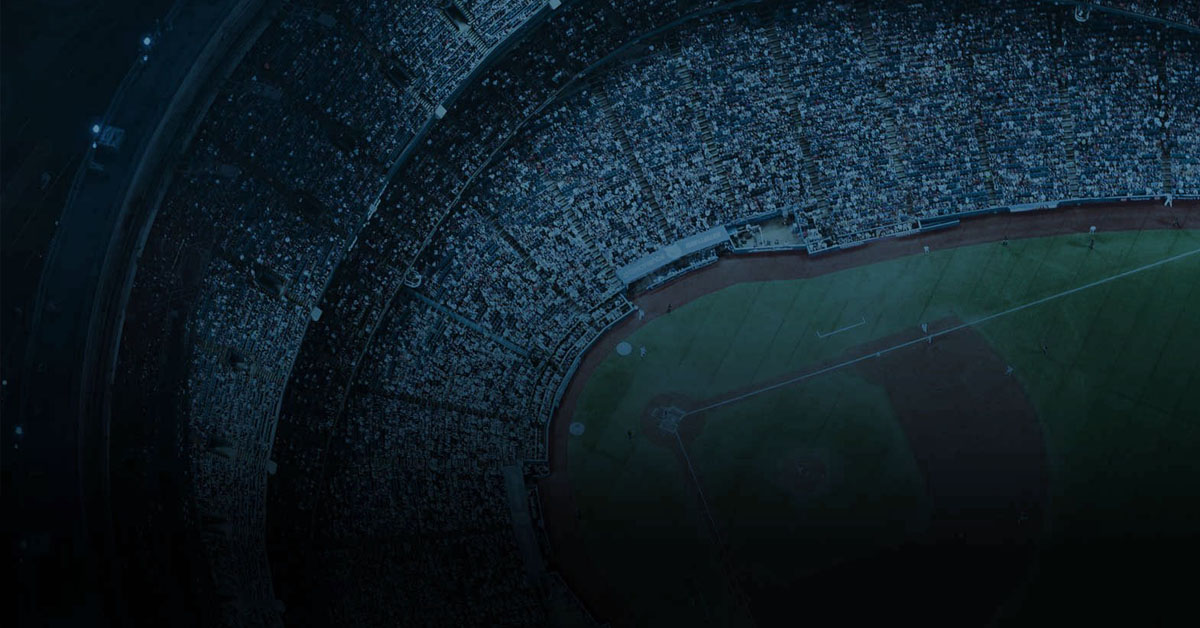
COVID-19: Service & Retention Ticket Requests
A key area we are keeping track of, both through our product usage and through our conversations with clients, is how the market is responding
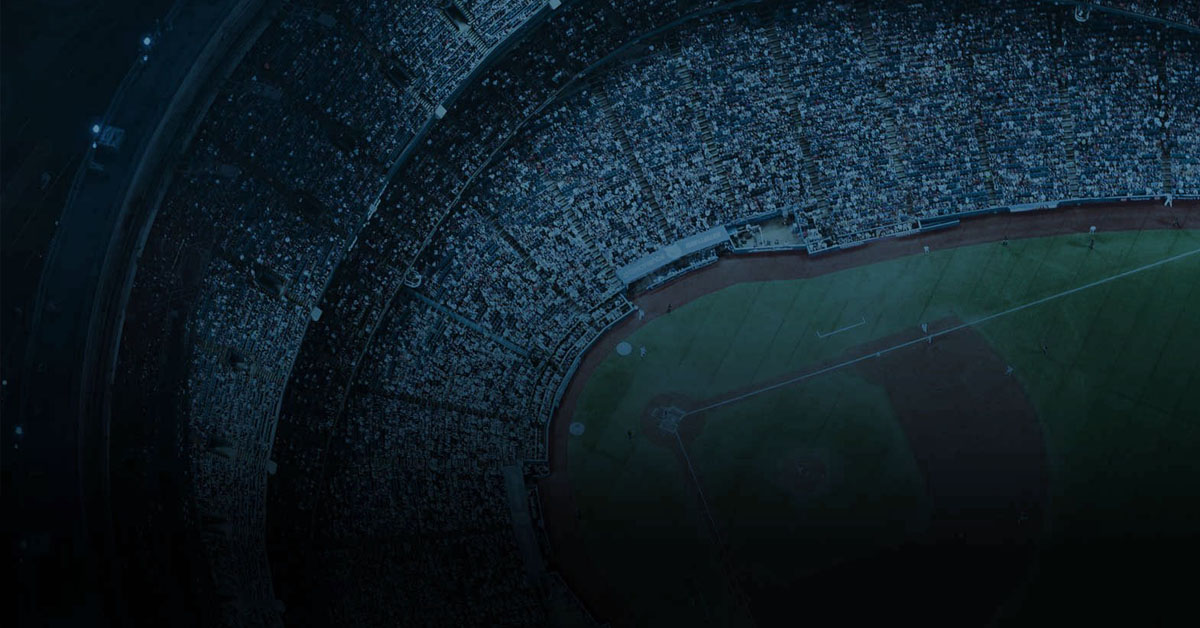
COVID-19: Sponsor Response, Insight, and Planning
With live events on pause, rights holders across the world are searching for answers on how to proceed in these unprecedented times: How can they
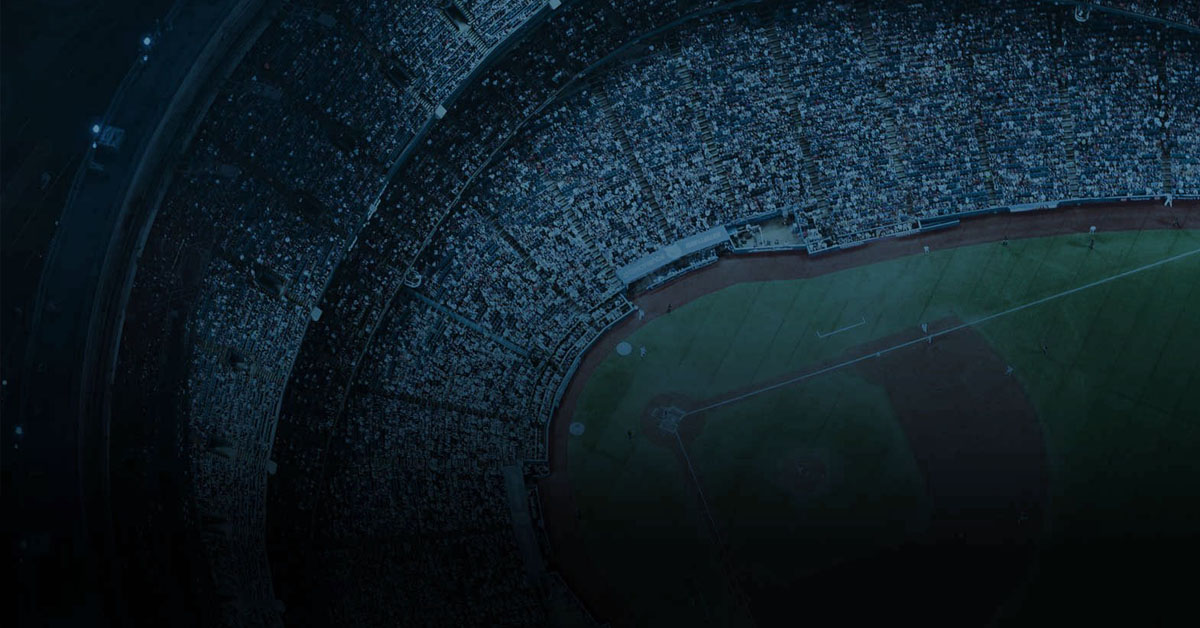
COVID-19: A Note From KORE Software
We hope you and yours are healthy, safe and settling into this new rhythm. Like most of you, the KORE team has been working from
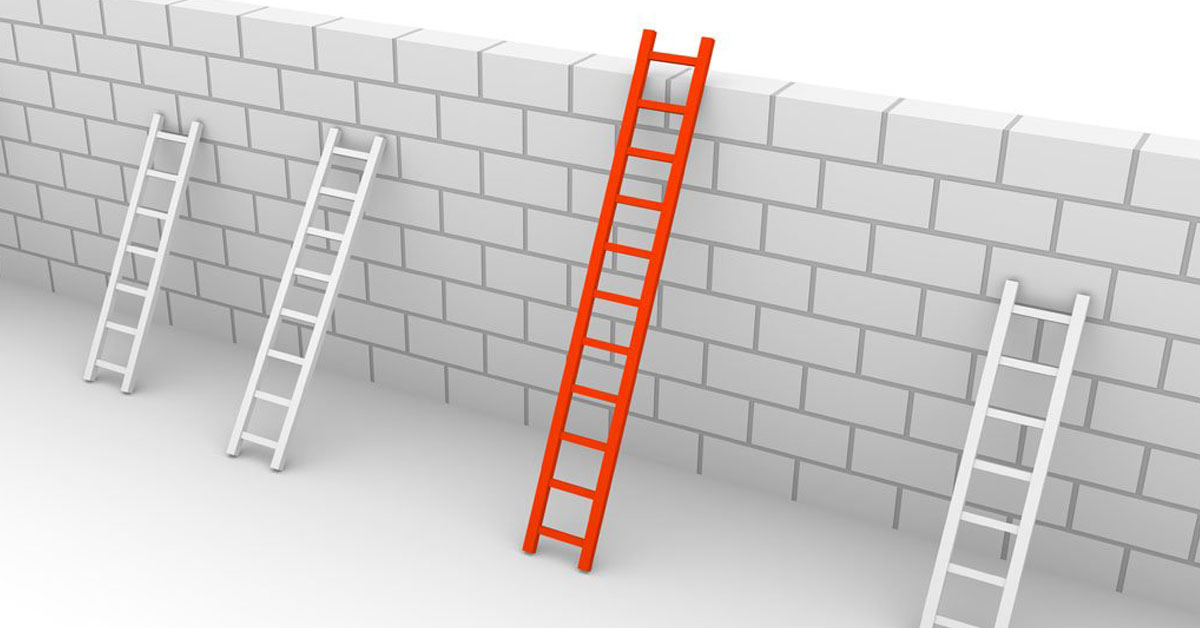
Governing Body vs Pro-Team – Which Has A Greater Commercial Advantage?
I have been lucky enough to work in the sporting commercial space with a huge variety of rights holders, ranging from volunteer clubs to governing
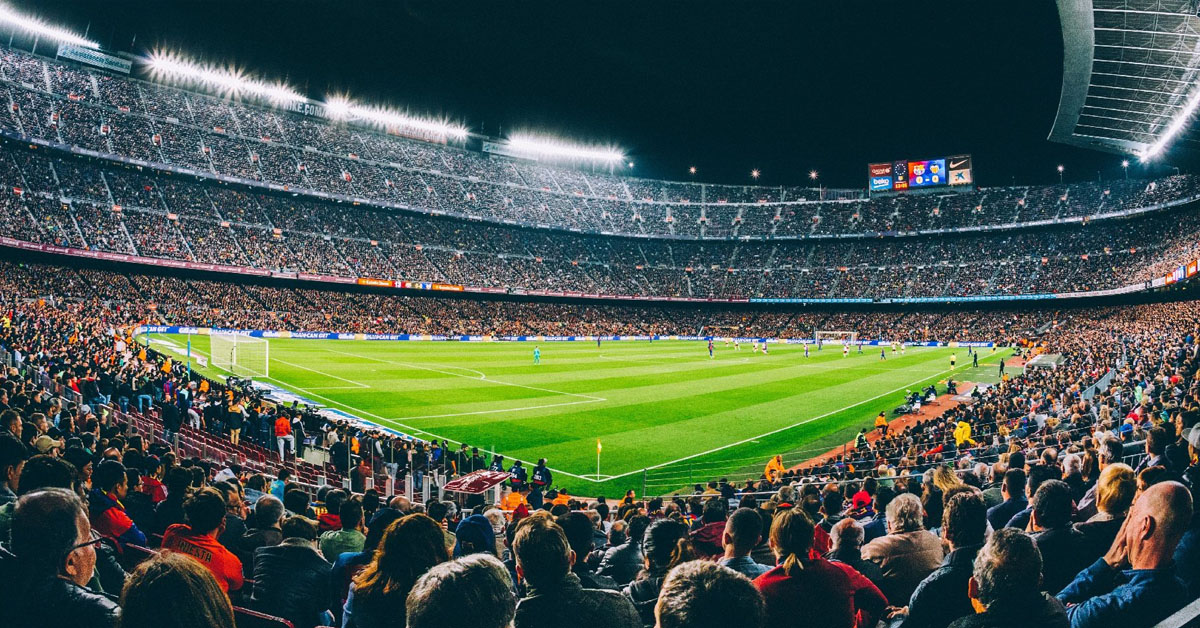
Jumpstart Your Ticket Plan Renewal Modeling
It’s that time of year for many teams and entertainment organizations to lock in their ticket plan renewals. The use of retention modeling is a
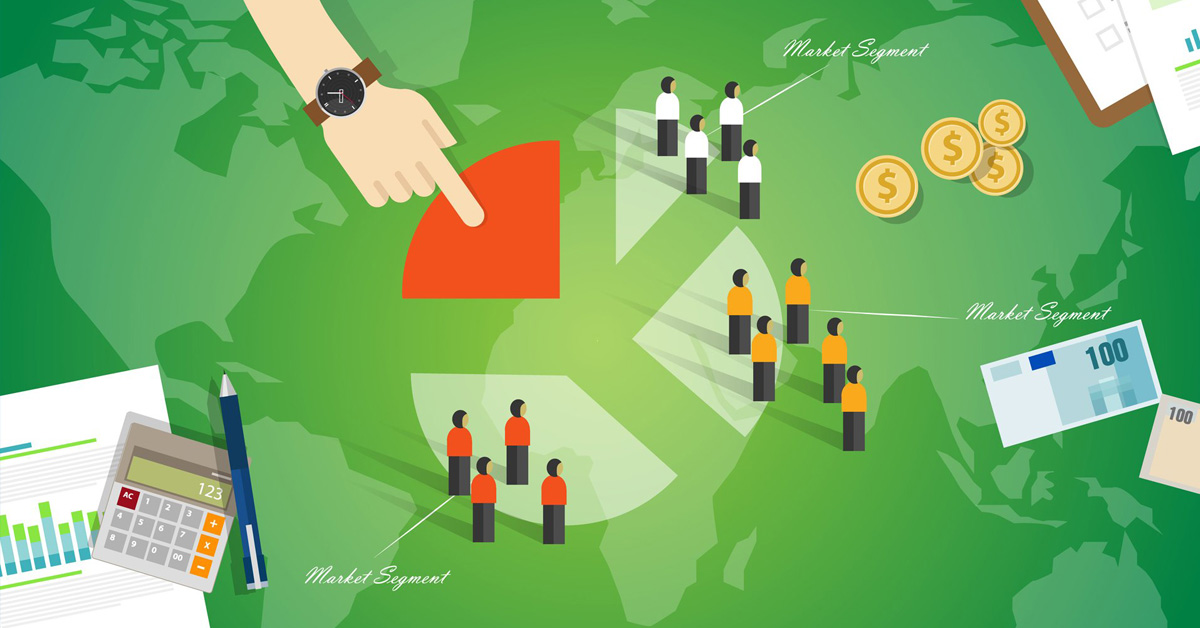
Quantity vs Quality – The Fan Segmentation Debate in Sponsorship
Last year, on Inside Sponsorship, we spoke with Ben Hartman, Chief Client Officer at Octagon, about award-winning trends in sponsorship. Although this largely looked at
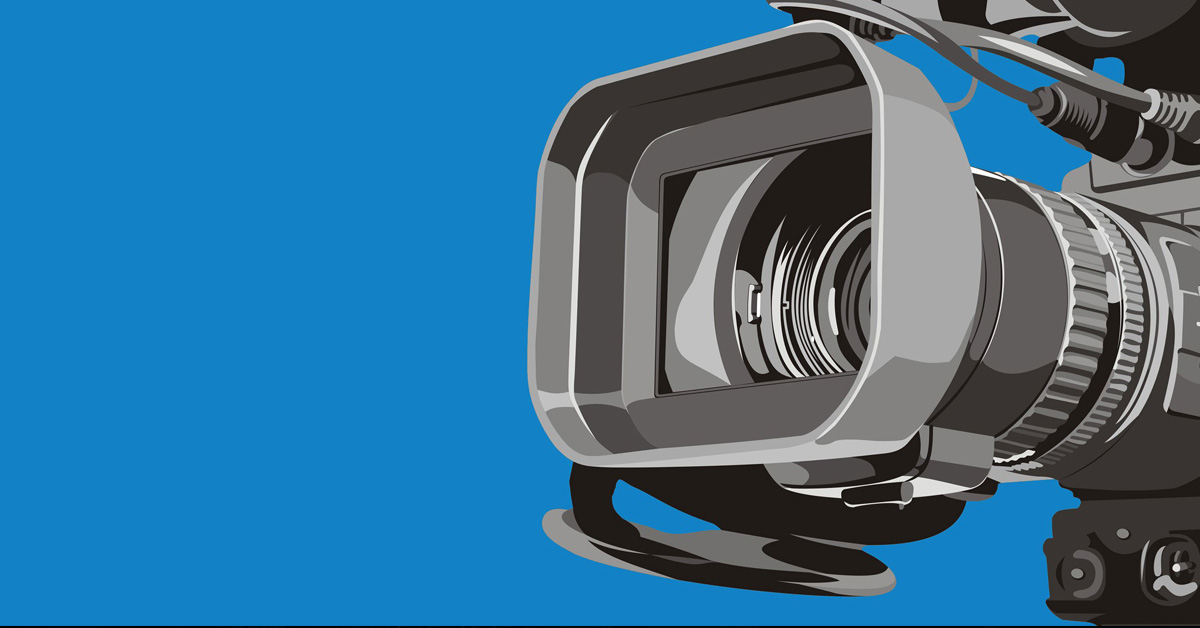
The Changing Face Of Broadcast And What This Means For Broadcast Partnerships
All of the sudden, in 2019, we had more ways than ever before to consume our sports and entertainment content. Just three to five years
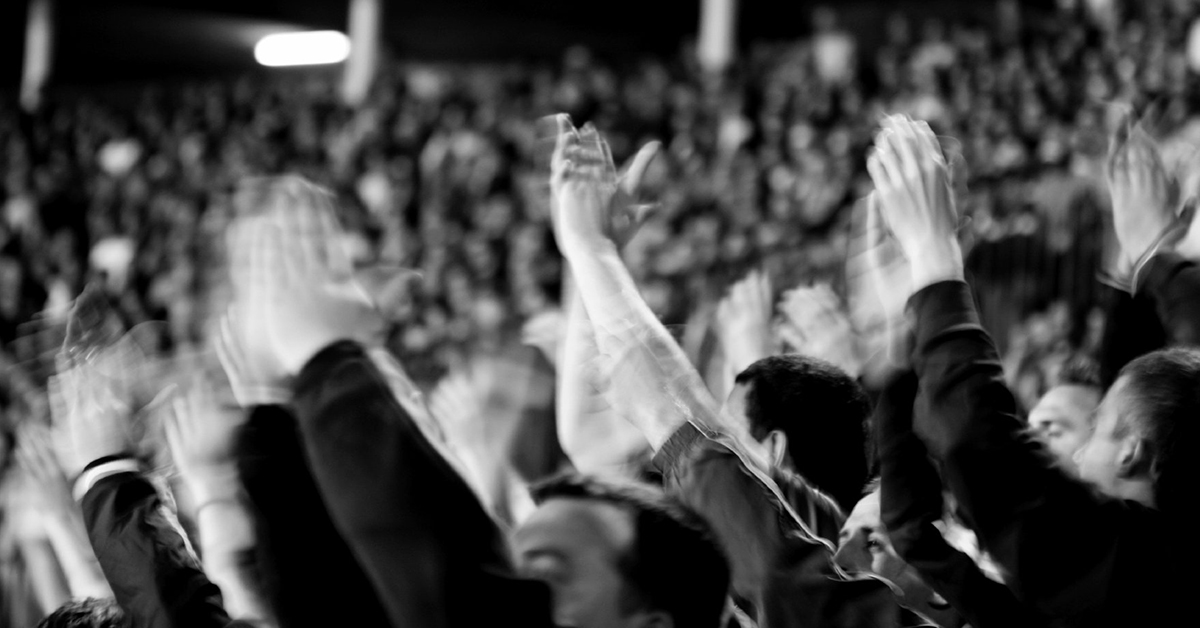
Trending in Intercollegiate Athletics: Takeaways from the SBJ Intercollegiate Athletics Forum 2019
Leading up to the annual NCAA awards ceremonies and ensuing college football bowl games, Sports Business Journal hosted their annual Intercollegiate Athletics Forum (IAF) in
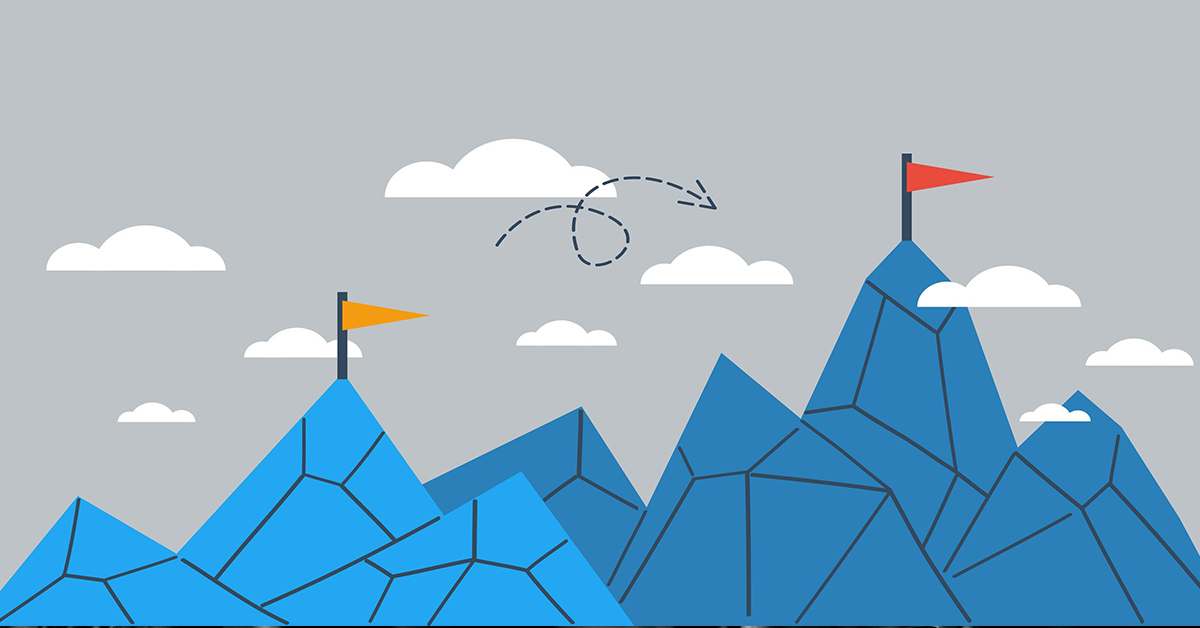
Expanding Competition Geographies and How Sponsorship Needs to be Approached
As a life-long Rugby fan, I watched on eagerly this year as Pro14 Rugby (who are also a great client of us here at KORE)
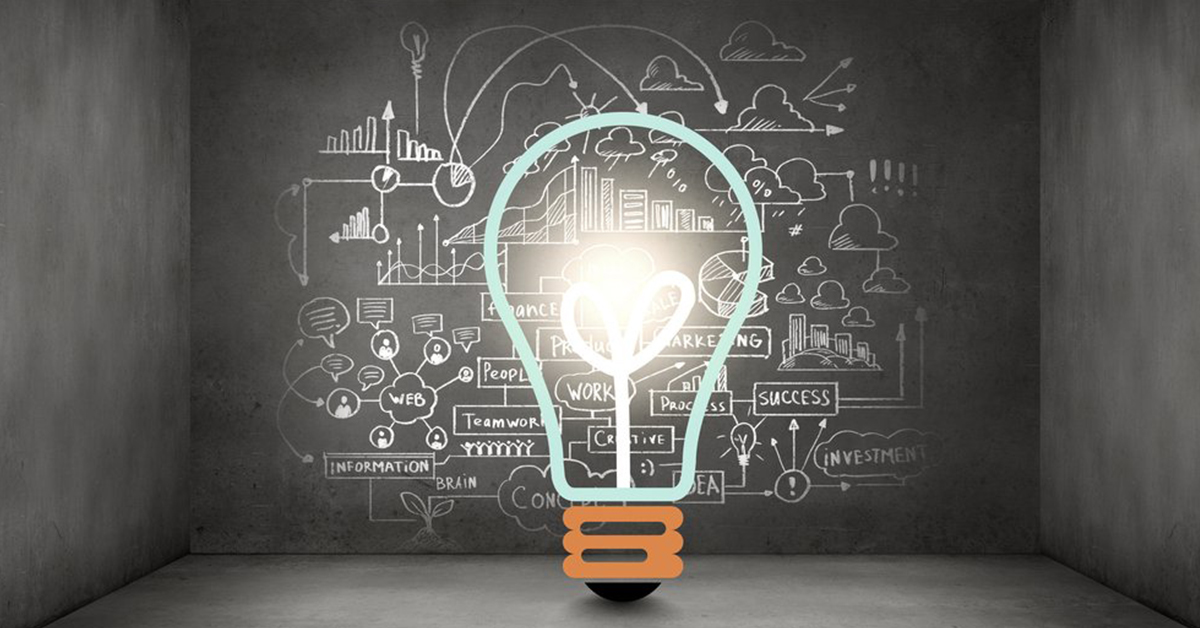
Three Takeaways from Sports Business Journal’s Sports Marketing Symposium 2019
Right from the opening remarks on understanding Gen Z content consumption, this year’s Sports Business Journal’s Sports Marketing Symposium provided some excellent insight into some
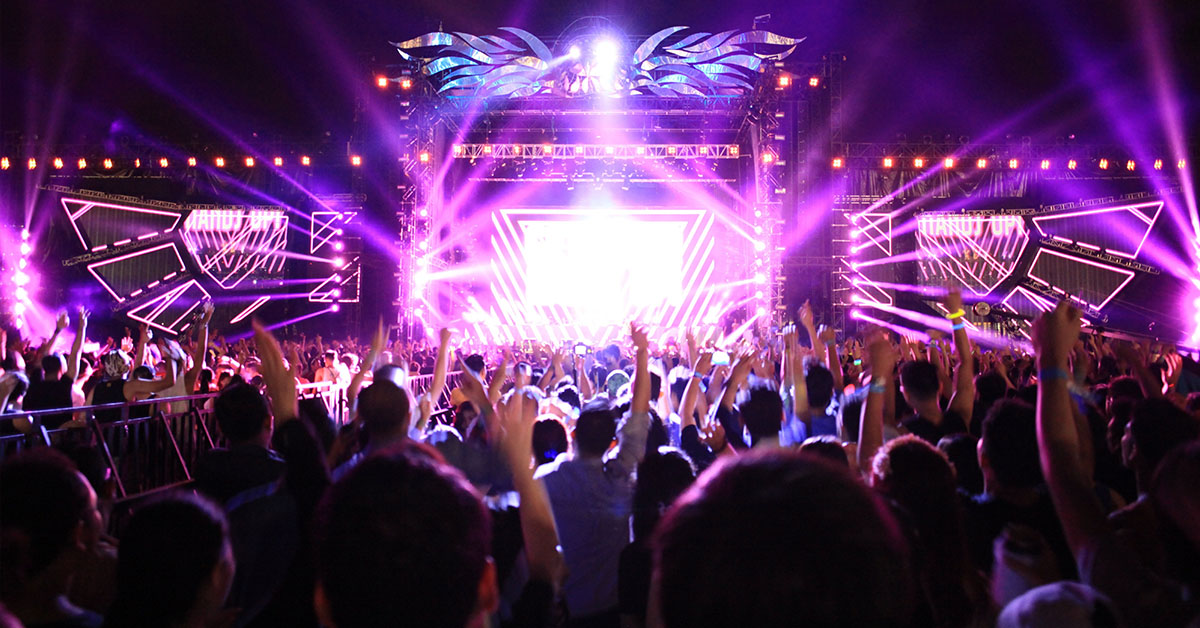
Stubhub, Viagogo, and an Interactive Look at Live Event Experiences
Yesterday it was announced that eBay has agreed to sell online ticketing platform StubHub to European rival, Viagogo, for about $4 billion in an all-cash
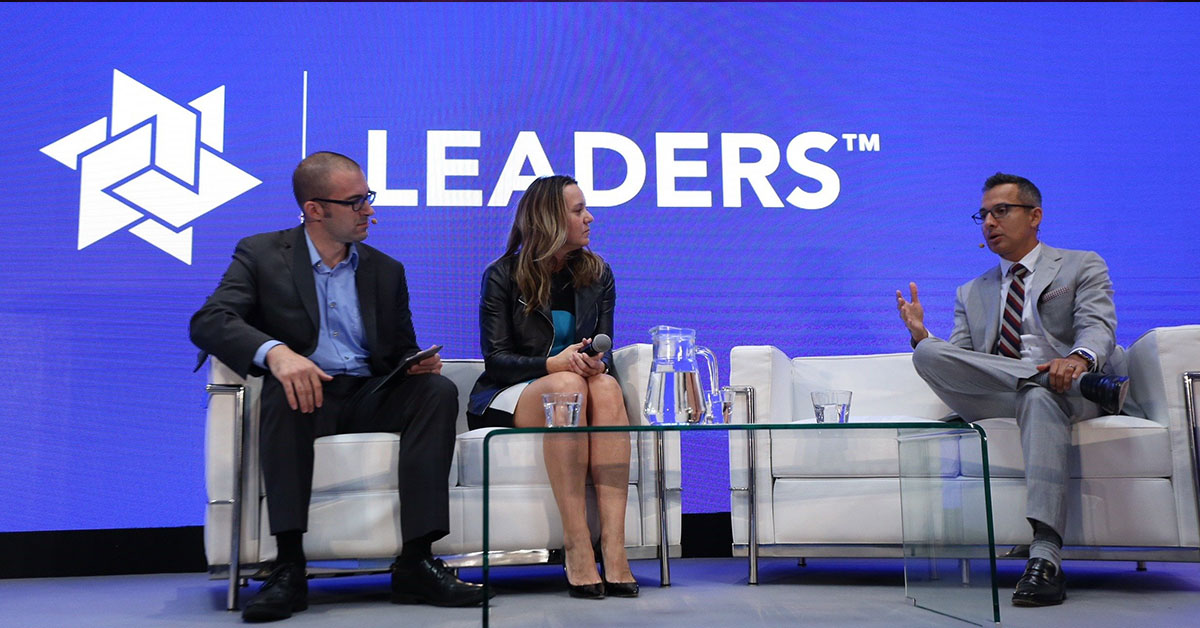
Leaders Week London 2019: Data Continues to Dominate
Last month, KORE attended and presented at Leaders Week London 2019, hosted at Twickenham Stadium and once again, Leaders delivered on another great event. The
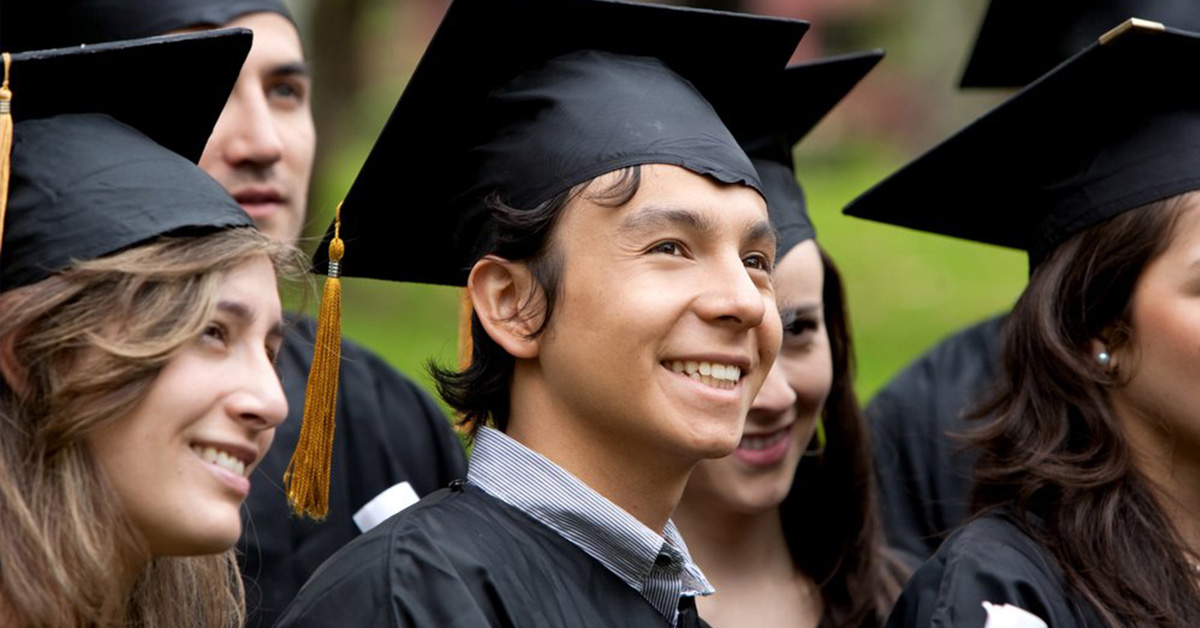
If I Only Knew Then What I Know Now: Career Advice in Sports Business Intelligence
If you’ve ever listened to KORE Software’s Inside Sports Business Intelligence podcast, you’ve probably heard Russell Scibetti ask “If you could go back and give
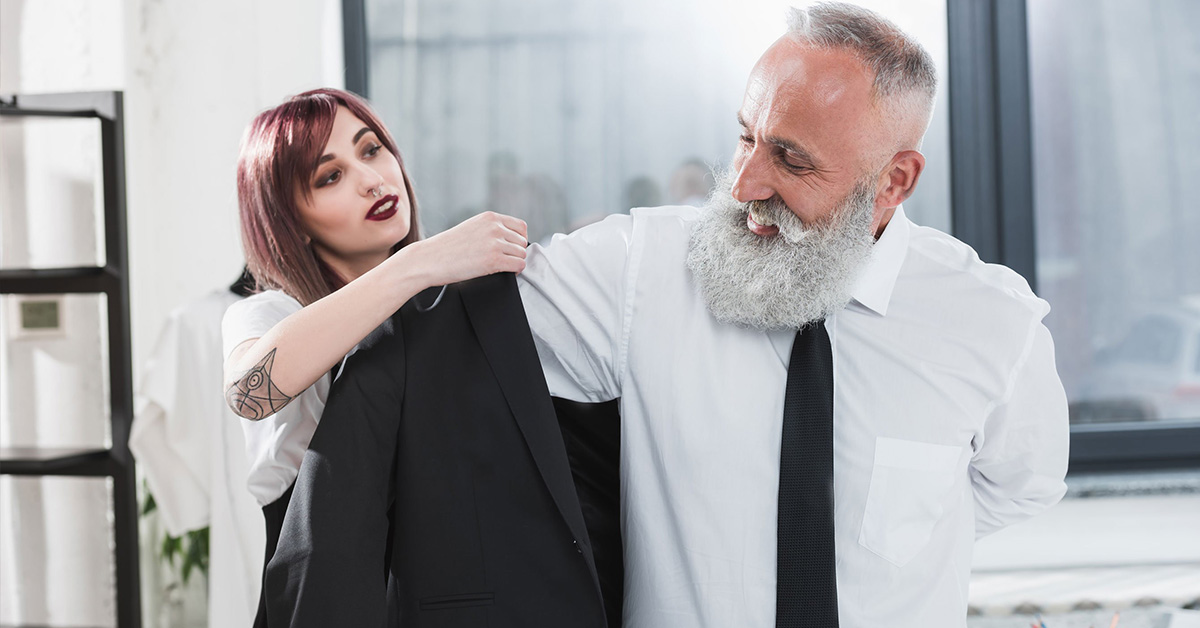
Does This Sponsorship Suit My Marketing Strategy?
Evaluating and determining whether a rights holder can provide a good opportunity, in line with an approved marketing spend, is arguably one of the biggest
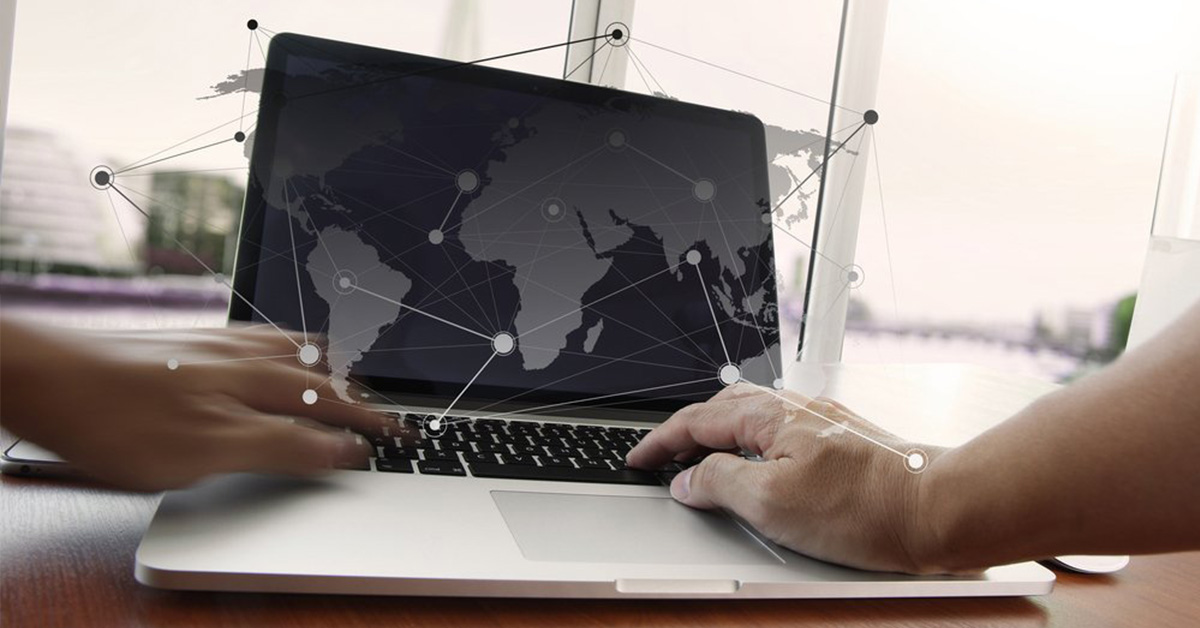
Digital Marketing Insights from The Business of Sports in China
Last month, KORE’s Chairman Matt Sebal was invited to participate in a conference in Shanghai on “The Business of Sports in China: Score and Change”
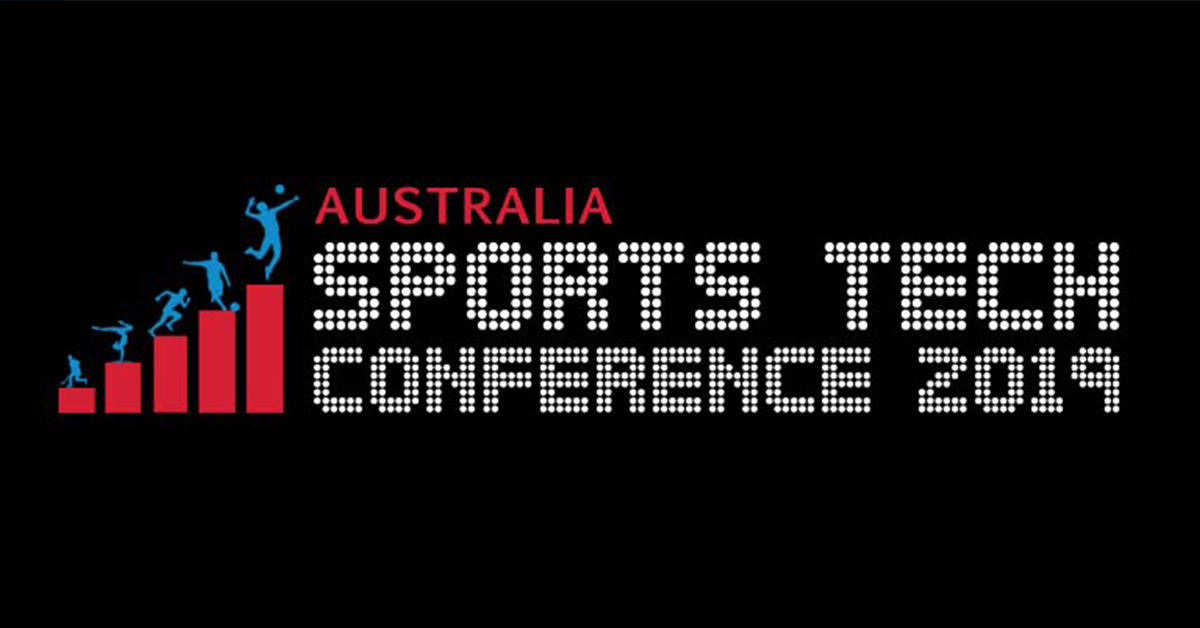
Australia Sports Tech Conference 2019: Fan Engagement is Alive and Flourishing
This August I was lucky enough to attend and co-present at the Australia Sports Tech Conference in Melbourne. It was an outstanding event, bringing together a variety
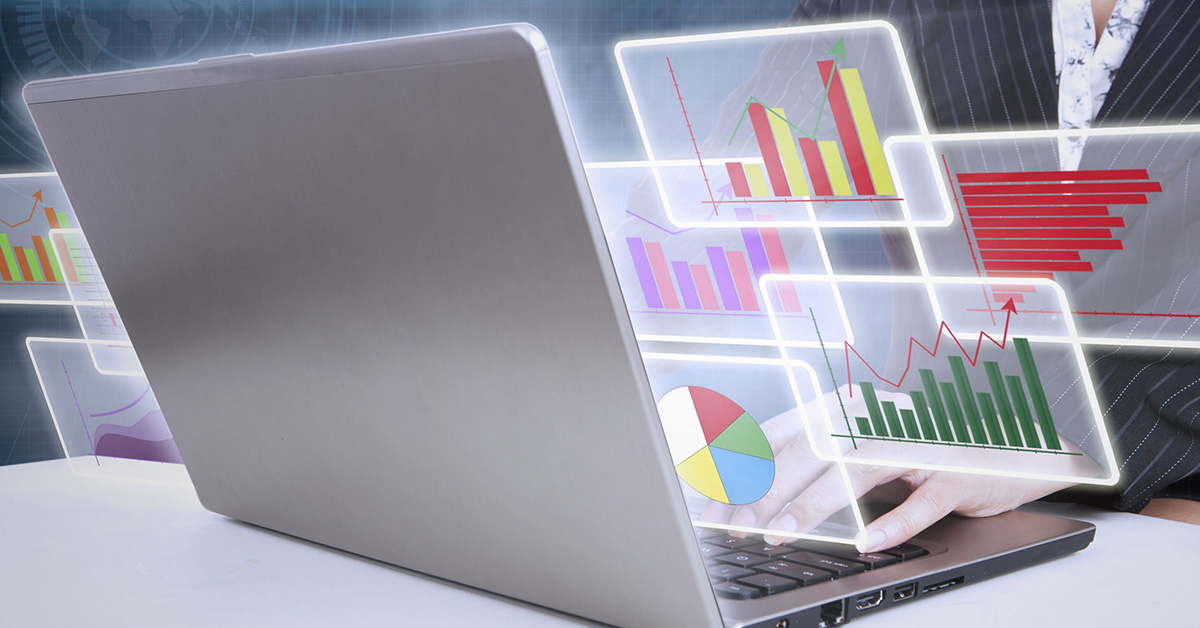
Sponsorship Measurement and Reporting – The Fan Data Way
Sponsorship measurement is not something new. It has been part of the industry for years and led by pioneers such as Repucom, Kantar, and a
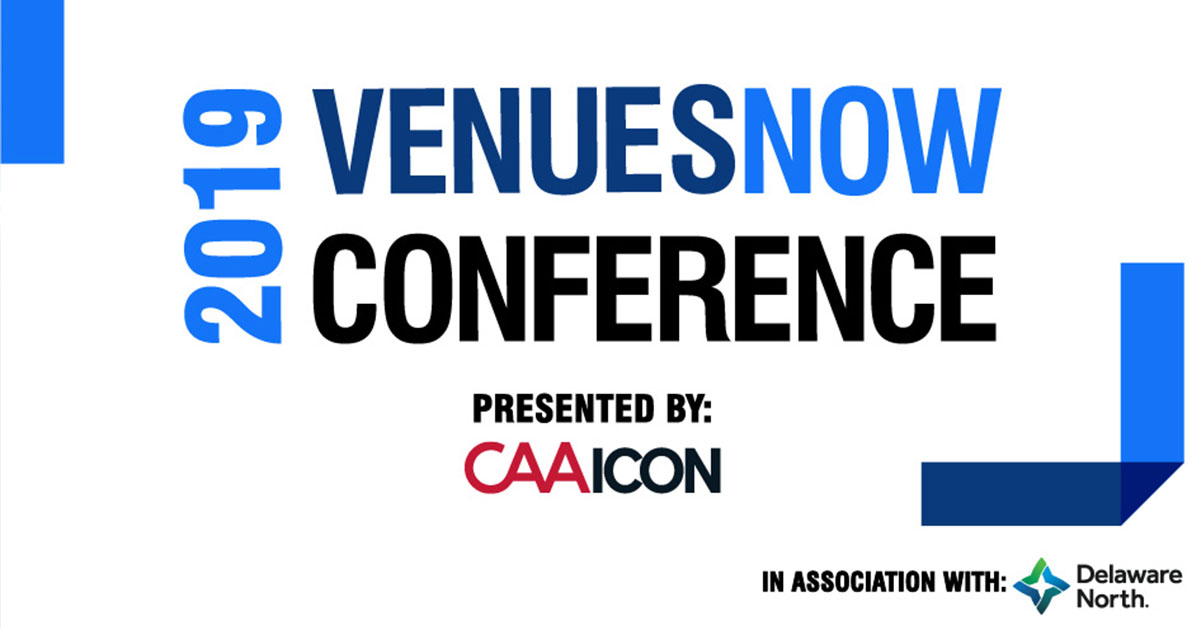
Top Takeaways From VenuesNow 2019
Most conferences that KORE attends have a clear focus around analytics and technology as it related to ticket sales, sponsorship, and marketing, but last week
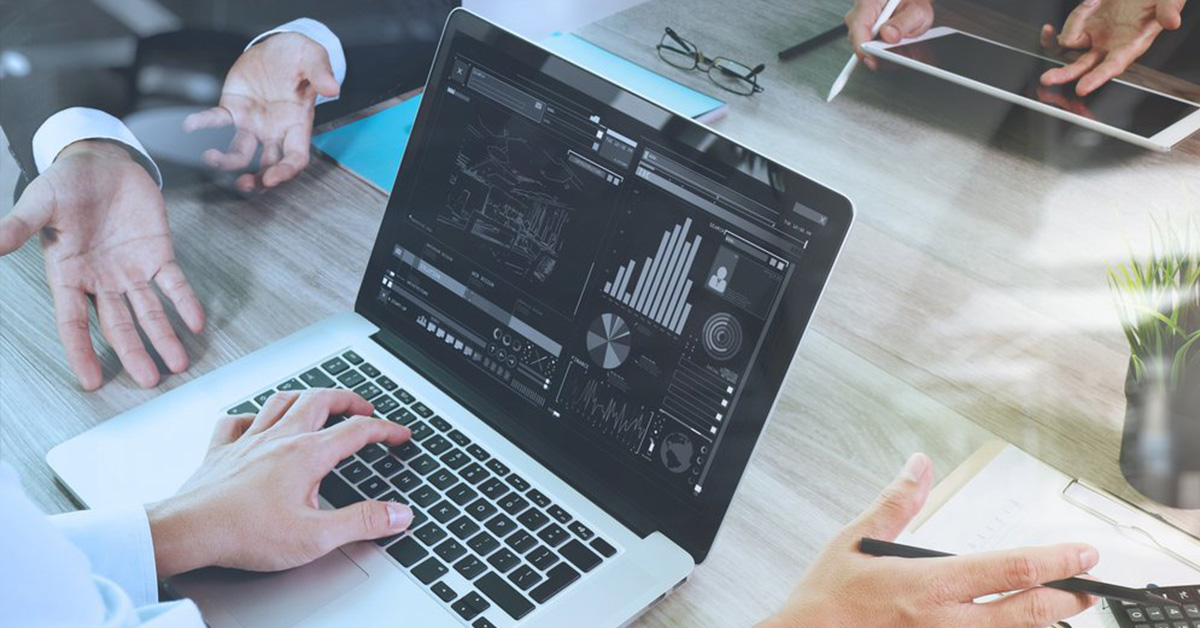
Business Intelligence Skill Development: Where Do I Begin?
We are all busy people. Your calendar is filled with meetings, conference calls, and a to-do list that seems to never end. In the constantly
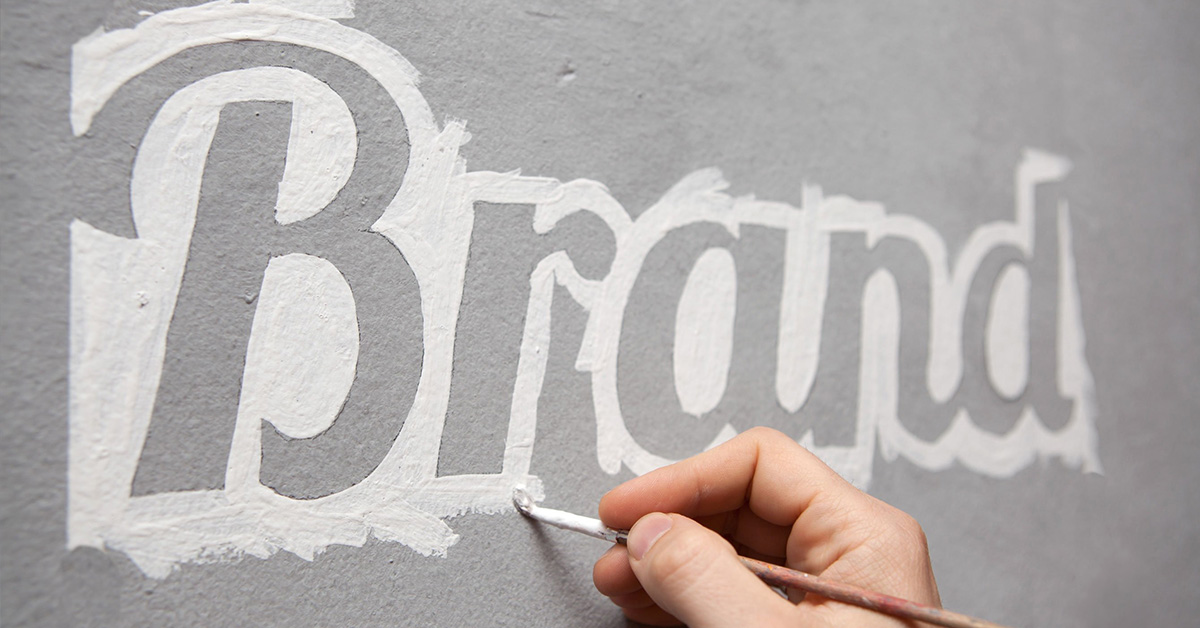
Is Sponsorship worth it if we don’t need the branding play?
Sponsorship, as a part of broader marketing strategy, is becoming increasingly effective as a method of reaching specific and targeted audiences than ever before. As
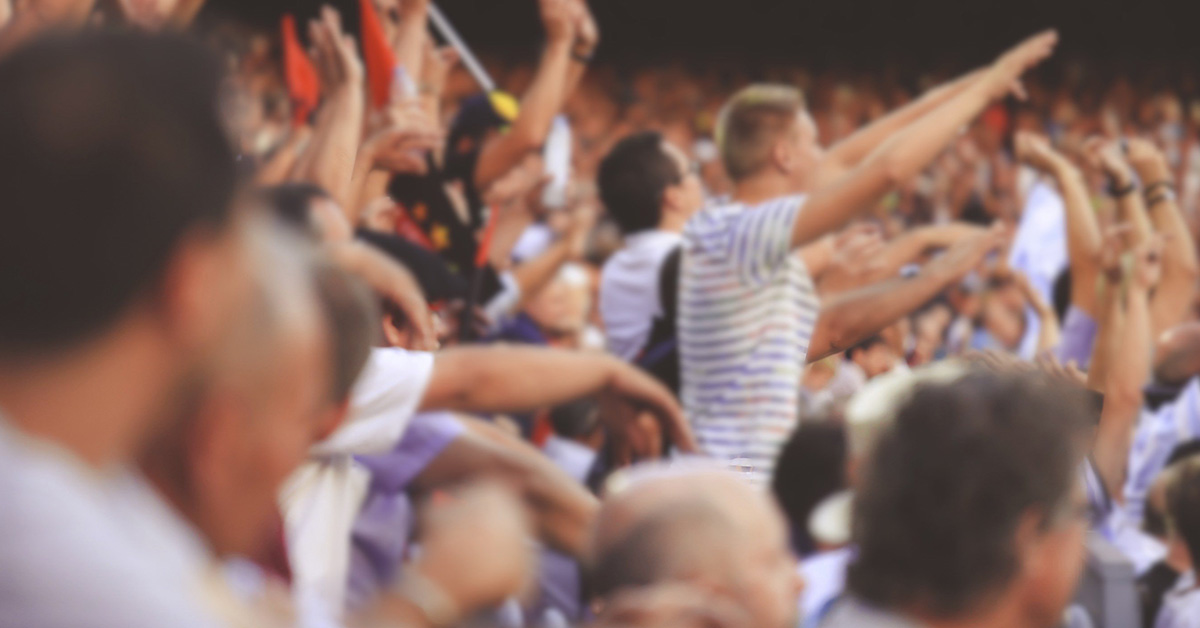
The Sales Slice and Dice: 3 Ways to Improve Your Lead List Through Segmentation
For sports business professionals, nothing quite compares to the energy behind the scenes leading up to a new banner year. With the start of the
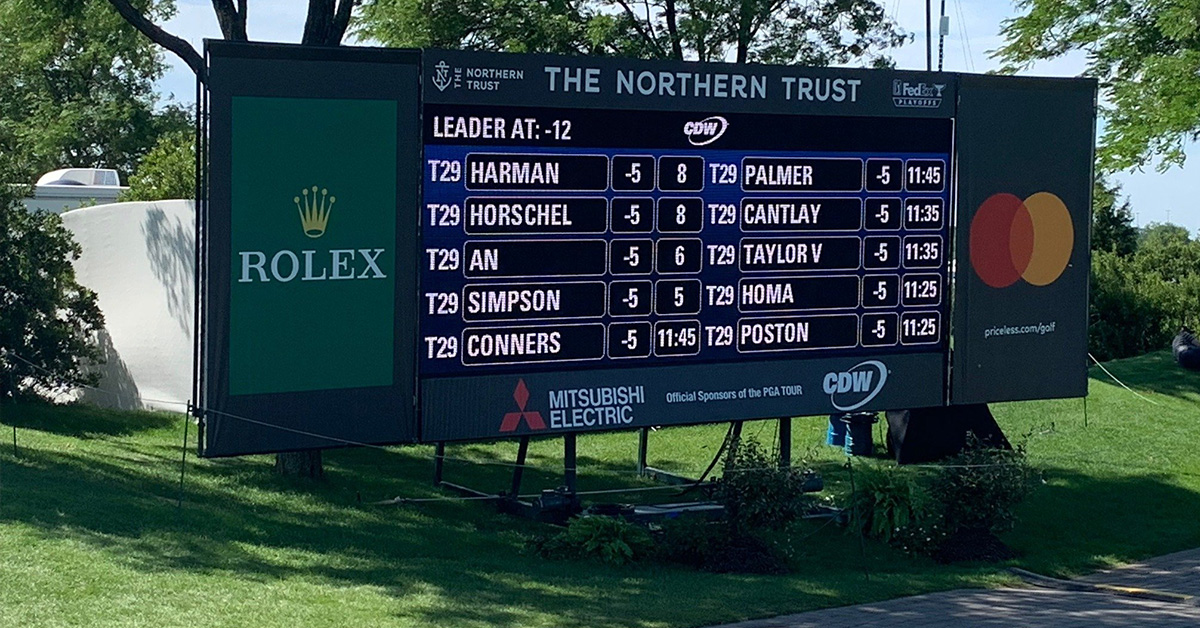
Six Sponsorship Activation Lessons from The Northern Trust
This past weekend I had the chance to attend The Northern Trust PGA TOUR event in Jersey City, and as I am prone to do, I
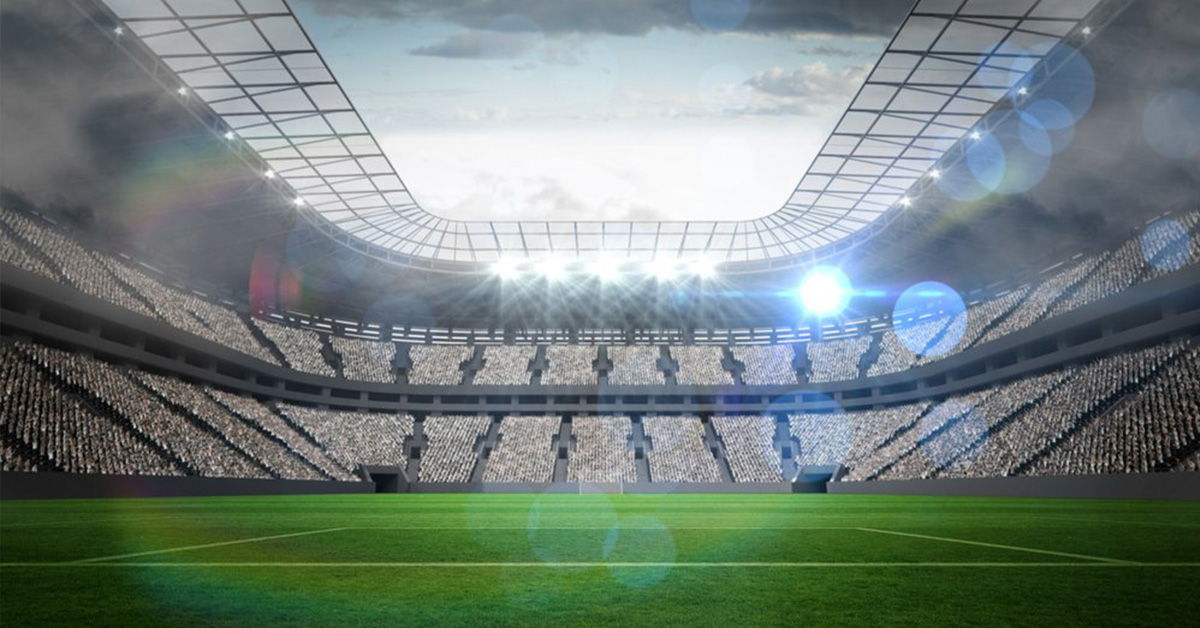
A Business Metrics Preview for the 2019-20 Premier League Season
With the 2019-20 Premier League season right around the corner, we thought we’d explore some key metrics across the member clubs around social, attendance, and
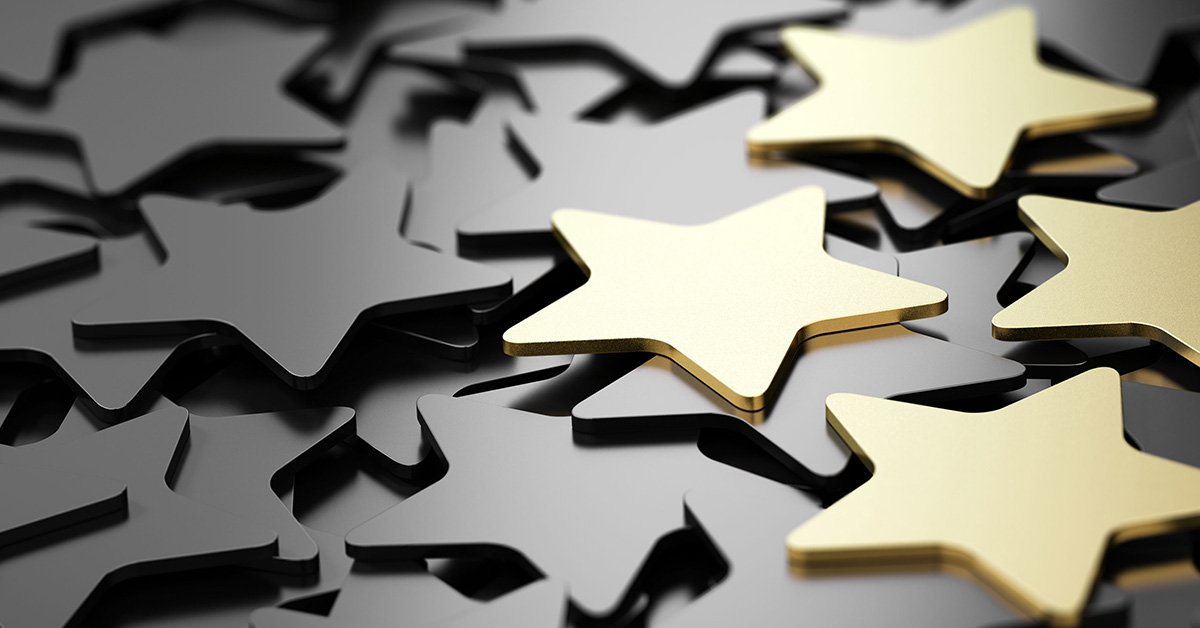
Is Sponsorship Keeping Up With Award Winning Trends?
Like most of my generation, the first thing I usually do in the morning is roll over, reach for my phone, and mindlessly scroll through
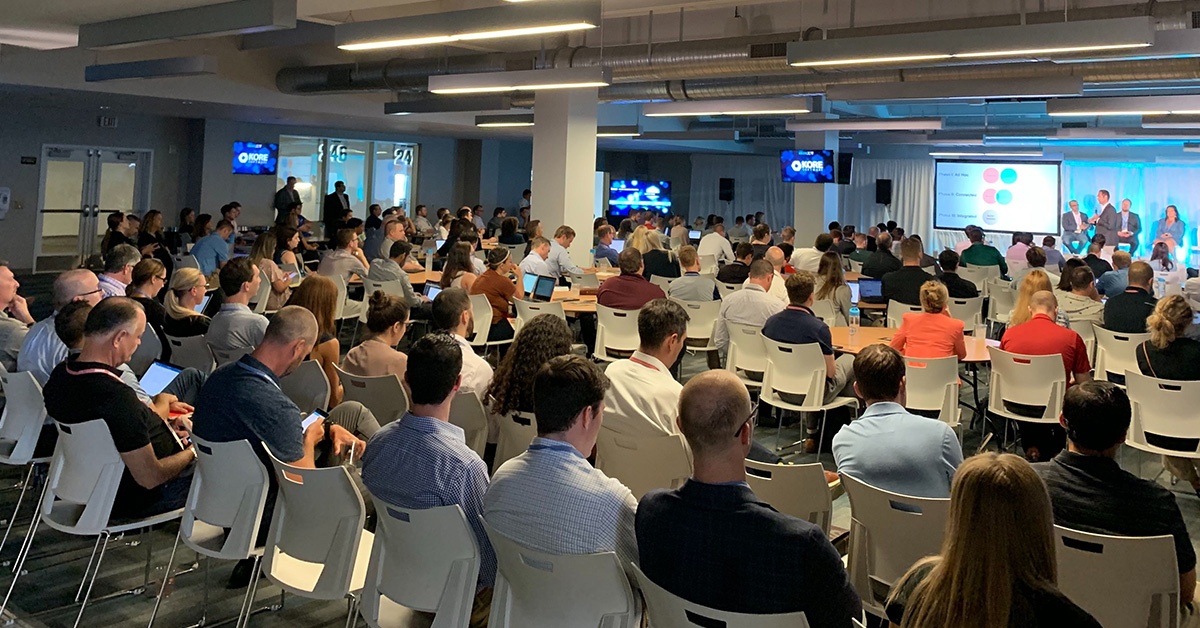
Trends and Insights from SEAT 2019
Last week I had the chance to attend my 9th SEAT Conference (or 11th, if you count the 2 I attended in London). KORE has
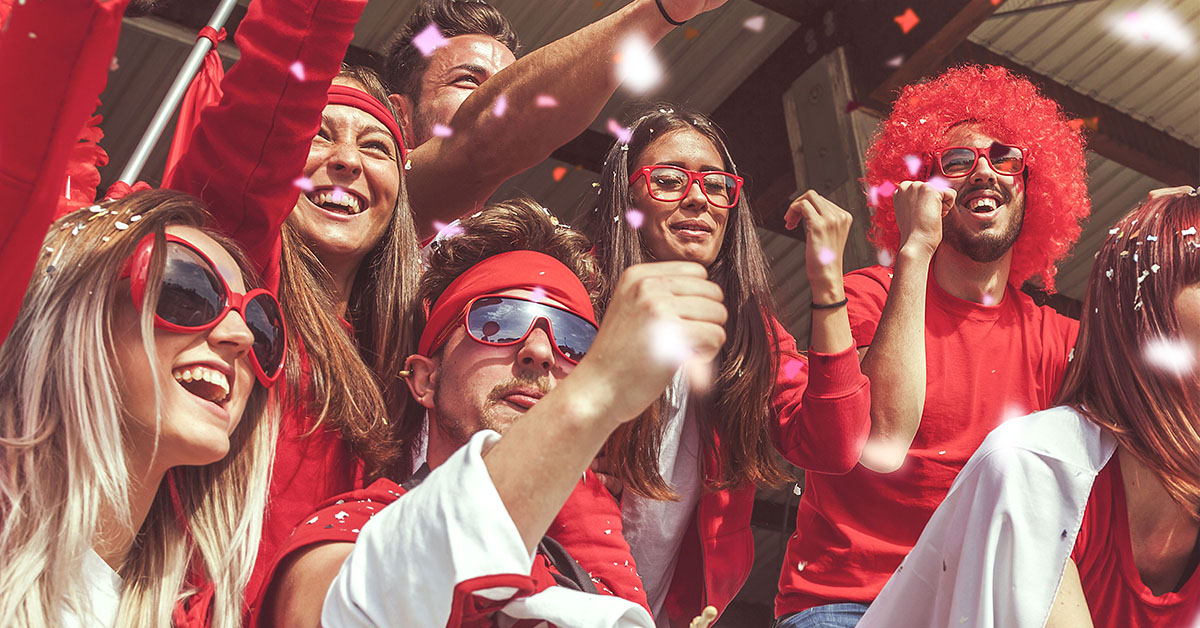
The Evolution of Sponsor Activations And How Businesses Are Changing To Manage Success
Sponsorship has been through a significant shift over the past decade or so. We have seen a drastic change from logo’s, tickets, corporate entertainment, and
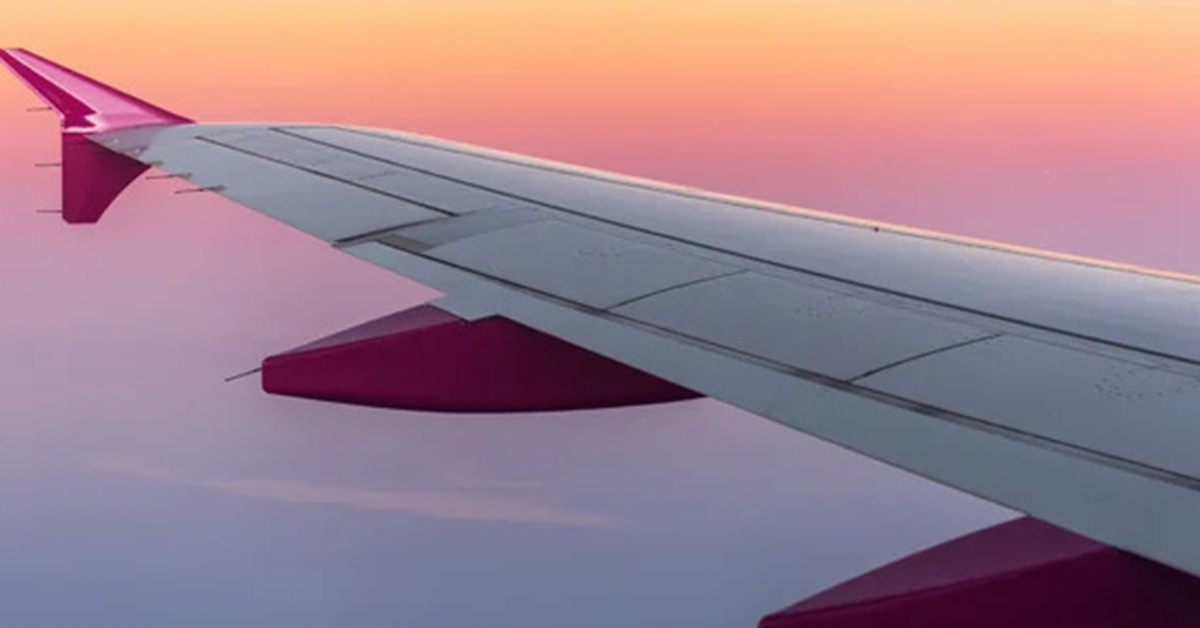
Airline Sponsorships – Surely, They do More Than Just Football?
With my eyes glued to the end of the European football season (English Premier League and the Champions League/Europa League), it was impossible not to
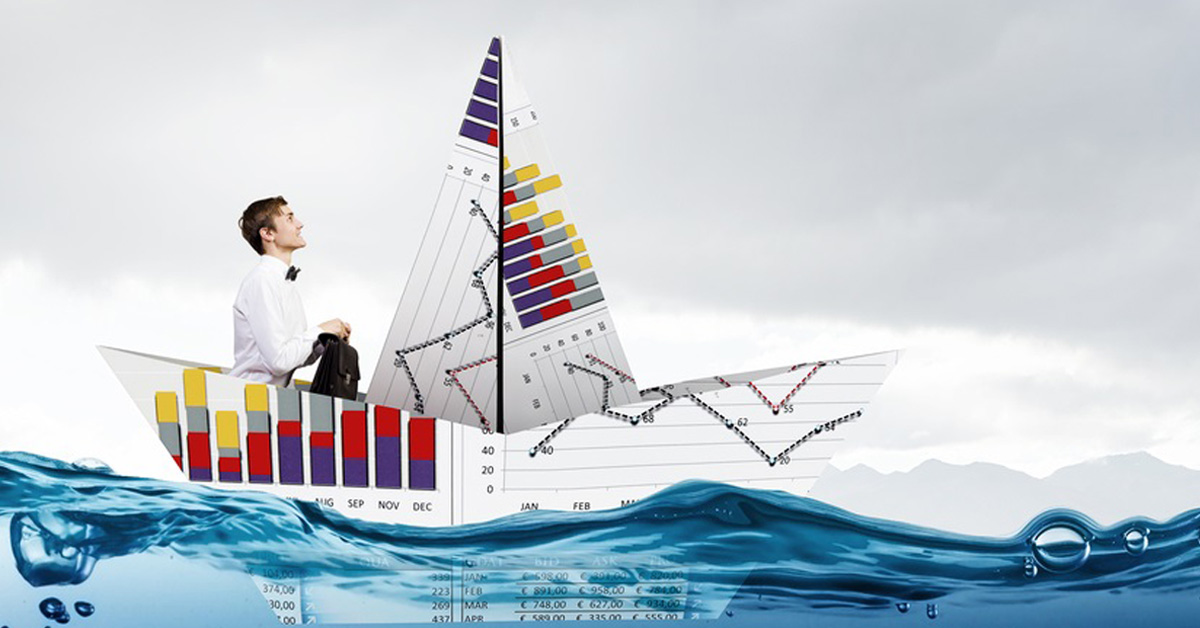
Has Your Sponsor-“ship” Team Sprung A Leak?
Would you cross the Atlantic Ocean in a ship filled with holes? Of course not, that seems like an obvious answer. Well, if you’re still
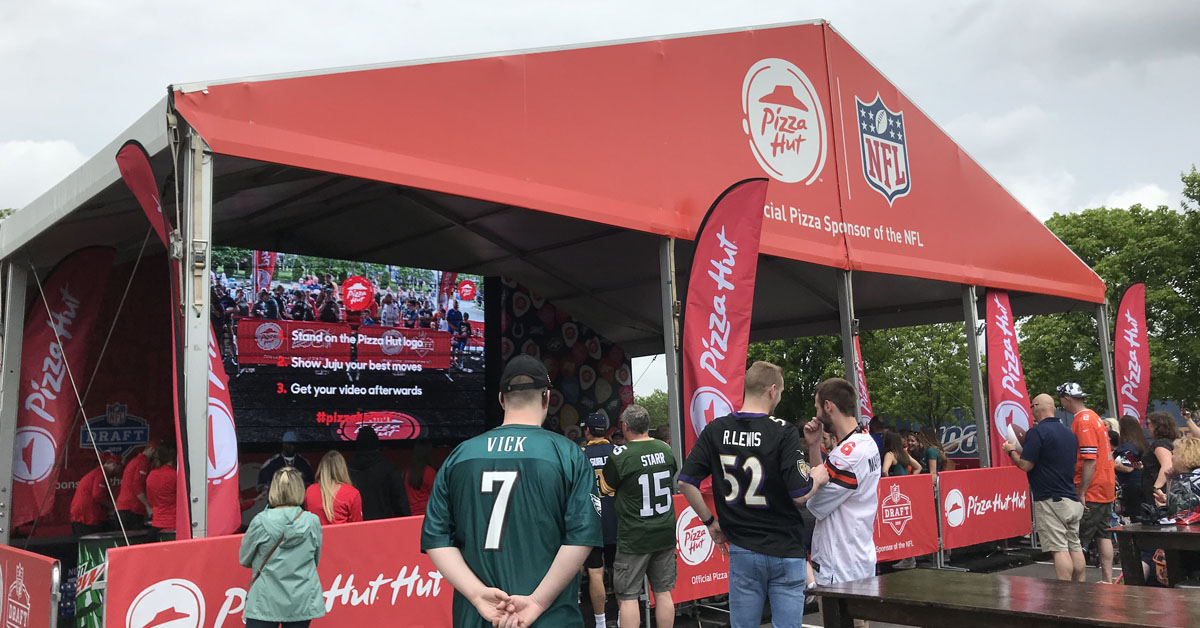
4 Things Brands Want You to Know About Sponsorship Activations
Have you ever been planning out an activation and thought “Would have loved to have known that earlier”? Chances are you just said “Yes” and had
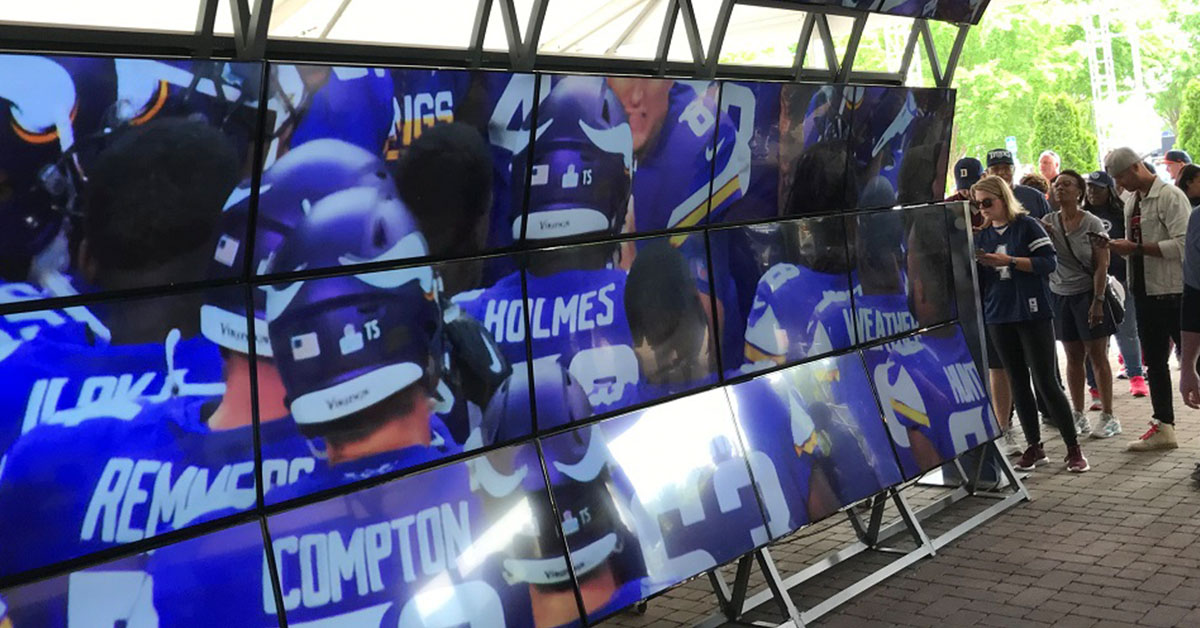
Strategic Activation at the NFL Draft Experience
Have you ever been planning out an activation and thought “Would have loved to have known that earlier”? Chances are you just said “Yes” and had
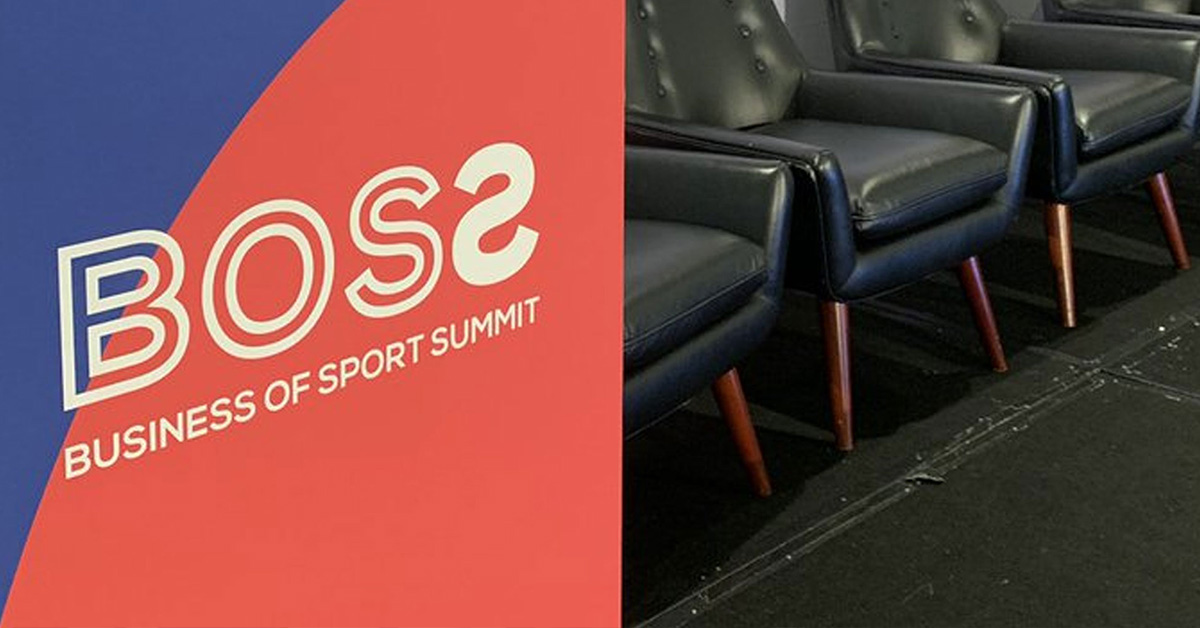
5 Takeaways From BOSS 2019
Recently, I was lucky enough to attend the Business of Sport Summit (BOSS) in Sydney Australia which included the privilege of sitting on a panel
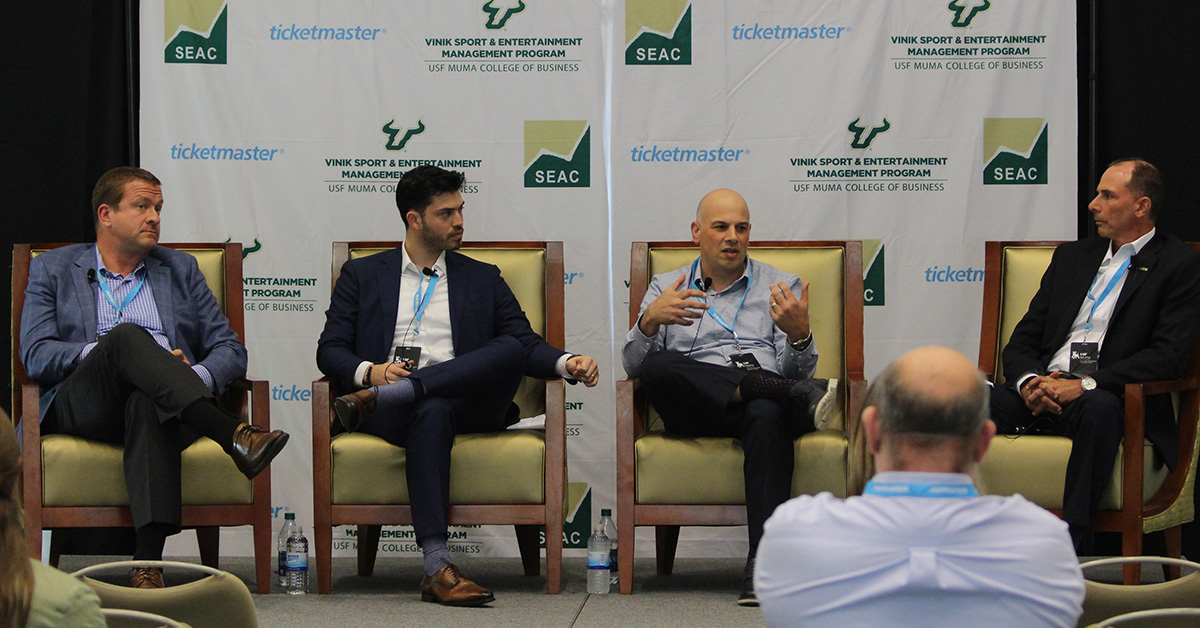
Key Takeaways from the USF Sport & Entertainment Analytics Conference
Every year the University of South Florida Vinik Sport & Entertainment Management MBA program hosts an annual Sport & Entertainment Analytics Conference (SEAC). Last week
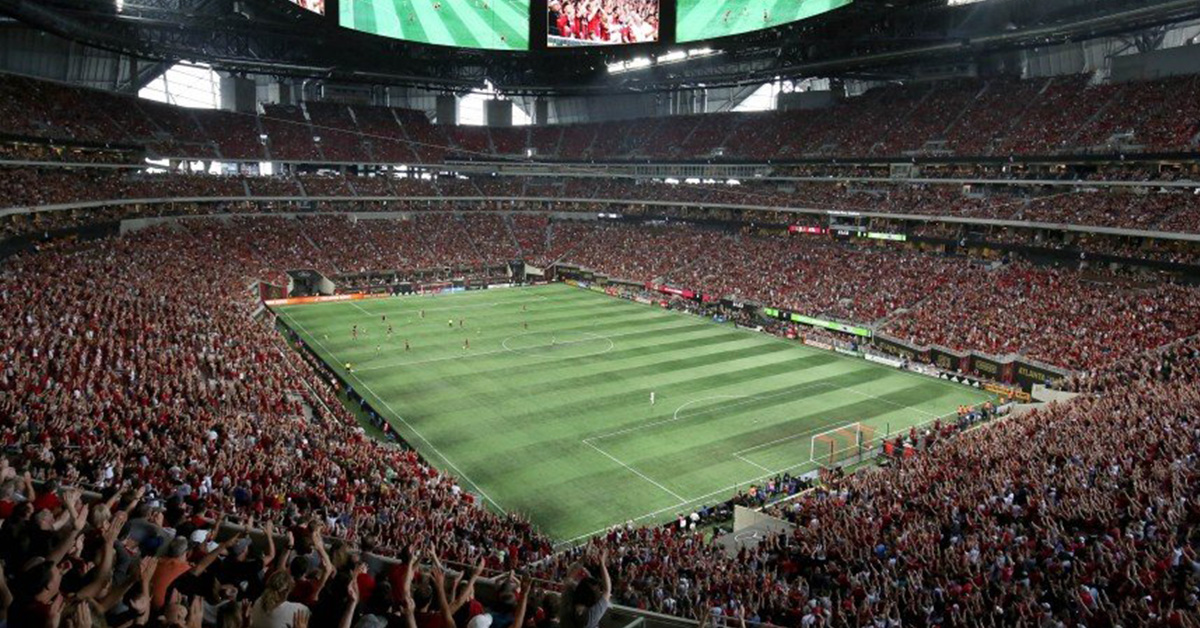
Looking at MLS Attendance Over the Years
We work with a lot of private data here at KORE, so sometimes it’s fun to mix it up and play with some publicly available
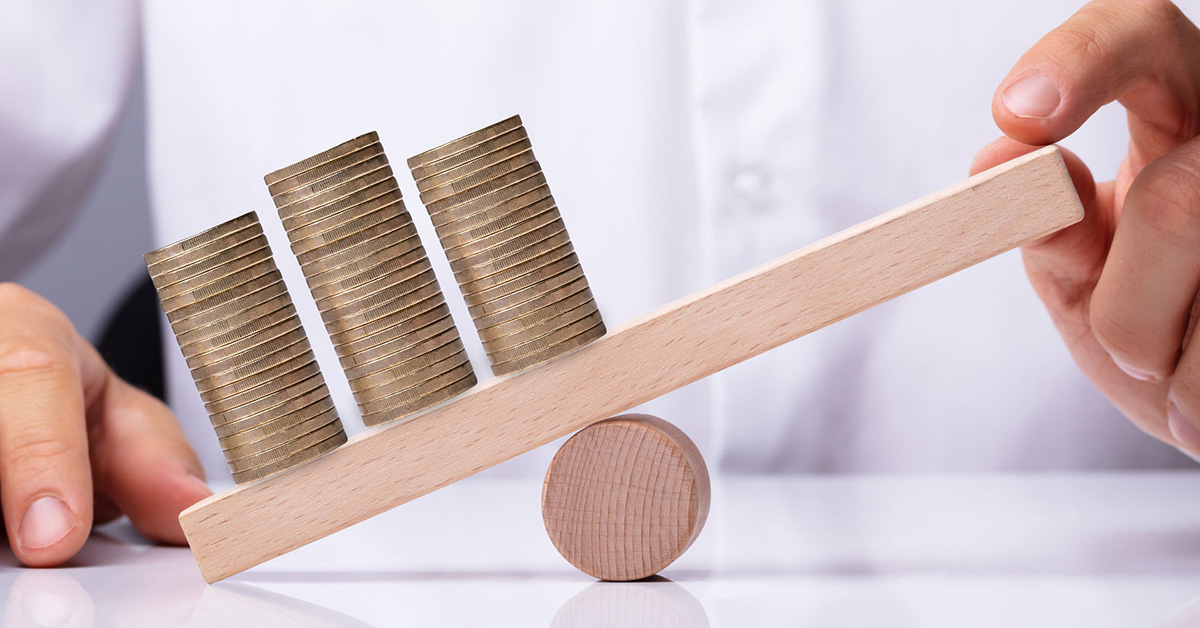
Where should the money go in the sponsorship deal or in the leverage?
“Do we need to spend over-and-above our sponsorship fee to maximise the deal’s return?” What a question! When signing off on a sponsorship deal, it
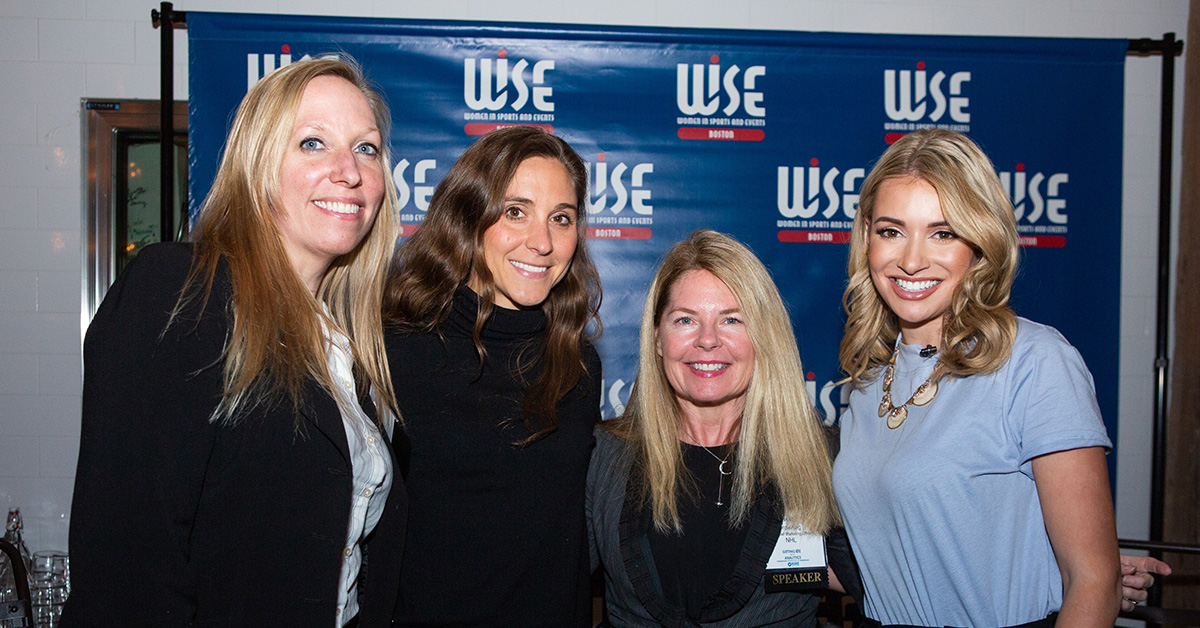
Getting WISE with Analytics and Digital Marketing
On February 28th, the Boston chapter of WISE (Women in Sports and Entertainment) and KORE Software hosted a panel on digital marketing and analytics. It
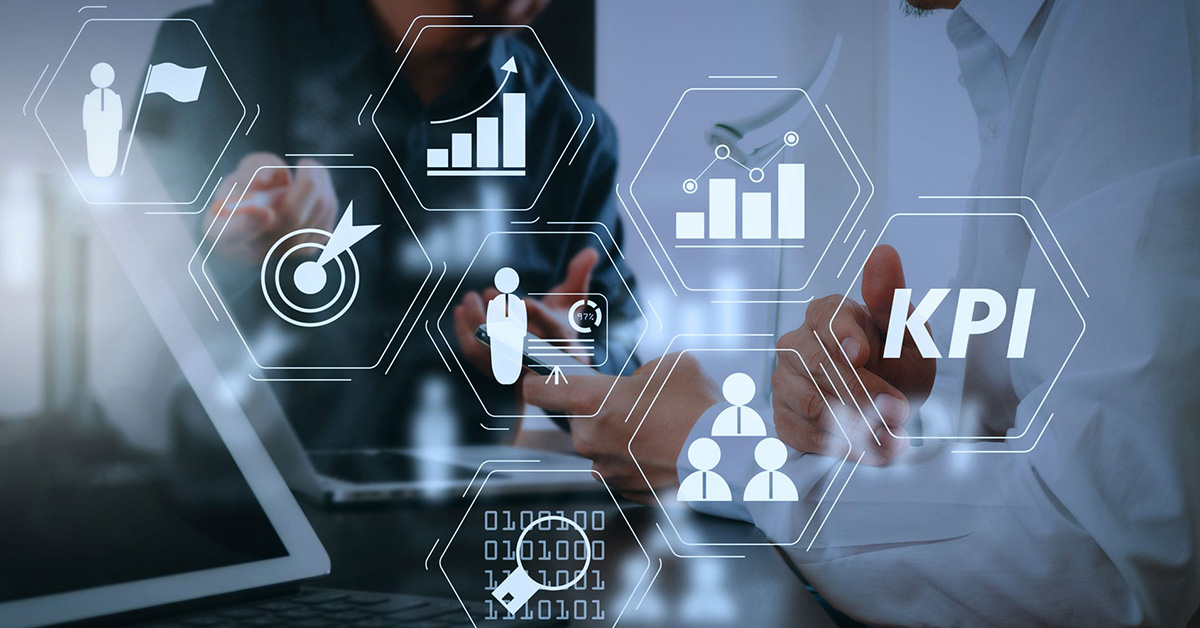
The Increasing Importance of B.I. in Sports and Entertainment
Sponsorship reporting is nothing new. It has been around since the dawn of sponsorship itself, however, as we know (and have spoken about in this
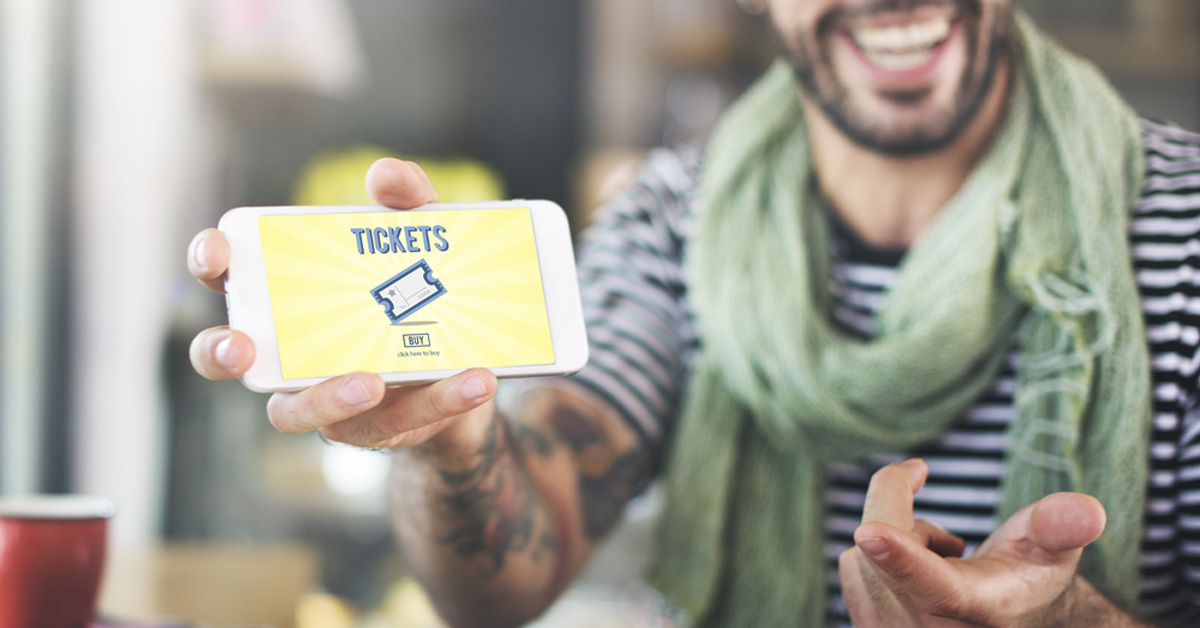
The Trend Towards Mobile-Only Ticketing
Today’s post originally ran in today’s JohnWallStreet newsletter which covers the intersection of sports and finance. It was based on an interview with Russell Scibetti, President of
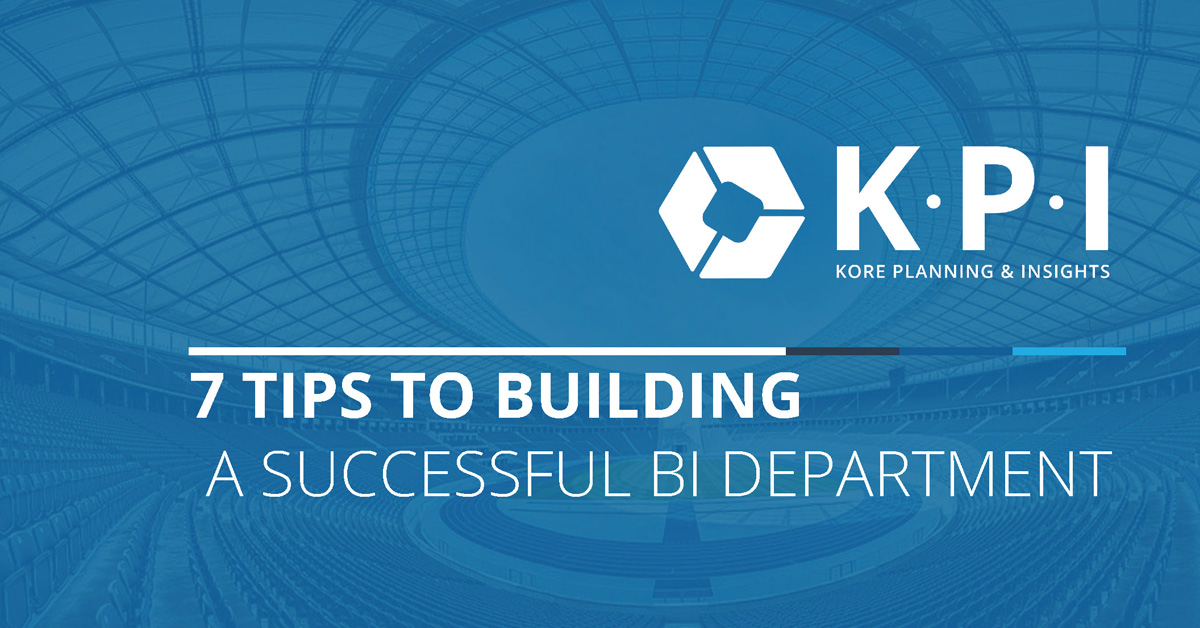
7 Tips for a Successful BI Department
There are few departments who deal with more rapid change and development than Business Intelligence. Technology doubles in capabilities approximately every two years. Team performance
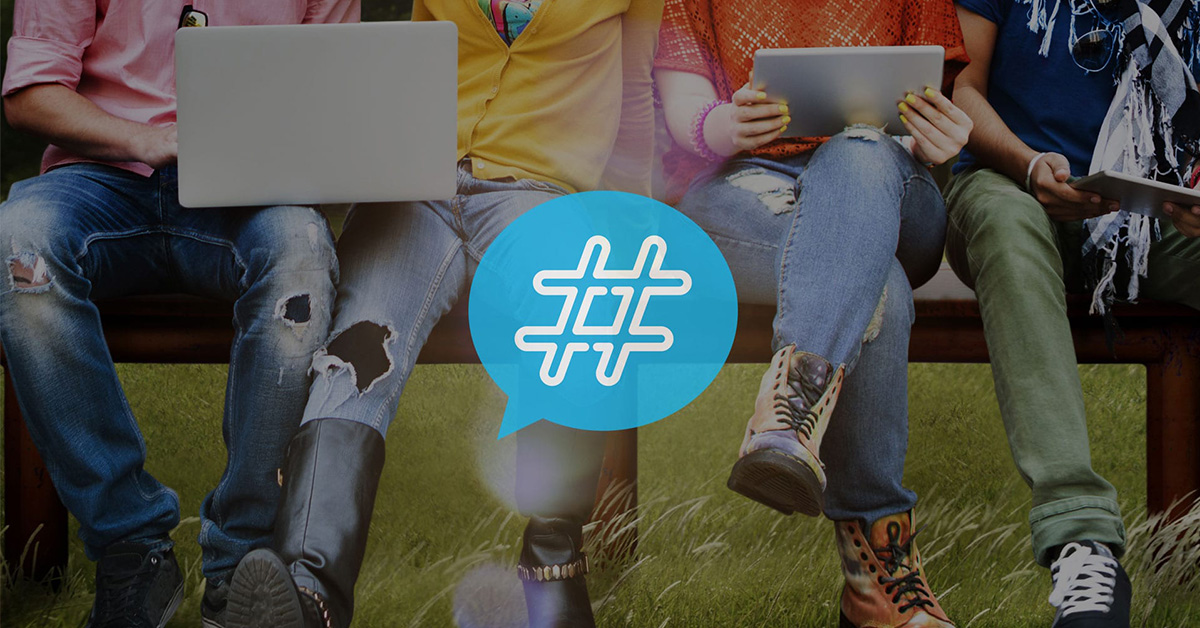
5 Trends in Sponsorship That We’re Not Talking About
Over the next few months, we’re going to begin seeing a range of Trends in Sponsorship reports bouncing around. For the most part, these are great
Partnership Optimization Activation Engagement Starts Here.
Partnership Optimization Activation Engagement Starts Here.
Let's talk about how your team can gain exceptional value from KORE's deep and connected ecosystem.